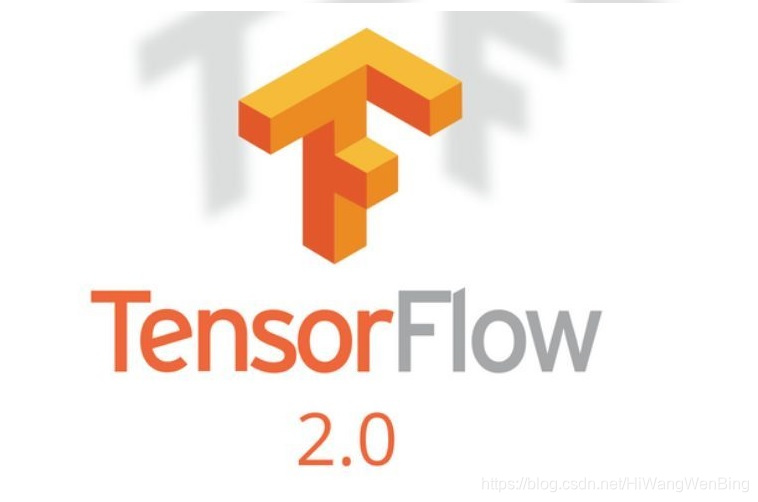
[TensorFlow系列-16]:TensorFlow基础 - 张量的操作 - 变形(降维、扩维、广播)

作者主页(文火冰糖的硅基工坊):https://blog.csdn.net/HiWangWenBing
本文网址:https://blog.csdn.net/HiWangWenBing/article/details/119651252
目录
2.1 tf.rank: 查看张量的阶数,即张量的维度方向数
2.2 tf.shape: 查看张量的形状,张量在各个维度方向长的长度
2.3 tf.size: 返回输入张量的size,即张量的元素数量或个数。
第3章 tf.reshape(tensor, shape): 改变张量形状,不改变元素顺序
第4章 tf.transpose(tensor):张量的转置
第5章 tf.expand_dims(input, axis): 不增加内容,增加一个维度
第6章 tf.squeeze(input, axis=None):删除维度
第7章 tf.broadcast_to(input, shape):在长度为1的维度上广播数据
第1章 Tensor运算与操作概述
https://tensorflow.google.cn/api_docs/python/tf
1.1 概述
TensorFlow提供了大量的张量运算与操作,基本上可以对标Numpy多维数组的运算,以支持对张量的各种复杂的运算。
这些操作运算中大多是对数组中每个元素执行相同的函数运算,并获得每个元素函数运算的结果序列,这些序列生成一个新的同维度的数组。
https://www.runoob.com/numpy/numpy-linear-algebra.html
不同维度张量的维度方向标识
- 随着张量维度的增加,张量维度的标识dim的范围也在扩宽
- 在张量维度扩展的过程中,维度标识值(dim=n)的含义也在发生变化。
- dim=0总是指向张量的多维数组存储的最外层:[ ] [ ] [ ], 这与物理存储的标识是相反的。
1.2 运算分类
(1)算术运算:加、减、系数乘、系数除
(2)函数运算:sin,cos
(3)取整运算:上取整、下取整
(4)统计运算:最大值、最小值、均值
(5)比较运算:大于,等于,小于、排序
(6)线性代数运算:矩阵、点乘、叉乘
1.3 张量的操作与变换
(1)变换内容: 变换张量元素的值。
(1)变换长度:变换张量的某个方向的长度(即向量的维度或长度),长度可增加,可减少。
(3)变换维度:变化张量的维度,维度可以增加,可减少。
1.4 环境准备
#环境准备
import numpy as np
import tensorflow as tf
print("hello world")
print("tensorflow version:", tf.__version__)
1.5 张量的操作 - 变形
所谓张量的变形,就是改变张量的形状。
形状包括:张量的维度+不同维度方向上的长度。
一般情况下,变形前后,张量的总元素的个数和内容不变。
变形相关的主要函数有:
(1)张量的形状显示
- tf.shape(input, name=None, out_type=tf.int32): 张量的形状
- tf.size(input, name=None, out_type=tf.int32):张量的总长度
- tf.rank(input, name=None)
(2)张量的形状改变,不改变元素的个数
- tf.reshape(tensor, shape, name=None): 改变张量的形状,不改变元素的顺序
- tf. 转置:改变张量的形状,也改变元素的顺序
第2章 张量的形状属性
2.1 tf.rank: 查看张量的阶数,即张量的维度方向数
tf.rank(input, name=None)
input:输入的张量
name:操作的名称
2.2 tf.shape: 查看张量的形状,张量在各个维度方向长的长度
tf.shape(input, name=None, out_type=tf.int32)
input:输入的张量
name:操作的名称
out_type:输出的类型(int32 or int64), 默认tf.int32
2.3 tf.size: 返回输入张量的size,即张量的元素数量或个数。
tf.size(input, name=None, out_type=tf.int32)
input:输入的张量
name:操作的名称
out_type:输出的类型 (int32 or int64),默认int32
2.4 代码示例
# 代码示例:
# 张量的属性
print("源张量:")
a = tf.constant([[1,3,5,7,9,11],[2,4,6,8,10,12]])
print(a)
print(a.shape)
print("\n张量的属性")
print(tf.rank(a)) # 张量的维度方向数,axis =0,1,2。。。。
