Tensorflow快餐教程(13) - 程序员学深度学习快速入门五步法

程序员学深度学习快速入门五步法
作为一个程序员,我们可以像学习编程一样学习深度学习模型开发。我们以Keras为例来说明。
我们可以用5步 + 4种基本元素 + 9种基本层结构,这5-4-9模型来总结。
5步法:
1. 构造网络模型
2. 编译模型
3. 训练模型
4. 评估模型
5. 使用模型进行预测
4种基本元素:
1. 网络结构:由10种基本层结构和其他层结构组成
2. 激活函数:如relu, softmax。口诀: 最后输出用softmax,其余基本都用relu
3. 损失函数:categorical_crossentropy多分类对数损失,binary_crossentropy对数损失,mean_squared_error平均方差损失, mean_absolute_error平均绝对值损失
4. 优化器:如SGD随机梯度下降, RMSProp, Adagrad, Adam, Adadelta等
9种基本层模型
包括3种主模型:
1. 全连接层Dense
2. 卷积层:如conv1d, conv2d
3. 循环层:如lstm, gru
3种辅助层:
1. Activation层
2. Dropout层
3. 池化层
3种异构网络互联层:
1. 嵌入层:用于第一层,输入数据到其他网络的转换
2. Flatten层:用于卷积层到全连接层之间的过渡
3. Permute层:用于RNN与CNN之间的接口
我们通过一张图来理解下它们之间的关系
五步法
五步法是用深度学习来解决问题的五个步骤:
1. 构造网络模型
2. 编译模型
3. 训练模型
4. 评估模型
5. 使用模型进行预测
在这五步之中,其实关键的步骤主要只有第一步,这一步确定了,后面的参数都可以根据它来设置。
过程化方法构造网络模型
我们先学习最容易理解的,过程化方法构造网络模型的过程。
Keras中提供了Sequential容器来实现过程式构造。只要用Sequential的add方法把层结构加进来就可以了。10种基本层结构我们会在后面详细讲。
例:
from keras.models import Sequential
from keras.layers import Dense, Activation
model = Sequential()
model.add(Dense(units=64, input_dim=100))
model.add(Activation("relu"))
model.add(Dense(units=10))
model.add(Activation("softmax"))
对于什么样的问题构造什么样的层结构,我们会在后面的例子中介绍。
编译模型
模型构造好之后,下一步就可以调用Sequential的compile方法来编译它。
model.compile(loss='categorical_crossentropy', optimizer='sgd', metrics=['accuracy'])
编译时需要指定两个基本元素:loss是损失函数,optimizer是优化函数。
如果只想用最基本的功能,只要指定字符串的名字就可以了。如果想配置更多的参数,调用相应的类来生成对象。例:我们想为随机梯度下降配上Nesterov动量,就生成一个SGD的对象就好了:
from keras.optimizers import SGD
model.compile(loss='categorical_crossentropy', optimizer=SGD(lr=0.01, momentum=0.9, nesterov=True))
lr是学习率,learning rate。这几个概念我们在《Tensorflow快餐教程(7) - 梯度下降》中曾经介绍过,需要复习的同学可以移步。
训练模型
调用fit函数,将输出的值X,打好标签的值y,epochs训练轮数,batch_size批次大小设置一下就可以了:
model.fit(x_train, y_train, epochs=5, batch_size=32)
评估模型
模型训练的好不好,训练数据不算数,需要用测试数据来评估一下:
loss_and_metrics = model.evaluate(x_test, y_test, batch_size=128)
用模型来预测
一切训练的目的是在于预测:
classes = model.predict(x_test, batch_size=128)
4种基本元素
网络结构
主要用后面的层结构来拼装。网络结构如何设计呢? 可以参考论文,比如这篇中不管是左边的19层的VGG-19,还是右边34层的resnet,只要按图去实现就好了。
激活函数
对于多分类的情况,最后一层是softmax。
其它深度学习层中多用relu。
二分类可以用sigmoid。
另外浅层神经网络也可以用tanh。
损失函数
- categorical_crossentropy:多分类对数损失
- binary_crossentropy:对数损失
- mean_squared_error:均方差
- mean_absolute_error:平均绝对值损失
对于多分类来说,主要用categorical_crossentropy。
优化器
- SGD:随机梯度下降
- Adagrad:Adaptive Gradient自适应梯度下降
- Adadelta:对于Adagrad的进一步改进
- RMSProp
- Adam
前三种在《Tensorflow快餐教程(7) - 梯度下降》中已经介绍过,后两种在后面的教程中会补充介绍。
深度学习中的函数式编程
前面介绍的各种基本层,除了可以add进Sequential容器串联之外,它们本身也是callable对象,被调用之后,返回的还是callable对象。所以可以将它们视为函数,通过调用的方式来进行串联。
来个官方例子:
from keras.layers import Input, Dense
from keras.models import Model
inputs = Input(shape=(784,))
x = Dense(64, activation='relu')(inputs)
x = Dense(64, activation='relu')(x)
predictions = Dense(10, activation='softmax')(x)
model = Model(inputs=inputs, outputs=predictions)
model.compile(optimizer='rmsprop',
loss='categorical_crossentropy',
metrics=['accuracy'])
model.fit(data, labels)
为什么要用函数式编程?
