第一个MapReduce案例集群模式&本地模式

第一个MapReduce案例集群模式&Linux本地模式
记录一下自己在开发MapReduce程序的过程。
思考点是:如何下手,怎样开发。
1.对于平台的要求:环境已搭建完毕,且测试通过。
我自己平台是:Centos6.4 +jdk1.7+hadoop2.5.1 都是64位的
我这里写的是非常简单的一种:
创建一个java工程,导入jar包,写三个类。分别是:Mapper类、Reducer类、Runner类
Mapper类、Reducer类就是继承框架的虚类中的Mapper、Reducer ,实现其方法:map()、reduce()。
Runner类是描述job作业(使用那个Mapper、哪个Reducer),然后将作业提交给集群。
关于MapReduce原理这里就不说了。
先写Mapper类:
Mapper类代码:
import java.io.IOException;
import org.apache.commons.lang.StringUtils;
import org.apache.hadoop.io.LongWritable;
import org.apache.hadoop.io.Text;
import org.apache.hadoop.mapreduce.Mapper;
map方法是读取文件将文件中字符分割出来,进行最初划分
/**
我们自定义的Mapper类继承自Mapper(extends)实现其map()方法。
Mapper类含有四个参数分别代表着输入和输出,可以结合hadoop的内置封装的数据类型来理解。
map()的功能:
接收键值对,输出中间键值对,是将整个任务分解为多个小任务。其后由MapReduce框架将键值相同的值传给reduce方法。
map()方法中的参数说明:
共有三个参数:LongWritable key ---键值对中key
Text value ---键值对中的value
Context context ---记录输入的key/value,记录key/value的运算状态
这里扩展的内容是hadoop内置的数据类型:
BooleanWriteable :标准布尔型数值
ByteWritable :单字节数值
DoubleWritable: 双字节数值
FloatWritable :浮点数
IntWritable : 整型数
LongWritable: 长整型数
Text :使用UTF-8格式存储的文本
NullWritable:当<key,value>中的key或value为空时使用
*/
public class WordCountMapper extends Mapper<LongWritable, Text, Text, LongWritable>{
/**
map()方法实现思路:
1.获取文件的每行内容
2.将这行内容切分,调用StringUtils的方法是split方法,分割符为“”,切分后的字符放到字符串数组内
3.遍历输出<word,1>
*/
@Override
protected void map(LongWritable key, Text value,Context context)
throws IOException, InterruptedException {
//获取到一行文件的内容
String line = value.toString();
//切分这一行的内容为一个单词数组
String[] words = StringUtils.split(line, " ");
//遍历 输出 <word,1>
for(String word:words){
context.write(new Text(word), new LongWritable(1));
}
}
}
Reducer类代码:
import java.io.IOException;
import org.apache.hadoop.io.LongWritable;
import org.apache.hadoop.io.Text;
import org.apache.hadoop.mapreduce.Reducer;
reduce方法得到从map方法提交的键值对,对具有相同键的进行合并,输出。
1.定义一个Reducer类继承自Reducer,Reducer包含有四个参数,<Text,LongWritable,Text,LongWritable>,
Reducer抽象类的四个形式参数类型指定了reduce函数的输入和输出类型。
在本例子中,输入键是单词,输入值是单词出现的次数,经过reduce()方法处理将单词出现的次数进行叠加,
输出单词和单词总数。
2.实现虚类Reducer的reduce()方法
reduce()方法的说明:
参数三个:
Text key -----单词
Iterable<LongWritable> values -----单词出现的次数
Context context -----任务的上下文,包含整个任务的全部信息
reduce()方法的功能是:
汇总并输出单词出现的总次数。
由上面我们可以得知reduce()方法接收的参数:key值为单词,value值是迭代器,该迭代内存储的是单词出现的次数,
context负责将生成的k/v输出。通过遍历values,调用values的get()方法获取Long值即为出现的次数,
累加后context对象调用其write()方法将结果输出。
public class WordCountReducer extends Reducer<Text, LongWritable, Text, LongWritable>{
// key: hello , values : {1,1,1,1,1.....}
@Override
protected void reduce(Text key, Iterable<LongWritable> values,Context context)
throws IOException, InterruptedException {
//定义一个累加计数器 定义为Long类型
long count = 0;
for(LongWritable value:values){
//调用value的get()方法将long值取出来
count += value.get();
}
//输出<单词:count>键值对
