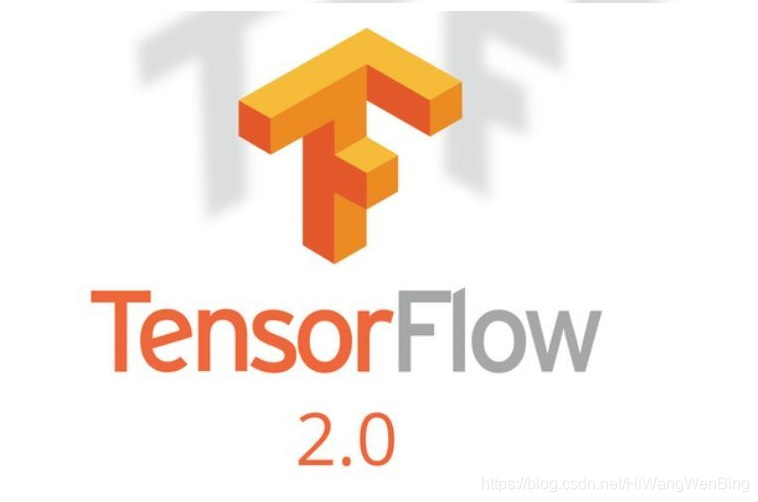
[Tensorflow系列-3]:Tensorflow基础 - Hello World程序与张量(Tensor)概述

作者主页(文火冰糖的硅基工坊):https://blog.csdn.net/HiWangWenBing
本文网址:https://blog.csdn.net/HiWangWenBing/article/details/119580837
目录
1. 导入库及检查版本
import numpy as np
import tensorflow as tf
2. Hello World
print("Hello world")
print("tensorflow version:", tf.__version__)
3. 基本数据单元
3.1 Tensorflow支持的数据结构
(1)张量:tensor (Torch定义了专门的结构)
从Python的List [ ]的数据结构 =》 Numpy.Array =》 Tensorflow的Tensor
- Python list:
数据元素可以是任意类型,数据元素的数据类型可以不相同。
- Numpy.Array:
Numpy数组元素的数据类型是相同的,且必须是数值类型,且通常是2维的,且支持在CPU上运行。
- Tensorflow Tensor:
与Numpy.array一样,Tensor中的元素的数据类型是必须相同的,与Numpy大体相同。
但Tensor在Numpy.array的基础上,进行了增强:
Tensor中的数据元素可以是任意维度的
Tensor可以支持在GPU上执行
支持自动求导等于深度学习相干的操作。
(2)变量:variable
Python的变量variable =》 深度学习框架的variable
深度学习框架定义了自己专有的variable变量,与Python的变量的区别是 深度学的variable用于存放升级网络的w和b的参数,是可以自动求导数的。
(3)神经网络:nn (深度学习定义了专门用于神经网络的数据结构)
3.2 数据的类型:dtype=tf.xxx
3.3 数据内容: [[xx,xx], [xx,xx]]
数据的内容,以多维数组的形式[[xx,xx], [xx,xx]]提供给Tensor。
3.4 数据运算的设备:device="xxx"
4. Tensor实例/样本创建概述
与PyTroch不同的是,Tensorflow使用的不是Tensor类模板来创建张量,也没有使用专有的名字“tensor”,而是使用了constant这个专有名词。constant实际上是一个函数名,而不是类模板的名字。
5. Tensor的成员
5.1 Tensor的公共属性
- xxx.dtype:Tensor数据类型, int、float、?
- xxx.device:执行的处理器,CPU or GPU
xxx.layout:内存布局,稀疏压缩存储和稠密非压缩存储。- xxx.shape:张量的形状(维度)
(1)属性的读
#代码示例
#直接构建Tensor内容和维度,内容数据是确定的
a = tf.constant([[1,2],[3,4]])
print(a)
print("\n读取tensor的属性")
print(a.device)
# print(a.layout) #不支持
print(a.dtype)
# print(a.type) #不支持
# print(a.type()) #不支持
# print(a.size) #不支持
print(a.shape)
#输出结果
tf.Tensor(
[[1 2]
[3 4]], shape=(2, 2), dtype=int32)
读取tensor的属性
/job:localhost/replica:0/task:0/device:CPU:0
<dtype: 'int32'>
(2, 2)
(2)属性的配
- 通过tensor函数进行配置
print("设置数据类型属性")
a = tf.constant([1,2,3], dtype=tf.float32)
print(a)
print (a.dtype)
输出:
设置数据类型属性
tf.Tensor([1. 2. 3.], shape=(3,), dtype=float32)
<dtype: 'float32'>
print("\n设置tensor的device属性:")
a.cpu() #设置device为CPU
print(a)
print(a.device)
输出:
设置tensor的device属性:
tf.Tensor([1. 2. 3.], shape=(3,), dtype=float32)
/job:localhost/replica:0/task:0/device:CPU:0
print("\n设置tensor的device属性:")
a.gpu() #设置device为GPU
print(a)
print(a.device)
tf.Tensor(
[[1 2]
[3 4]], shape=(2, 2), dtype=int32)
/job:localhost/replica:0/task:0/device:CPU:0
设置tensor的device属性:
.......
RuntimeError: GPU:0 unknown device.
备注:
由于没有安装GPU的环境,因此设置gpu device失败。
5.2 Tensor的公共操作
type(): 如上图size(): 如上图
5.3 Tenosor的运算方法
(1)使用重载后的Python运算符:+、-
(2)使用Torch提供的通用方法:tf.add、tf.sub......
(3)使用Tensor实例自带的成员函数xxx.add()、xxx.sub => 不修改tensor实例的值 # 不支持
(4)使用Tensor实例自带“in place”运算: xxx_add()、xxx.sub_=> 直接修改tensor实例的值
也就是说,要完成Tensor的某种运算,有很多种方法和手段。
但tensorflow而言,比Pytorch的运算方法要少,后两者方法在tensorflow中是不支持的。
# 代码示例:
a = tf.constant([1,2,3,4])
print(a)
b = tf.constant([5,6,7,8])
print(b)
print("\n运算符法:")
c = a + b
print(c)
print("\n全局函数法:")
c = tf.add(a,b)
print(c)
print("\n成员函数法:")
# c = a.add(b) # 不支持
# print(c)
输出:
tf.Tensor([1 2 3 4], shape=(4,), dtype=int32)
tf.Tensor([5 6 7 8], shape=(4,), dtype=int32)
运算符法:
tf.Tensor([ 6 8 10 12], shape=(4,), dtype=int32)
全局函数法:
tf.Tensor([ 6 8 10 12], shape=(4,), dtype=int32)
成员函数法:
# tensorflow不支持
作者主页(文火冰糖的硅基工坊):https://blog.csdn.net/HiWangWenBing
本文网址:https://blog.csdn.net/HiWangWenBing/article/details/119580837
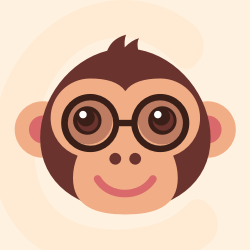



更多推荐
所有评论(0)