print(tf.shape(a)) # 张量在各个维度方向长的长度
print(tf.size(a)) # 张量的所有元素个数,shape的各个分量的乘积。
输出:
源张量:
tf.Tensor(
[[ 1 3 5 7 9 11]
[ 2 4 6 8 10 12]], shape=(2, 6), dtype=int32)
(2, 6)
张量的属性
tf.Tensor(2, shape=(), dtype=int32)
tf.Tensor([2 6], shape=(2,), dtype=int32)
tf.Tensor(12, shape=(), dtype=int32)
第3章 tf.reshape(tensor, shape): 改变张量形状,不改变元素顺序
tf.reshape改变一个张量的形状,按照指定的shape返回一个新的张量。
要是形状的某个分量是特殊值-1,那么就会计算该维度的大小,使得总大小(元素数量)保持不变,特殊的,如果传入的形状是[-1],那么意味着把整个张量弄平为1维。
必须确保变形前和变形之后的总元素个数是相同的。
tf.reshape (tensor, shape, name=None)
- tensor:输入的张量,待被改变形状的张量
- shape:张量,必须是 int32, int64,决定了输出张量的形状
- name:操作的名称
3.1 降维变换
#代码示例:
# 降维变换
print("源张量:")
a = tf.constant([[1,3,5,7,9,11],[2,4,6,8,10,12]])
b = a
print(b)
print(tf.rank(b)) # rank=2,
print(tf.shape(b)) # shape = 2*6
print(tf.size(b)) # size =12
print("\n张量变形1")
b = tf.reshape(a, [1,12]) #
print(b)
print(tf.rank(b)) # rank=2
print(tf.shape(b)) # shape = 1*12
print(tf.size(b)) # size =12
输出:
源张量:
tf.Tensor(
[[ 1 3 5 7 9 11]
[ 2 4 6 8 10 12]], shape=(2, 6), dtype=int32)
tf.Tensor(2, shape=(), dtype=int32)
tf.Tensor([2 6], shape=(2,), dtype=int32)
tf.Tensor(12, shape=(), dtype=int32)
张量变形1
tf.Tensor([[ 1 3 5 7 9 11 2 4 6 8 10 12]], shape=(1, 12), dtype=int32)
tf.Tensor(2, shape=(), dtype=int32)
tf.Tensor([ 1 12], shape=(2,), dtype=int32)
tf.Tensor(12, shape=(), dtype=int32)
3.2 同维变换
#代码示例:
# 同维变换
print("源张量:")
a = tf.constant([[1,3,5,7,9,11],[2,4,6,8,10,12]])
b = a
print(b)
print(tf.rank(b)) # rank=2,
print(tf.shape(b)) # shape = 2*6
print(tf.size(b)) # size =12
print("\n张量变形2")
b = tf.reshape(a,[3,4])
print(b)
print(tf.rank(b)) # rank=2
print(tf.shape(b)) # shape = 3*4
print(tf.size(b)) # size =12
输出:
源张量:
tf.Tensor(
[[ 1 3 5 7 9 11]
[ 2 4 6 8 10 12]], shape=(2, 6), dtype=int32)
tf.Tensor(2, shape=(), dtype=int32)
tf.Tensor([2 6], shape=(2,), dtype=int32)
tf.Tensor(12, shape=(), dtype=int32)
张量变形2
tf.Tensor(
[[ 1 3 5 7]
[ 9 11 2 4]
[ 6 8 10 12]], shape=(3, 4), dtype=int32)
tf.Tensor(2, shape=(), dtype=int32)
tf.Tensor([3 4], shape=(2,), dtype=int32)
tf.Tensor(12, shape=(), dtype=int32)
3.3 升维变换
# 代码示例:
# 升维变换
print("源张量:")
a = tf.constant([[1,3,5,7,9,11],[2,4,6,8,10,12]])
b = a
print(b)
print(tf.rank(b)) # rank=2,