答案是,复杂的网络结构并不是都是线性的add进容器中的。并行的,重用的,什么情况都有。这时候callable的优势就发挥出来了。
比如下面的Google Inception模型,就是带并联的:
我们的代码自然是以并联应对并联了,一个输入input_img被三个模型所重用:
from keras.layers import Conv2D, MaxPooling2D, Input
input_img = Input(shape=(256, 256, 3))
tower_1 = Conv2D(64, (1, 1), padding='same', activation='relu')(input_img)
tower_1 = Conv2D(64, (3, 3), padding='same', activation='relu')(tower_1)
tower_2 = Conv2D(64, (1, 1), padding='same', activation='relu')(input_img)
tower_2 = Conv2D(64, (5, 5), padding='same', activation='relu')(tower_2)
tower_3 = MaxPooling2D((3, 3), strides=(1, 1), padding='same')(input_img)
tower_3 = Conv2D(64, (1, 1), padding='same', activation='relu')(tower_3)
output = keras.layers.concatenate([tower_1, tower_2, tower_3], axis=1)
案例教程
CNN处理MNIST手写识别
光说不练是假把式。我们来看看符合五步法的处理MNIST的例子。
首先解析一下核心模型代码,因为模型是线性的,我们还是用Sequential容器
model = Sequential()
核心是两个卷积层:
model.add(Conv2D(32, kernel_size=(3, 3),
activation='relu',
input_shape=input_shape))
model.add(Conv2D(64, (3, 3), activation='relu'))
为了防止过拟合,我们加上一个最大池化层,再加上一个Dropout层:
model.add(MaxPooling2D(pool_size=(2, 2)))
model.add(Dropout(0.25))
下面要进入全连接层输出了,这两个中间的数据转换需要一个Flatten层:
model.add(Flatten())
下面是全连接层,激活函数是relu。
还怕过拟合,再来个Dropout层!
model.add(Dense(128, activation='relu'))
model.add(Dropout(0.5))
最后通过一个softmax激活函数的全连接网络输出:
model.add(Dense(num_classes, activation='softmax'))
下面是编译这个模型,损失函数是categorical_crossentropy多类对数损失函数,优化器选用Adadelta,我们在《Tensorflow快餐教程(7) - 梯度下降》中有过介绍。
model.compile(loss=keras.losses.categorical_crossentropy,
optimizer=keras.optimizers.Adadelta(),
metrics=['accuracy'])
下面是可以运行的完整代码:
from __future__ import print_function
import keras
from keras.datasets import mnist
from keras.models import Sequential
from keras.layers import Dense, Dropout, Flatten
from keras.layers import Conv2D, MaxPooling2D
from keras import backend as K
batch_size = 128
num_classes = 10
epochs = 12
# input image dimensions
img_rows, img_cols = 28, 28
# the data, split between train and test sets
(x_train, y_train), (x_test, y_test) = mnist.load_data()
if K.image_data_format() == 'channels_first':
x_train = x_train.reshape(x_train.shape[0], 1, img_rows, img_cols)
x_test = x_test.reshape(x_test.shape[0], 1, img_rows, img_cols)
input_shape = (1, img_rows, img_cols)
else:
x_train = x_train.reshape(x_train.shape[0], img_rows, img_cols, 1)
x_test = x_test.reshape(x_test.shape[0], img_rows, img_cols, 1)
input_shape = (img_rows, img_cols, 1)
x_train = x_train.astype('float32')
x_test = x_test.astype('float32')
x_train /= 255
x_test /= 255
print('x_train shape:', x_train.shape)
print(x_train.shape[0], 'train samples')
print(x_test.shape[0], 'test samples')
# convert class vectors to binary class matrices
y_train = keras.utils.to_categorical(y_train, num_classes)
