tensorflow中使用GPU的设置方法
tensorflow
一个面向所有人的开源机器学习框架
项目地址:https://gitcode.com/gh_mirrors/te/tensorflow

·
方法一:
Config=tf.ConfigProto(allow_soft_placement=True) ##:如果你指定的设备不存在,允许TF自动分配设备
Config.gpu_options.allow_growth=True ##动态分配内存
sess=tf.session(config=config)
方法二:
gpu_options = tf.GPUOptions(allow_growth=True)
gpu_options =tf.GPUOptions(per_process_gpu_memory_fraction=0.8,allow_growth=True) ##每个gpu占用0.8 的显存
config=tf.ConfigProto(gpu_options=gpu_options,allow_soft_placement=True)
sess=tf.session(config=config)##如果电脑有多个GPU,tensorflow默认全部使用。如果想只使用部分GPU,可以设置CUDA_VISIBLE_DEVICES。
控制使用哪个gpu
os.environ[“CUDA_DEVICE_ORDER”] = “PCI_BUS_ID” ##指定的设备名称
os.environ[‘CUDA_VISIBLE_DEVICES’] = ‘0’ #使用 GPU 0
os.environ[‘CUDA_VISIBLE_DEVICES’] = ‘0,1’ # 使用 GPU 0,1
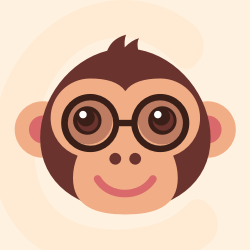



一个面向所有人的开源机器学习框架
最近提交(Master分支:1 个月前 )
a49e66f2
PiperOrigin-RevId: 663726708
1 个月前
91dac11a
This test overrides disabled_backends, dropping the default
value in the process.
PiperOrigin-RevId: 663711155
1 个月前
更多推荐
所有评论(0)