print(tf.shape(b)) # shape = 2*6
print(tf.size(b)) # size =12
print("\n张量变形3")
b = tf.reshape(a, [2,2,3])
print(b)
print(tf.rank(b)) # rank=3
print(tf.shape(b)) # shape = 2*2*3
print(tf.size(b)) # size =12
输出:
源张量:
tf.Tensor(
[[ 1 3 5 7 9 11]
[ 2 4 6 8 10 12]], shape=(2, 6), dtype=int32)
tf.Tensor(2, shape=(), dtype=int32)
tf.Tensor([2 6], shape=(2,), dtype=int32)
tf.Tensor(12, shape=(), dtype=int32)
张量变形3
tf.Tensor(
[[[ 1 3 5]
[ 7 9 11]]
[[ 2 4 6]
[ 8 10 12]]], shape=(2, 2, 3), dtype=int32)
tf.Tensor(3, shape=(), dtype=int32)
tf.Tensor([2 2 3], shape=(3,), dtype=int32)
tf.Tensor(12, shape=(), dtype=int32)
3.4 自动计算长度
#代码示例:
# -1的使用
print("源张量:")
a = tf.constant([[1,3,5,7,9,11],[2,4,6,8,10,12]])
b = a
print(b)
print(tf.rank(b)) # rank=2,
print(tf.shape(b)) # shape = 2*6
print(tf.size(b)) # size =12
print("\n张量变形4")
b = tf.reshape(a, [2,2,-1]) #自动计算剩余维度上的长度
print(b)
print(tf.rank(b)) # rank=3
print(tf.shape(b)) # shape = 2*2*3
print(tf.size(b)) # size =12
输出:
源张量:
tf.Tensor(
[[ 1 3 5 7 9 11]
[ 2 4 6 8 10 12]], shape=(2, 6), dtype=int32)
tf.Tensor(2, shape=(), dtype=int32)
tf.Tensor([2 6], shape=(2,), dtype=int32)
tf.Tensor(12, shape=(), dtype=int32)
张量变形4
tf.Tensor(
[[[ 1 3 5]
[ 7 9 11]]
[[ 2 4 6]
[ 8 10 12]]], shape=(2, 2, 3), dtype=int32)
tf.Tensor(3, shape=(), dtype=int32)
tf.Tensor([2 2 3], shape=(3,), dtype=int32)
tf.Tensor(12, shape=(), dtype=int32)
第4章 tf.transpose(tensor):张量的转置
转置是按照对称轴进行对换,而不是旋转。
4.1 一维转置
#代码演示
# 一维转置,还是自身
print("源张量:")
a = tf.constant([1,3,5,7,9,11])
b = a
print(b)
print(tf.rank(b))
print(tf.shape(b))
print(tf.size(b))
print("\n张量转置1:")
b = tf.transpose(a) # 一维转置,还是自身
print(b)
print(tf.rank(b))
print(tf.shape(b))
print(tf.size(b))
输出:
源张量:
tf.Tensor([ 1 3 5 7 9 11], shape=(6,), dtype=int32)
tf.Tensor(1, shape=(), dtype=int32)
tf.Tensor([6], shape=(1,), dtype=int32)
tf.Tensor(6, shape=(), dtype=int32)
张量转置1:
tf.Tensor([ 1 3 5 7 9 11], shape=(6,), dtype=int32)
tf.Tensor(1, shape=(), dtype=int32)
tf.Tensor([6], shape=(1,), dtype=int32)
tf.Tensor(6, shape=(), dtype=int32)
4.2 二维转置
# 输出
# 二维转置,行列互换
print("源张量:")
a = tf.constant([[1,3,5,7,9,11]])
b = a
print(b)
print(tf.rank(b))
print(tf.shape(b))
print(tf.size(b))