context.write(key, new LongWritable(count));
}
}
Runner类代码:
import java.io.IOException;
import org.apache.hadoop.conf.Configuration;
import org.apache.hadoop.fs.Path;
import org.apache.hadoop.io.LongWritable;
import org.apache.hadoop.io.Text;
import org.apache.hadoop.mapreduce.Job;
import org.apache.hadoop.mapreduce.lib.input.FileInputFormat;
import org.apache.hadoop.mapreduce.lib.output.FileOutputFormat;
/**
* 用来描述一个作业job(使用哪个mapper类,哪个reducer类,输入文件在哪,输出结果放哪。。。。)
* 然后提交这个job给hadoop集群
* @author duanhaitao@itcast.cn
*两个jar包,两个类型,两个类,两个路径
*/
//cn.itheima.bigdata.hadoop.mr.wordcount.WordCountRunner
public class WordCountRunner {
public static void main(String[] args) throws Exception {
Configuration conf = new Configuration();
//创建job对象需要conf对象,conf对象包含的信息是:所用的jar包,
Job wcjob = Job.getInstance(conf);
//设置job所使用的jar包,使用Configuration对象调用set()方法,设置mapreduce.job.jar wcount.jar
conf.set("mapreduce.job.jar", "wcount.jar");
//设置wcjob中的资源所在的jar包
//调用job对象的setJarByClass()方法,参数是WordCountRunner.class,设置job作业中的资源所在的jar包
wcjob.setJarByClass(WordCountRunner.class);
//wcjob要使用哪个mapper类,job对象调用setMapperClass()方法,参数是WordCountMapper.class
wcjob.setMapperClass(WordCountMapper.class);
//wcjob要使用哪个reducer类,job对象调用setReducerClass()方法,参数为WordCountReducer.class
wcjob.setReducerClass(WordCountReducer.class);
//wcjob的mapper类输出的kv数据类型
//job对象调用setMapperOutputKeyClass();设置Mapper类输出的key值的类型--Text
//job对象调用setMapperOutputValueClass();设置Mapper类输出value值的类型--LongWritable
wcjob.setMapOutputKeyClass(Text.class);
wcjob.setMapOutputValueClass(LongWritable.class);
//wcjob的reducer类输出的kv数据类型
//job对象调用setOutputKey
wcjob.setOutputKeyClass(Text.class);
wcjob.setOutputValueClass(LongWritable.class);
//指定要处理的原始数据所存放的路径
//调用FileInputFormat对象的setInputPath()方法,参数的文件路径,是设置的源数据路径,当此处为集群的路径是就是跑在集群上的程序,
//如果设置在当前机器的路径,就是本地模式
FileInputFormat.setInputPaths(wcjob, "hdfs://hadoop01:9000/wc/srcdata");
//指定处理之后的结果输出到哪个路径,注意此时应当在路径应当是差不多的
FileOutputFormat.setOutputPath(wcjob, new Path("hdfs://hadoop:9000/wc/output"));
//调用job对象的waitForCompletion()方法,提交作业。
boolean res = wcjob.waitForCompletion(true);
System.exit(res?0:1);
}
}
上面的代码是运行在集群上的,如果运行在本地是将文件输入路径和输出路径写为本地的路径即可。对于其他的不需要改变。
在创建完成这三个类后,打开Linux终端:
1.选中创建完成的工程,点击右键,选择Export,以jar包的格式导出该文件。
2.输入命令:jps 查看此时进程确定有没有启动hadoop、yarn,若未启动hadoop则输入命令:start-dfs.sh、start-yarn.sh启动即可
3.输入命令:hadoop jar wcount.jar Runner类的全类名
这样就可以跑起来了。在跑完程序之后
4.输入命令:hadoop fs -ls /wc/output ----程序运行结束后输出文件的位置,确定文件正常输出
5.输入命令:hadoop fs -cat /wc/output/xxxx ----查看该文件
本地运行模式就是直接执行Runner的main方法产生一个RunJar的客户端,会持有本地提交器,持有一个localsumbitter,向本地MR模拟器提交任务。在Linux本地实现的。
遇到的异常:
java.lang.Exception: java.io.IOException: Mkdirs failed to create file:/home/my/_temporary/0/_temporary/attempt_local2063472742_0001_r_000000_0