y_test = keras.utils.to_categorical(y_test, num_classes)
model = Sequential()
model.add(Conv2D(32, kernel_size=(3, 3),
activation='relu',
input_shape=input_shape))
model.add(Conv2D(64, (3, 3), activation='relu'))
model.add(MaxPooling2D(pool_size=(2, 2)))
model.add(Dropout(0.25))
model.add(Flatten())
model.add(Dense(128, activation='relu'))
model.add(Dropout(0.5))
model.add(Dense(num_classes, activation='softmax'))
model.compile(loss=keras.losses.categorical_crossentropy,
optimizer=keras.optimizers.Adadelta(),
metrics=['accuracy'])
model.fit(x_train, y_train,
batch_size=batch_size,
epochs=epochs,
verbose=1,
validation_data=(x_test, y_test))
score = model.evaluate(x_test, y_test, verbose=0)
print('Test loss:', score[0])
print('Test accuracy:', score[1])
MNIST的例子实在用了太多遍了,我也有点不好意思了。下面我们来个surprise,处理一下各种语言之间的翻译!
机器翻译:多语种互译!
英译汉,汉译英之类的事情,在学生时代是不是一直难为这你呢?
现在不用担心了,只要有两种语言的对照表,我们就可以训练一个模型来像做一个机器翻译。
首先得下载一个字典:http://www.manythings.org/anki/
然后我们还是老办法,我们先看一下核心代码。没啥说的,这类序列化处理的问题用的一定是RNN,通常都是用LSTM.
下面就是用LSTM建模的过程:
encoder_inputs = Input(shape=(None, num_encoder_tokens))
encoder = LSTM(latent_dim, return_state=True)
encoder_outputs, state_h, state_c = encoder(encoder_inputs)
encoder_states = [state_h, state_c]
decoder_inputs = Input(shape=(None, num_decoder_tokens))
decoder_lstm = LSTM(latent_dim, return_sequences=True, return_state=True)
decoder_outputs, _, _ = decoder_lstm(decoder_inputs,
initial_state=encoder_states)
decoder_dense = Dense(num_decoder_tokens, activation='softmax')
decoder_outputs = decoder_dense(decoder_outputs)
model = Model([encoder_inputs, decoder_inputs], decoder_outputs)
优化器选用rmsprop,损失函数还是categorical_crossentropy.
validation_split是将一个集合随机分成训练集和测试集。
# Run training
model.compile(optimizer='rmsprop', loss='categorical_crossentropy')
model.fit([encoder_input_data, decoder_input_data], decoder_target_data,
batch_size=batch_size,
epochs=epochs,
validation_split=0.2)
最后,训练一个模型不容易,我们将其存储起来。
model.save('s2s.h5')
下面是完整的实现了机器翻译功能的代码,加上注释和空行其实也就不过100多行:
from __future__ import print_function
from keras.models import Model
from keras.layers import Input, LSTM, Dense
import numpy as np
batch_size = 64 # Batch size for training.
epochs = 100 # Number of epochs to train for.
latent_dim = 256 # Latent dimensionality of the encoding space.
num_samples = 10000 # Number of samples to train on.
# Path to the data txt file on disk.
data_path = 'fra-eng/fra.txt'
# Vectorize the data.
input_texts = []
target_texts = []
input_characters = set()
target_characters = set()
with open(data_path, 'r', encoding='utf-8') as f:
lines = f.read().split('\n')
for line in lines[: min(num_samples, len(lines) - 1)]:
input_text, target_text = line.split('\t')