print("\n张量转置1:")
b = tf.transpose(a) # 二维转置,行列互换
print(b)
print(tf.rank(b))
print(tf.shape(b))
print(tf.size(b))
输出:
源张量:
tf.Tensor([[ 1 3 5 7 9 11]], shape=(1, 6), dtype=int32)
tf.Tensor(2, shape=(), dtype=int32)
tf.Tensor([1 6], shape=(2,), dtype=int32)
tf.Tensor(6, shape=(), dtype=int32)
张量转置1:
tf.Tensor(
[[ 1]
[ 3]
[ 5]
[ 7]
[ 9]
[11]], shape=(6, 1), dtype=int32)
tf.Tensor(2, shape=(), dtype=int32)
tf.Tensor([6 1], shape=(2,), dtype=int32)
tf.Tensor(6, shape=(), dtype=int32)
# 代码示例:
# 二维转置,行列互换
# transpose变形
print("源张量:")
a = tf.constant([[1,3,5,7,9,11],[2,4,6,8,10,12]])
b = a
print(b)
print(tf.rank(b))
print(tf.shape(b))
print(tf.size(b))
print("\n张量转置1:")
b = tf.transpose(a) #二维转置,行列互换
print(b)
print(tf.rank(b))
print(tf.shape(b))
print(tf.size(b))
源张量:
tf.Tensor(
[[ 1 3 5 7 9 11]
[ 2 4 6 8 10 12]], shape=(2, 6), dtype=int32)
tf.Tensor(2, shape=(), dtype=int32)
tf.Tensor([2 6], shape=(2,), dtype=int32)
tf.Tensor(12, shape=(), dtype=int32)
张量转置1:
tf.Tensor(
[[ 1 2]
[ 3 4]
[ 5 6]
[ 7 8]
[ 9 10]
[11 12]], shape=(6, 2), dtype=int32)
tf.Tensor(2, shape=(), dtype=int32)
tf.Tensor([6 2], shape=(2,), dtype=int32)
tf.Tensor(12, shape=(), dtype=int32)
4.3 三维转置
#代码示例:
# 三维转置
# transpose变形
print("源张量:")
a = tf.range(0,24)
print(a)
print(tf.rank(a))
print(tf.shape(a))
print(tf.size(a))
print("\n张量reshape:")
b = tf.reshape(a, [2,3,4]) # reshape成三维张量
print(b)
print(tf.rank(b))
print(tf.shape(b))
print(tf.size(b))
print("\n张量转置2:")
c = tf.transpose(b) #形状对称转置,而不是旋转
print(c)
print(tf.rank(c))
print(tf.shape(c))
print(tf.size(c))
输出:
源张量:
tf.Tensor([ 0 1 2 3 4 5 6 7 8 9 10 11 12 13 14 15 16 17 18 19 20 21 22 23], shape=(24,), dtype=int32)
tf.Tensor(1, shape=(), dtype=int32)
tf.Tensor([24], shape=(1,), dtype=int32)
tf.Tensor(24, shape=(), dtype=int32)
张量reshape:
tf.Tensor(
[[[ 0 1 2 3]
[ 4 5 6 7]
[ 8 9 10 11]]
[[12 13 14 15]
[16 17 18 19]
[20 21 22 23]]], shape=(2, 3, 4), dtype=int32)
tf.Tensor(3, shape=(), dtype=int32)
tf.Tensor([2 3 4], shape=(3,), dtype=int32)
tf.Tensor(24, shape=(), dtype=int32)
张量转置2:
tf.Tensor(
[[[ 0 12]
[ 4 16]
[ 8 20]]
[[ 1 13]
[ 5 17]
[ 9 21]]
[[ 2 14]
[ 6 18]
[10 22]]
[[ 3 15]
[ 7 19]
[11 23]]], shape=(4, 3, 2), dtype=int32)
