tensorflow 任意 batch size 不溢出显存( OOM ),使用 darknet 的 sub batch 方法
tensorflow
一个面向所有人的开源机器学习框架
项目地址:https://gitcode.com/gh_mirrors/te/tensorflow

·
这方法很久之前就想弄了,网上( 百度 )除了 darknet 之外,没人弄这东西,无奈那时对 tf 的 Optimizer 和 梯度计算 理解很浅,没法弄,不久前看了个 tensorflow 的 eager run 的例子才弄懂 tf 的梯度计算方式。
原理很简单,例如一个 batch 的 size = 100,直接放进显卡会溢出,那我把这个 batch 再分成 10 个 sub batch,每个 sub batch 的 size = 10,先分别放进显卡计算梯度,然后累加到梯度缓存中,然后对梯度缓存求平均,再应用梯度。这样的效果跟batch size 为 100 是一样的,区别在于时间。拿时间换空间
这个方法的只有一个必要的要求,batch size = 1 的时候不能溢出。如果 batch size = 1 都溢出了,只能放 cpu 跑了。。
把 batch size 设置为 数据集大小,就变成 真 批量下降算法了
import tensorflow as tf
import tensorlayer as tl
import numpy as np
from progressbar import progressbar
import time
# 加载数据集
x_dataset, y_dataset = tl.files.load_fashion_mnist_dataset((-1, 28, 28, 1), '../datasets')[:2]
act = tf.nn.leaky_relu
epoch = 200
batch_size = 5000
n_batch = len(x_dataset) // batch_size
# 把 batch 分成多少个 sub batch 来计算
subdivisions = 50
subdivisions_batch_size = int(np.ceil(batch_size / subdivisions))
# 是否使用 sub batch 方法,设置为 False 代表使用默认方法
is_on_subdivisions = True
def get_model(x, is_train=True, reuse=False):
with tf.variable_scope('model', reuse=reuse):
net = tl.layers.InputLayer(x)
net = tl.layers.Conv2d(net, 128, (3, 3), (2, 2), None, 'SAME', b_init=None, name='c1')
net = tl.layers.BatchNormLayer(net, act=act, is_train=is_train, name='b1')
net = tl.layers.Conv2d(net, 128*2, (3, 3), (2, 2), None, 'SAME', b_init=None, name='c2')
net = tl.layers.BatchNormLayer(net, act=act, is_train=is_train, name='b2')
net = tl.layers.Conv2d(net, 128*3, (3, 3), (1, 1), None, 'SAME', b_init=None, name='c3')
net = tl.layers.BatchNormLayer(net, act=act, is_train=is_train, name='b3')
net = tl.layers.Conv2d(net, 128*4, (3, 3), (1, 1), None, 'SAME', b_init=None, name='c4')
net = tl.layers.BatchNormLayer(net, act=act, is_train=is_train, name='b4')
net = tl.layers.Conv2d(net, 128*5, (3, 3), (1, 1), None, 'SAME', b_init=None, name='c5')
net = tl.layers.BatchNormLayer(net, act=act, is_train=is_train, name='b5')
net = tl.layers.GlobalMeanPool2d(net)
net = tl.layers.DenseLayer(net, 10, None)
return net
x = tf.placeholder(tf.float32, [None, 28, 28, 1])
y = tf.placeholder(tf.int32, [None,])
net = get_model(x)
loss_op = tf.losses.sparse_softmax_cross_entropy(y, net.outputs)
optim = tf.train.AdamOptimizer(0.01)
grads_vars = optim.compute_gradients(loss_op, net.all_params)
# 删掉没梯度的参数, 倒序删除,减少麻烦
for i in range(len(grads_vars))[::-1]:
if grads_vars[i][0] is None:
del grads_vars[i]
# 生成梯度缓存
grads_cache = [tf.Variable(np.zeros(t[0].shape.as_list(), np.float32), trainable=False) for t in grads_vars]
# 清空梯度缓存op,每一 batch 开始前调用
clear_grads_cache_op = tf.group([gc.assign(tf.zeros_like(gc)) for gc in grads_cache])
# 累积梯度op,累积每个 sub batch 的梯度
accumulate_grad_op = tf.group([gc.assign_add(gv[0]) for gc, gv in zip(grads_cache, grads_vars)])
# 求平均梯度,
mean_grad = [gc/tf.to_float(subdivisions) for gc in grads_cache]
# 组装梯度列表
new_grads_vars = [(g, gv[1]) for g, gv in zip(mean_grad, grads_vars)]
# 应用梯度op,累积完所有 sub batch 的梯度后,应用梯度
apply_grad_op = optim.apply_gradients(new_grads_vars)
# 原来的 optim ,跟上面做对照
ori_optim_op = tf.train.AdamOptimizer(0.01).minimize(loss_op, var_list=net.all_params)
config = tf.ConfigProto()
config.gpu_options.allow_growth = True
config.allow_soft_placement = True
sess = tf.Session(config=config)
sess.run(tf.global_variables_initializer())
for e in range(epoch):
loss_sum = 0
for b in progressbar(range(n_batch)):
x_batch = x_dataset[b * batch_size: (b + 1) * batch_size]
y_batch = y_dataset[b * batch_size: (b + 1) * batch_size]
if is_on_subdivisions:
# 每一批开始前需要清空梯度缓存
sess.run(clear_grads_cache_op)
sub_loss_sum = 0
for s in range(subdivisions):
x_sub_batch = x_batch[s * subdivisions_batch_size: (s + 1) * subdivisions_batch_size]
y_sub_batch = y_batch[s * subdivisions_batch_size: (s + 1) * subdivisions_batch_size]
if len(x_sub_batch) == 0:
break
feed_dict = {x: x_sub_batch, y: y_sub_batch}
_, los = sess.run([accumulate_grad_op, loss_op], feed_dict)
sub_loss_sum += los
loss_sum += sub_loss_sum / subdivisions
# 梯度累积完成,开始应用梯度
sess.run(apply_grad_op)
# 本批次结束
else:
feed_dict = {x: x_batch, y: y_batch}
_, los = sess.run([ori_optim_op, loss_op], feed_dict)
loss_sum += los
time.sleep(0.2)
print('loss', loss_sum / n_batch)
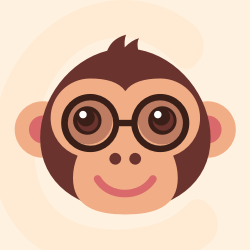



一个面向所有人的开源机器学习框架
最近提交(Master分支:2 个月前 )
a49e66f2
PiperOrigin-RevId: 663726708
2 个月前
91dac11a
This test overrides disabled_backends, dropping the default
value in the process.
PiperOrigin-RevId: 663711155
2 个月前
更多推荐
所有评论(0)