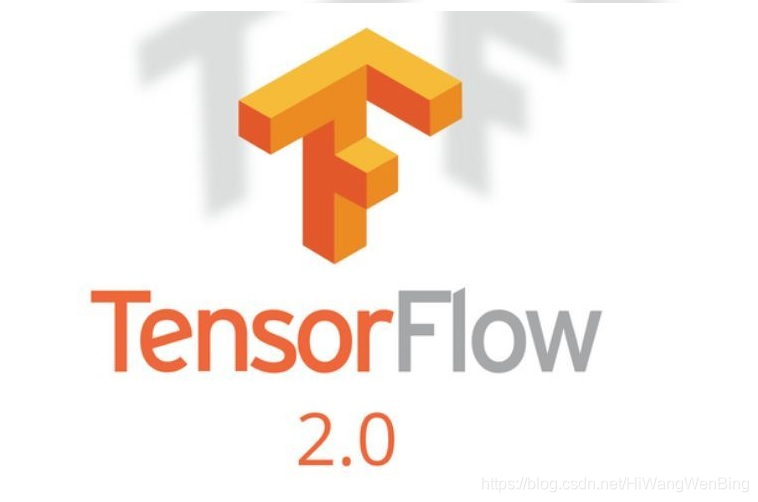
[TensorFlow系列-15]:TensorFlow基础 - 张量的操作 - 拆分与分割

作者主页(文火冰糖的硅基工坊):https://blog.csdn.net/HiWangWenBing
本文网址:https://blog.csdn.net/HiWangWenBing/article/details/119651181
目录
第2章 均匀降低维度的拆分:tf.unstack(value, num=None, axis=0)
第3章 非均匀降低维度的拆分:tf.split(value, num_or_size_splits, axis=0)
第1章 Tensor运算概述
https://tensorflow.google.cn/api_docs/python/tf
1.1 概述
TensorFlow提供了大量的张量运算,基本上可以对标Numpy多维数组的运算,以支持对张量的各种复杂的运算。
这些操作运算中大多是对数组中每个元素执行相同的函数运算,并获得每个元素函数运算的结果序列,这些序列生成一个新的同维度的数组。
不同维度张量的维度方向标识
- 随着张量维度的增加,张量维度的标识dim的范围也在扩宽
- 在张量维度扩展的过程中,维度标识值(dim=n)的含义也在发生变化。
- dim=0总是指向张量的多维数组存储的最外层:[ ] [ ] [ ], 这与物理存储的标识是相反的。
1.2 运算分类
(1)算术运算:加、减、系数乘、系数除
(2)函数运算:sin,cos
(3)取整运算:上取整、下取整
(4)统计运算:最大值、最小值、均值
(5)比较运算:大于,等于,小于、排序
(6)线性代数运算:矩阵、点乘、叉乘
1.3 张量的操作与变换
(1)变换内容: 变换张量元素的值。
(1)变换长度:变换张量的某个方向的长度(即向量的维度或长度),长度可增加,可减少。
(3)变换维度:变化张量的维度,维度可以增加,可减少。
1.4 环境准备
#环境准备
import numpy as np
import tensorflow as tf
print("hello world")
print("tensorflow version:", tf.__version__)
1.5 张量的操作 - 拆分与分割
张量的切分有两种主要的基本策略:
- 在某个维度方向,平均分割:tf.unstack(value, num=None, axis=0)
- 在某个维度方向,指定分割:tf.split(value, num_or_size_splits, axis=0)
第2章 均匀降低维度的拆分:tf.unstack(value, num=None, axis=0)
2.1 函数说明
功能:把一个张量,在某个维度方向上,拆成多个相同维度、相同长度的等分的张量。
原型:unstack(values, num,axis)
输入参数:
values: 输入张量
num:分拆后张量的个数(均匀分拆)
axis:分拆的维度方向
2.2 代码示例
# 张量的拼接:增加阶数,
a = tf.constant([[1,1,1,1], [2,2,2,2],[3,3,3,3]])
print("源张量a")
print(a)
print(tf.rank(a))
print(tf.shape(a))
print(tf.size(a))
print("\n降维张量axis=0")
b = tf.unstack(a, axis=0)
print(b)
print("\n降维张量axis=1")
b = tf.unstack(a, num=4, axis=1)
print(b)
输出:
源张量a
tf.Tensor(
[[1 1 1 1]
[2 2 2 2]
[3 3 3 3]], shape=(3, 4), dtype=int32)
tf.Tensor(2, shape=(), dtype=int32)
tf.Tensor([3 4], shape=(2,), dtype=int32)
tf.Tensor(12, shape=(), dtype=int32)
降维张量axis=0
[<tf.Tensor: shape=(4,), dtype=int32, numpy=array([1, 1, 1, 1])>, <tf.Tensor: shape=(4,), dtype=int32, numpy=array([2, 2, 2, 2])>, <tf.Tensor: shape=(4,), dtype=int32, numpy=array([3, 3, 3, 3])>]