tf.Tensor(3, shape=(), dtype=int32)
tf.Tensor([4 3 2], shape=(3,), dtype=int32)
tf.Tensor(24, shape=(), dtype=int32)
4.4 按照维度方向,任意转置
#代码示例:
任意的映射
# transpose变形
print("源张量:")
a = tf.range(0,24)
print(a)
print(tf.rank(a))
print(tf.shape(a))
print(tf.size(a))
print("\n张量reshape:")
b = tf.reshape(a, [2,3,4]) # reshape成三维张量, axis=0的长度为2, axis=1的长度为3, axis=3的长度为4
print(b)
print(tf.rank(b))
print(tf.shape(b))
print(tf.size(b))
print("\n张量转置:")
#新张量axis=0方向的数据来着源张量的axis=2.
#新张量axis=1方向的数据来着源张量的axis=0.
#新张量axis=2方向的数据来着源张量的axis=1.
c = tf.transpose(b, perm=[2,0,1])
print(c)
print(tf.rank(c))
print(tf.shape(c))
print(tf.size(c))
输出:
源张量:
tf.Tensor([ 0 1 2 3 4 5 6 7 8 9 10 11 12 13 14 15 16 17 18 19 20 21 22 23], shape=(24,), dtype=int32)
tf.Tensor(1, shape=(), dtype=int32)
tf.Tensor([24], shape=(1,), dtype=int32)
tf.Tensor(24, shape=(), dtype=int32)
张量reshape:
tf.Tensor(
[[[ 0 1 2 3]
[ 4 5 6 7]
[ 8 9 10 11]]
[[12 13 14 15]
[16 17 18 19]
[20 21 22 23]]], shape=(2, 3, 4), dtype=int32)
tf.Tensor(3, shape=(), dtype=int32)
tf.Tensor([2 3 4], shape=(3,), dtype=int32)
tf.Tensor(24, shape=(), dtype=int32)
张量转置:
tf.Tensor(
[[[ 0 4 8]
[12 16 20]]
[[ 1 5 9]
[13 17 21]]
[[ 2 6 10]
[14 18 22]]
[[ 3 7 11]
[15 19 23]]], shape=(4, 2, 3), dtype=int32)
tf.Tensor(3, shape=(), dtype=int32)
tf.Tensor([4 2 3], shape=(3,), dtype=int32)
tf.Tensor(24, shape=(), dtype=int32)
代码解读:
transpose改变形状:2*3*4 =》 4 * 2 * 3,改变元素在张量中的位置,不但调整了元素的形状结构,还挪动了整个维度方向上的数据。这种方式类似于公司的部门调整,把整个部门的人一起调动了位置,新部门的人员,在新的维度上,执行新的任务。
reshape改变形状: 2*3*4 =》 4 * 2 * 3, 不改变元素在张量中的位置,即元素的相对位置不变,只改变了组织和管理这些元素的逻辑方式。这种方式类似于公司的部门调整,所有的 人员(张量元素)不动,只是重选对人员进行编排,分组,重选规划分组后的部门(张量维度)名称。
4.5 任意维度转置的应用:
不同的深度学习框架,数据的物理组织方式不同,通过上述转置方式,可以实现不同深度学习框架,数据的转换。
如图片的组织方式:
Pytorch:[batch, channel, hight, width]
Tensorflow:[batch, high, widht, channel]
(1)如果源数据是Pytorch格式,目的数据是Tensorflow格式,这转换函数为:
Pytorch:[batch, channel, hight, width]
Tensorflow:[batch, high, widht, channel]
b = tf.transpose(a, perm=[0,2, 3, 1])
(2)如果源数据是Tensorflow格式,目的数据是Pytorch格式,这转换函数为:
Tensorflow:[batch, high, widht, channel]
Pytorch:[batch, channel, hight, width]
b = torch.transpose(a, perm=[0,3, 1, 2])
第5章 tf.expand_dims(input, axis): 不增加内容,增加一个维度
