让keras训练深度网络时使用多个显卡
linux-dash
A beautiful web dashboard for Linux
项目地址:https://gitcode.com/gh_mirrors/li/linux-dash

·
1、使用nvidia-smi pmon 查看linux系统的gpu情况,如下:
显然是2张显卡,如何让它们都工作呢
2、keras提供了keras.utils import multi_gpu_model使用多个显卡的功能:
在原来的model基础上使用multi_gpu_model函数指定一下gpu个数即可:
model = multi_gpu_model(model, 2)
完整列子如下(如粗黑色字体描述):
root@deeplearning:/opt/soft/keras/examples# cat mnist_mlp_multi_gpu.py
'''Trains a simple deep NN on the MNIST dataset.
Gets to 98.40% test accuracy after 20 epochs
(there is *a lot* of margin for parameter tuning).
2 seconds per epoch on a K520 GPU.
'''
from __future__ import print_function
import keras
from keras.datasets import mnist
from keras.models import Sequential
from keras.layers import Dense, Dropout
from keras.optimizers import RMSprop
from keras.utils import multi_gpu_model
batch_size = 128
num_classes = 10
epochs = 20
# the data, shuffled and split between train and test sets
(x_train, y_train), (x_test, y_test) = mnist.load_data()
x_train = x_train.reshape(60000, 784)
x_test = x_test.reshape(10000, 784)
x_train = x_train.astype('float32')
x_test = x_test.astype('float32')
x_train /= 255
x_test /= 255
print(x_train.shape[0], 'train samples')
print(x_test.shape[0], 'test samples')
# convert class vectors to binary class matrices
y_train = keras.utils.to_categorical(y_train, num_classes)
y_test = keras.utils.to_categorical(y_test, num_classes)
model = Sequential()
model.add(Dense(512, activation='relu', input_shape=(784,)))
model.add(Dropout(0.2))
model.add(Dense(512, activation='relu'))
model.add(Dropout(0.2))
model.add(Dense(num_classes, activation='softmax'))
model = multi_gpu_model(model, 2)
model.summary()
model.compile(loss='categorical_crossentropy',
optimizer=RMSprop(),
metrics=['accuracy'])
history = model.fit(x_train, y_train,
batch_size=batch_size,
epochs=epochs,
verbose=1,
validation_data=(x_test, y_test))
score = model.evaluate(x_test, y_test, verbose=0)
print('Test loss:', score[0])
print('Test accuracy:', score[1])
最终运行的结果如下:
root@deeplearning:/opt/soft/keras/examples# python mnist_mlp_multi_gpu.py
Using TensorFlow backend.
60000 train samples
10000 test samples
2018-02-17 18:14:41.147652: I tensorflow/core/platform/cpu_feature_guard.cc:137] Your CPU supports instructions that this TensorFlow binary was not compiled to use: SSE4.1 SSE4.2 AVX AVX2 FMA
2018-02-17 18:14:41.304376: I tensorflow/stream_executor/cuda/cuda_gpu_executor.cc:892] successful NUMA node read from SysFS had negative value (-1), but there must be at least one NUMA node, so returning NUMA node zero
2018-02-17 18:14:41.304757: I tensorflow/core/common_runtime/gpu/gpu_device.cc:1030] Found device 0 with properties:
name: Tesla P4 major: 6 minor: 1 memoryClockRate(GHz): 1.1135
pciBusID: 0000:00:07.0
totalMemory: 7.43GiB freeMemory: 7.32GiB
2018-02-17 18:14:41.399113: I tensorflow/stream_executor/cuda/cuda_gpu_executor.cc:892] successful NUMA node read from SysFS had negative value (-1), but there must be at least one NUMA node, so returning NUMA node zero
2018-02-17 18:14:41.399487: I tensorflow/core/common_runtime/gpu/gpu_device.cc:1030] Found device 1 with properties:
name: Tesla P4 major: 6 minor: 1 memoryClockRate(GHz): 1.1135
pciBusID: 0000:00:08.0
totalMemory: 7.43GiB freeMemory: 7.32GiB
2018-02-17 18:14:41.399579: I tensorflow/core/common_runtime/gpu/gpu_device.cc:1045] Device peer to peer matrix
2018-02-17 18:14:41.399613: I tensorflow/core/common_runtime/gpu/gpu_device.cc:1051] DMA: 0 1
2018-02-17 18:14:41.399627: I tensorflow/core/common_runtime/gpu/gpu_device.cc:1061] 0: Y N
2018-02-17 18:14:41.399632: I tensorflow/core/common_runtime/gpu/gpu_device.cc:1061] 1: N Y
2018-02-17 18:14:41.399650: I tensorflow/core/common_runtime/gpu/gpu_device.cc:1120] Creating TensorFlow device (/device:GPU:0) -> (device: 0, name: Tesla P4, pci bus id: 0000:00:07.0, compute capability: 6.1)
2018-02-17 18:14:41.399663: I tensorflow/core/common_runtime/gpu/gpu_device.cc:1120] Creating TensorFlow device (/device:GPU:1) -> (device: 1, name: Tesla P4, pci bus id: 0000:00:08.0, compute capability: 6.1)
__________________________________________________________________________________________________
Layer (type) Output Shape Param # Connected to