降维张量axis=1
[<tf.Tensor: shape=(3,), dtype=int32, numpy=array([1, 2, 3])>, <tf.Tensor: shape=(3,), dtype=int32, numpy=array([1, 2, 3])>, <tf.Tensor: shape=(3,), dtype=int32, numpy=array([1, 2, 3])>, <tf.Tensor: shape=(3,), dtype=int32, numpy=array([1, 2, 3])>]
第3章 非均匀降低维度的拆分:tf.split(value, num_or_size_splits, axis=0)
3.1 函数说明
功能:把一个张量,在某个维度方向上,拆分成不同长度的相同维度的张量。
原型:split(values, num_or_size_splits,axis)
输入参数:
values: 输入张量
num_or_size_splits:在拆分维度上,多个不同的长度列表。
axis:分拆的维度方向
3.2 代码示例
# 代码示例:
# 张量的拼接:增加阶数,
a = tf.constant([[1,1,1,1], [2,2,2,2],[3,3,3,3]])
print("源张量a")
print(a)
print(tf.rank(a))
print(tf.shape(a))
print(tf.size(a))
print("\n降维张量axis=0")
b = tf.split(a, num_or_size_splits=[1,2], axis=0) #分割长分别为1和2的两个张量。
print(b)
print("\n降维张量axis=1")
b = tf.split(a, num_or_size_splits = 2 ,axis=1) #分割成2个长度相等的张量
print(b)
print("\n降维张量axis=1")
b = tf.split(a, num_or_size_splits = [1,2,1] ,axis=1) #分割成长度为 1、2、1三个张量
print(b)
输出:
源张量a
tf.Tensor(
[[1 1 1 1]
[2 2 2 2]
[3 3 3 3]], shape=(3, 4), dtype=int32)
tf.Tensor(2, shape=(), dtype=int32)
tf.Tensor([3 4], shape=(2,), dtype=int32)
tf.Tensor(12, shape=(), dtype=int32)
降维张量axis=0
[<tf.Tensor: shape=(1, 4), dtype=int32, numpy=array([[1, 1, 1, 1]])>, <tf.Tensor: shape=(2, 4), dtype=int32, numpy=
array([[2, 2, 2, 2],
[3, 3, 3, 3]])>]
降维张量axis=1
[<tf.Tensor: shape=(3, 2), dtype=int32, numpy=
array([[1, 1],
[2, 2],
[3, 3]])>, <tf.Tensor: shape=(3, 2), dtype=int32, numpy=
array([[1, 1],
[2, 2],
[3, 3]])>]
降维张量axis=1
[<tf.Tensor: shape=(3, 1), dtype=int32, numpy=
array([[1],
[2],
[3]])>, <tf.Tensor: shape=(3, 2), dtype=int32, numpy=
array([[1, 1],
[2, 2],
[3, 3]])>, <tf.Tensor: shape=(3, 1), dtype=int32, numpy=
array([[1],
[2],
[3]])>]
作者主页(文火冰糖的硅基工坊):https://blog.csdn.net/HiWangWenBing
本文网址:https://blog.csdn.net/HiWangWenBing/article/details/119651181
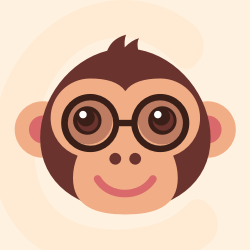



更多推荐
所有评论(0)