5.1 在axis=0的方向上增加一个维度
# 代码示例
# 新增一个维度方向,新增维度的向量长度为1
print("源张量:")
a = tf.constant([[1,3,5],[2,4,6]])
b = a
print(b)
print(tf.rank(b))
print(tf.shape(b))
print(tf.size(b))
print("\n扩维后张量axis=0:")
b = tf.expand_dims(a,axis=0)
print(b)
print(tf.rank(b))
print(tf.shape(b))
print(tf.size(b))
输出:
源张量:
tf.Tensor(
[[1 3 5]
[2 4 6]], shape=(2, 3), dtype=int32)
tf.Tensor(2, shape=(), dtype=int32)
tf.Tensor([2 3], shape=(2,), dtype=int32)
tf.Tensor(6, shape=(), dtype=int32)
扩维后张量axis=0:
tf.Tensor(
[[[1 3 5]
[2 4 6]]], shape=(1, 2, 3), dtype=int32)
tf.Tensor(3, shape=(), dtype=int32)
tf.Tensor([1 2 3], shape=(3,), dtype=int32)
tf.Tensor(6, shape=(), dtype=int32)
5.3 在axis=0的方向上增加一个维度
# 代码示例:
print("源张量:")
a = tf.constant([[1,3,5],[2,4,6]])
b = a
print(b)
print(tf.rank(b))
print(tf.shape(b))
print(tf.size(b))
print("\n扩维后张量axis=1:")
b = tf.expand_dims(a,axis=1)
print(b)
print(tf.rank(b))
print(tf.shape(b))
print(tf.size(b))
输出:
源张量:
tf.Tensor(
[[1 3 5]
[2 4 6]], shape=(2, 3), dtype=int32)
tf.Tensor(2, shape=(), dtype=int32)
tf.Tensor([2 3], shape=(2,), dtype=int32)
tf.Tensor(6, shape=(), dtype=int32)
扩维后张量axis=1:
tf.Tensor(
[[[1 3 5]]
[[2 4 6]]], shape=(2, 1, 3), dtype=int32)
tf.Tensor(3, shape=(), dtype=int32)
tf.Tensor([2 1 3], shape=(3,), dtype=int32)
tf.Tensor(6, shape=(), dtype=int32)
5.3 在axis=0的方向上增加一个维度
# 代码示例
print("源张量:")
a = tf.constant([[1,3,5],[2,4,6]])
b = a
print(b)
print(tf.rank(b))
print(tf.shape(b))
print(tf.size(b))
print("\n扩维后张量axis=2:")
b = tf.expand_dims(a,axis=2)
print(b)
print(tf.rank(b))
print(tf.shape(b))
print(tf.size(b))
输出:
源张量:
tf.Tensor(
[[1 3 5]
[2 4 6]], shape=(2, 3), dtype=int32)
tf.Tensor(2, shape=(), dtype=int32)
tf.Tensor([2 3], shape=(2,), dtype=int32)
tf.Tensor(6, shape=(), dtype=int32)
扩维后张量axis=2:
tf.Tensor(
[[[1]
[3]
[5]]
[[2]
[4]
[6]]], shape=(2, 3, 1), dtype=int32)
tf.Tensor(3, shape=(), dtype=int32)
tf.Tensor([2 3 1], shape=(3,), dtype=int32)
tf.Tensor(6, shape=(), dtype=int32)
第6章 tf.squeeze(input, axis=None):删除维度
6.1 压缩掉所有长度为1的维度, 元素个数不变
#代码示例
# squeeze剔除所有张量维度方向上长度为1的维度
print("源张量:")
a = tf.constant([[1,3,5,7,9,11],[2,4,6,8,10,12]])
print(a)
print(tf.rank(a))
print(tf.shape(a))
print(tf.size(a))