==================================================================================================
dense_1_input (InputLayer) (None, 784) 0
__________________________________________________________________________________________________
lambda_1 (Lambda) (None, 784) 0 dense_1_input[0][0]
__________________________________________________________________________________________________
lambda_2 (Lambda) (None, 784) 0 dense_1_input[0][0]
__________________________________________________________________________________________________
sequential_1 (Sequential) (None, 10) 669706 lambda_1[0][0]
lambda_2[0][0]
__________________________________________________________________________________________________
dense_3 (Concatenate) (None, 10) 0 sequential_1[1][0]
sequential_1[2][0]
==================================================================================================
Total params: 669,706
Trainable params: 669,706
Non-trainable params: 0
__________________________________________________________________________________________________
Train on 60000 samples, validate on 10000 samples
Epoch 1/20
60000/60000 [==============================] - 4s 61us/step - loss: 0.2460 - acc: 0.9234 - val_loss: 0.1110 - val_acc: 0.9647
Epoch 2/20
60000/60000 [==============================] - 3s 49us/step - loss: 0.1018 - acc: 0.9693 - val_loss: 0.0804 - val_acc: 0.9766
Epoch 3/20
60000/60000 [==============================] - 3s 49us/step - loss: 0.0742 - acc: 0.9776 - val_loss: 0.0745 - val_acc: 0.9771
Epoch 4/20
60000/60000 [==============================] - 3s 49us/step - loss: 0.0599 - acc: 0.9820 - val_loss: 0.0698 - val_acc: 0.9793
Epoch 5/20
60000/60000 [==============================] - 3s 49us/step - loss: 0.0514 - acc: 0.9844 - val_loss: 0.0761 - val_acc: 0.9793
Epoch 6/20
60000/60000 [==============================] - 3s 49us/step - loss: 0.0425 - acc: 0.9872 - val_loss: 0.0775 - val_acc: 0.9800
Epoch 7/20
60000/60000 [==============================] - 3s 49us/step - loss: 0.0390 - acc: 0.9886 - val_loss: 0.0840 - val_acc: 0.9813
Epoch 8/20
60000/60000 [==============================] - 3s 49us/step - loss: 0.0334 - acc: 0.9903 - val_loss: 0.0890 - val_acc: 0.9805
Epoch 9/20
60000/60000 [==============================] - 3s 49us/step - loss: 0.0310 - acc: 0.9904 - val_loss: 0.0909 - val_acc: 0.9818
Epoch 10/20
60000/60000 [==============================] - 3s 49us/step - loss: 0.0277 - acc: 0.9920 - val_loss: 0.0877 - val_acc: 0.9825
Epoch 11/20
60000/60000 [==============================] - 3s 49us/step - loss: 0.0264 - acc: 0.9924 - val_loss: 0.0905 - val_acc: 0.9832
Epoch 12/20
60000/60000 [==============================] - 3s 49us/step - loss: 0.0257 - acc: 0.9926 - val_loss: 0.0868 - val_acc: 0.9836
Epoch 13/20
60000/60000 [==============================] - 3s 49us/step - loss: 0.0232 - acc: 0.9936 - val_loss: 0.0944 - val_acc: 0.9837
Epoch 14/20
60000/60000 [==============================] - 3s 49us/step - loss: 0.0217 - acc: 0.9941 - val_loss: 0.1022 - val_acc: 0.9834
Epoch 15/20
60000/60000 [==============================] - 3s 49us/step - loss: 0.0223 - acc: 0.9938 - val_loss: 0.0952 - val_acc: 0.9816
Epoch 16/20
60000/60000 [==============================] - 3s 49us/step - loss: 0.0190 - acc: 0.9949 - val_loss: 0.1015 - val_acc: 0.9840
Epoch 17/20
60000/60000 [==============================] - 3s 49us/step - loss: 0.0197 - acc: 0.9946 - val_loss: 0.1161 - val_acc: 0.9848
Epoch 18/20
60000/60000 [==============================] - 3s 49us/step - loss: 0.0210 - acc: 0.9945 - val_loss: 0.1078 - val_acc: 0.9822
Epoch 19/20
60000/60000 [==============================] - 3s 49us/step - loss: 0.0190 - acc: 0.9950 - val_loss: 0.1235 - val_acc: 0.9832
Epoch 20/20
60000/60000 [==============================] - 3s 49us/step - loss: 0.0185 - acc: 0.9954 - val_loss: 0.0980 - val_acc: 0.9843
Test loss: 0.0980480428516
Test accuracy: 0.9843
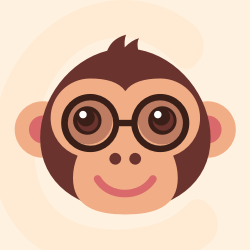



A beautiful web dashboard for Linux
最近提交(Master分支:3 个月前 )
186a802e
added ecosystem file for PM2 4 年前
5def40a3
Add host customization support for the NodeJS version 4 年前
更多推荐
所有评论(0)