at org.apache.hadoop.mapred.LocalJobRunner$Job.runTasks(LocalJobRunner.java:462)
at org.apache.hadoop.mapred.LocalJobRunner$Job.run(LocalJobRunner.java:529)
Caused by: java.io.IOException: Mkdirs failed to create file:/home/my/_temporary/0/_temporary/attempt_local2063472742_0001_r_000000_0
at org.apache.hadoop.fs.ChecksumFileSystem.create(ChecksumFileSystem.java:438)
at org.apache.hadoop.fs.ChecksumFileSystem.create(ChecksumFileSystem.java:424)
at org.apache.hadoop.fs.FileSystem.create(FileSystem.java:906)
at org.apache.hadoop.fs.FileSystem.create(FileSystem.java:887)
at org.apache.hadoop.fs.FileSystem.create(FileSystem.java:784)
at org.apache.hadoop.mapreduce.lib.output.TextOutputFormat.getRecordWriter(TextOutputFormat.java:132)
at org.apache.hadoop.mapred.ReduceTask$NewTrackingRecordWriter.<init>(ReduceTask.java:540)
at org.apache.hadoop.mapred.ReduceTask.runNewReducer(ReduceTask.java:614)
at org.apache.hadoop.mapred.ReduceTask.run(ReduceTask.java:389)
at org.apache.hadoop.mapred.LocalJobRunner$Job$ReduceTaskRunnable.run(LocalJobRunner.java:319)
at java.util.concurrent.Executors$RunnableAdapter.call(Executors.java:441)
at java.util.concurrent.FutureTask$Sync.innerRun(FutureTask.java:303)
at java.util.concurrent.FutureTask.run(FutureTask.java:138)
at java.util.concurrent.ThreadPoolExecutor$Worker.runTask(ThreadPoolExecutor.java:886)
at java.util.concurrent.ThreadPoolExecutor$Worker.run(ThreadPoolExecutor.java:908)
at java.lang.Thread.run(Thread.java:662)
解决办法:
输出的结果文件应当放到一起同一目录,即如我的代码中,这个异常就是我的输出的目录与我的源文件的目录不一致造成的。
对于如何将本地模式的基础上修改为集群模式的方法是添加四个xml文件:
core-site.xml hdfs-site.xml mapred-site.xml yarn-site.xml
总结 MR Job的几种运行模式 :
1.在服务器上运行yarn集群模式:
在Eclipse中开发好mr程序(在Windows或Linux中都可),然后打包成jar包,上传到服务器。
执行命令 hadoop jar jar包名 Runner类的全类名
2.在Linux中Eclipse开发平台中直接启动Runner类运行main方法,这种方式既可以为yarn集群模式,也可以为本地模式:
关键点是:
取决于一个配置参数:mapreduce.framework.name=local(yarn)
当为local时则运行为本地模式;当为yarn时则为运行yarn集群模式。
----yarn模式:
a.将mr工程导出为jar包,放在工程目录下。
b.在main方法中,加入一个配置参数,conf.set("mapreduce.job.jar","mr导出的jar包名")
----本地模式:
在Eclipse中将mr工程导出为jar包,拷贝到当前目录下,直接运行main方法。
3.在Windows的Eclipse中运行本地模式的步骤:
a.在Windows中存放hadoop的安装包,并配置其环境变量。
b.根据Windows的版本,对应的替换掉hadoop安装包中的本地库(bin、lib)
c.mr工程中不需要设置mapreduce.framework.name。
4.在Windows的Eclipse中运行yarn集群模式的步骤:
此时只添加该单一的参数,设置conf.set("mapreduce.job.jar","mr导出的jar包名")是不行的,因为在Windows中还需要其他的系统变量如:JAVA_HOME 等。因为在将job提交到服务器Linux中会有不兼容的问题出现,比如环境变量%与Linux中的$的区别。此时我们可以修改yarn-Runner方法的源码。此处修改较多。不建议使用。
************在后期时遇到的一些异常***********************
1.connection refused ---没写端口号
2.在第一次提交作业后一旦任务执行成功,必须将输出的文件删除,这是使用Hadoop shell命令:
hadoop fs -rm -r /output
3.对于将工程导出jar包,jar包的位置,并没有特殊的设置,就是jar包在那儿,那么运行hadoop jar 命令时必须在该目录下。
4.对于jar包实在安装包中找,按住ctr键查看的源代码是需要再下载的,并添加路径
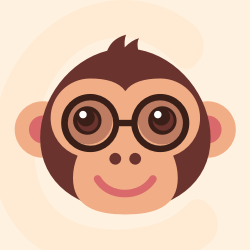



更多推荐
所有评论(0)