print("\n张量reshape(元素个数不变):")
b = tf.reshape(a, [1,1,12])
print(b)
print(tf.rank(b))
print(tf.shape(b))
print(tf.size(b))
print("\n张量压缩(元素个数不变):")
c = tf.squeeze(b)
print(c)
print(tf.rank(c))
print(tf.shape(c))
print(tf.size(c))
输出:
源张量:
tf.Tensor(
[[ 1 3 5 7 9 11]
[ 2 4 6 8 10 12]], shape=(2, 6), dtype=int32)
tf.Tensor(2, shape=(), dtype=int32)
tf.Tensor([2 6], shape=(2,), dtype=int32)
tf.Tensor(12, shape=(), dtype=int32)
张量reshape(元素个数不变):
tf.Tensor([[[ 1 3 5 7 9 11 2 4 6 8 10 12]]], shape=(1, 1, 12), dtype=int32)
tf.Tensor(3, shape=(), dtype=int32)
tf.Tensor([ 1 1 12], shape=(3,), dtype=int32)
tf.Tensor(12, shape=(), dtype=int32)
张量压缩(元素个数不变):
tf.Tensor([ 1 3 5 7 9 11 2 4 6 8 10 12], shape=(12,), dtype=int32)
tf.Tensor(1, shape=(), dtype=int32)
tf.Tensor([12], shape=(1,), dtype=int32)
tf.Tensor(12, shape=(), dtype=int32)
6.2 压缩掉长度为1的指定维度
# 代码示例:
# squeeze剔除张量维度方向上长度为1的维度
print("源张量:")
a = tf.constant([[1,3,5,7,9,11],[2,4,6,8,10,12]])
print(a)
print(tf.rank(a))
print(tf.shape(a))
print(tf.size(a))
print("\n张量reshape(元素个数不变):")
b = tf.reshape(a, [1,1,12])
print(b)
print(tf.rank(b))
print(tf.shape(b))
print(tf.size(b))
print("\n张量压缩(元素个数不变):")
c = tf.squeeze(b,axis=0)
print(c)
print(tf.rank(c))
print(tf.shape(c))
print(tf.size(c))
print("\n张量压缩(元素个数不变):")
c = tf.squeeze(b,axis=1)
print(c)
print(tf.rank(c))
print(tf.shape(c))
print(tf.size(c))
输出:
源张量:
tf.Tensor(
[[ 1 3 5 7 9 11]
[ 2 4 6 8 10 12]], shape=(2, 6), dtype=int32)
tf.Tensor(2, shape=(), dtype=int32)
tf.Tensor([2 6], shape=(2,), dtype=int32)
tf.Tensor(12, shape=(), dtype=int32)
张量reshape(元素个数不变):
tf.Tensor([[[ 1 3 5 7 9 11 2 4 6 8 10 12]]], shape=(1, 1, 12), dtype=int32)
tf.Tensor(3, shape=(), dtype=int32)
tf.Tensor([ 1 1 12], shape=(3,), dtype=int32)
tf.Tensor(12, shape=(), dtype=int32)
张量压缩(元素个数不变):
tf.Tensor([[ 1 3 5 7 9 11 2 4 6 8 10 12]], shape=(1, 12), dtype=int32)
tf.Tensor(2, shape=(), dtype=int32)
tf.Tensor([ 1 12], shape=(2,), dtype=int32)
tf.Tensor(12, shape=(), dtype=int32)
张量压缩(元素个数不变):
tf.Tensor([[ 1 3 5 7 9 11 2 4 6 8 10 12]], shape=(1, 12), dtype=int32)
tf.Tensor(2, shape=(), dtype=int32)
tf.Tensor([ 1 12], shape=(2,), dtype=int32)
tf.Tensor(12, shape=(), dtype=int32)
第7章 tf.broadcast_to(input, shape):在长度为1的维度上广播数据
广播的本质是增加某个维度方向数据长度,实际上是把长度1的维度方向上的数据,复制多份,其维度方向上数据的长度。
7.1 在以有长度为1的维度上广播数据
#代码示例:
print("源张量:")
a = tf.constant([1,3,5,7,9,11])
print(a)
print(tf.rank(a))
print(tf.shape(a))
print(tf.size(a))
print("\n在axis=0上扩充一个维度:")
b = tf.expand_dims(a, axis=0)
print(b)
print(tf.rank(b))
print(tf.shape(b))
print(tf.size(b))
print("\n只能在长度为1的维度方向上进行广播,增加其长度:")
# 把1*6的张量广播成4*6的张量
# 新加长度的数据,来自于原先的维度中数据
b = tf.broadcast_to(b,shape=[4,6])
print(b)
print(tf.rank(b))
print(tf.shape(b))
print(tf.size(b))
输出:
源张量:
tf.Tensor([ 1 3 5 7 9 11], shape=(6,), dtype=int32)
tf.Tensor(1, shape=(), dtype=int32)
tf.Tensor([6], shape=(1,), dtype=int32)
tf.Tensor(6, shape=(), dtype=int32)
在axis=0上扩充一个维度:
tf.Tensor([[ 1 3 5 7 9 11]], shape=(1, 6), dtype=int32)
tf.Tensor(2, shape=(), dtype=int32)
tf.Tensor([1 6], shape=(2,), dtype=int32)
tf.Tensor(6, shape=(), dtype=int32)
只能在长度为1的维度方向上进行广播,增加其长度:
tf.Tensor(
[[ 1 3 5 7 9 11]
[ 1 3 5 7 9 11]
[ 1 3 5 7 9 11]
[ 1 3 5 7 9 11]], shape=(4, 6), dtype=int32)
tf.Tensor(2, shape=(), dtype=int32)
tf.Tensor([4 6], shape=(2,), dtype=int32)
tf.Tensor(24, shape=(), dtype=int32)
7.2 先扩维后广播
#代码示例:
print("源张量:")
a = tf.constant([[1,3,5,7,9,11],[2,4,6,8,10,12]])
print(a)
print(tf.rank(a))
print(tf.shape(a))
print(tf.size(a))
print("\n广播后,维度不变,长度变化:")
# 把2*3的张量广播成4*6的张量
# 新加长度的数据,来自于原先的维度中数据
c = tf.broadcast_to(a,shape=[4,2,6])
print(c)
print(tf.rank(c))
print(tf.shape(c))
print(tf.size(c))
输出:
源张量:
tf.Tensor(
[[ 1 3 5 7 9 11]
[ 2 4 6 8 10 12]], shape=(2, 6), dtype=int32)
tf.Tensor(2, shape=(), dtype=int32)
tf.Tensor([2 6], shape=(2,), dtype=int32)
tf.Tensor(12, shape=(), dtype=int32)
广播后,维度不变,长度变化:
tf.Tensor(
[[[ 1 3 5 7 9 11]
[ 2 4 6 8 10 12]]
[[ 1 3 5 7 9 11]
[ 2 4 6 8 10 12]]
[[ 1 3 5 7 9 11]
[ 2 4 6 8 10 12]]
[[ 1 3 5 7 9 11]
[ 2 4 6 8 10 12]]], shape=(4, 2, 6), dtype=int32)
tf.Tensor(3, shape=(), dtype=int32)
tf.Tensor([4 2 6], shape=(3,), dtype=int32)
tf.Tensor(48, shape=(), dtype=int32)
备注:长度为4的维度是先扩维,广播数据,增加其长度。
作者主页(文火冰糖的硅基工坊):https://blog.csdn.net/HiWangWenBing
本文网址:https://blog.csdn.net/HiWangWenBing/article/details/119651252
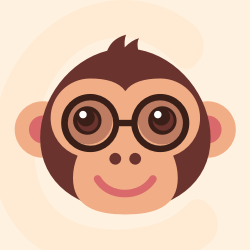



更多推荐
所有评论(0)