# We use "tab" as the "start sequence" character
# for the targets, and "\n" as "end sequence" character.
target_text = '\t' + target_text + '\n'
input_texts.append(input_text)
target_texts.append(target_text)
for char in input_text:
if char not in input_characters:
input_characters.add(char)
for char in target_text:
if char not in target_characters:
target_characters.add(char)
input_characters = sorted(list(input_characters))
target_characters = sorted(list(target_characters))
num_encoder_tokens = len(input_characters)
num_decoder_tokens = len(target_characters)
max_encoder_seq_length = max([len(txt) for txt in input_texts])
max_decoder_seq_length = max([len(txt) for txt in target_texts])
print('Number of samples:', len(input_texts))
print('Number of unique input tokens:', num_encoder_tokens)
print('Number of unique output tokens:', num_decoder_tokens)
print('Max sequence length for inputs:', max_encoder_seq_length)
print('Max sequence length for outputs:', max_decoder_seq_length)
input_token_index = dict(
[(char, i) for i, char in enumerate(input_characters)])
target_token_index = dict(
[(char, i) for i, char in enumerate(target_characters)])
encoder_input_data = np.zeros(
(len(input_texts), max_encoder_seq_length, num_encoder_tokens),
dtype='float32')
decoder_input_data = np.zeros(
(len(input_texts), max_decoder_seq_length, num_decoder_tokens),
dtype='float32')
decoder_target_data = np.zeros(
(len(input_texts), max_decoder_seq_length, num_decoder_tokens),
dtype='float32')
for i, (input_text, target_text) in enumerate(zip(input_texts, target_texts)):
for t, char in enumerate(input_text):
encoder_input_data[i, t, input_token_index[char]] = 1.
for t, char in enumerate(target_text):
# decoder_target_data is ahead of decoder_input_data by one timestep
decoder_input_data[i, t, target_token_index[char]] = 1.
if t > 0:
# decoder_target_data will be ahead by one timestep
# and will not include the start character.
decoder_target_data[i, t - 1, target_token_index[char]] = 1.
# Define an input sequence and process it.
encoder_inputs = Input(shape=(None, num_encoder_tokens))
encoder = LSTM(latent_dim, return_state=True)
encoder_outputs, state_h, state_c = encoder(encoder_inputs)
# We discard `encoder_outputs` and only keep the states.
encoder_states = [state_h, state_c]
# Set up the decoder, using `encoder_states` as initial state.
decoder_inputs = Input(shape=(None, num_decoder_tokens))
# We set up our decoder to return full output sequences,
# and to return internal states as well. We don't use the
# return states in the training model, but we will use them in inference.
decoder_lstm = LSTM(latent_dim, return_sequences=True, return_state=True)
decoder_outputs, _, _ = decoder_lstm(decoder_inputs,
initial_state=encoder_states)
decoder_dense = Dense(num_decoder_tokens, activation='softmax')
decoder_outputs = decoder_dense(decoder_outputs)
# Define the model that will turn
# `encoder_input_data` & `decoder_input_data` into `decoder_target_data`
model = Model([encoder_inputs, decoder_inputs], decoder_outputs)
# Run training
model.compile(optimizer='rmsprop', loss='categorical_crossentropy')
model.fit([encoder_input_data, decoder_input_data], decoder_target_data,
batch_size=batch_size,
epochs=epochs,
validation_split=0.2)
# Save model
model.save('s2s.h5')
encoder_model = Model(encoder_inputs, encoder_states)
decoder_state_input_h = Input(shape=(latent_dim,))
decoder_state_input_c = Input(shape=(latent_dim,))
decoder_states_inputs = [decoder_state_input_h, decoder_state_input_c]
decoder_outputs, state_h, state_c = decoder_lstm(
decoder_inputs, initial_state=decoder_states_inputs)
decoder_states = [state_h, state_c]
decoder_outputs = decoder_dense(decoder_outputs)
decoder_model = Model(
[decoder_inputs] + decoder_states_inputs,
[decoder_outputs] + decoder_states)
# Reverse-lookup token index to decode sequences back to
# something readable.
reverse_input_char_index = dict(
(i, char) for char, i in input_token_index.items())
reverse_target_char_index = dict(
(i, char) for char, i in target_token_index.items())
def decode_sequence(input_seq):
# Encode the input as state vectors.
states_value = encoder_model.predict(input_seq)
# Generate empty target sequence of length 1.
target_seq = np.zeros((1, 1, num_decoder_tokens))
# Populate the first character of target sequence with the start character.
target_seq[0, 0, target_token_index['\t']] = 1.
# Sampling loop for a batch of sequences
# (to simplify, here we assume a batch of size 1).
stop_condition = False
decoded_sentence = ''
while not stop_condition:
output_tokens, h, c = decoder_model.predict(
[target_seq] + states_value)
# Sample a token
sampled_token_index = np.argmax(output_tokens[0, -1, :])
sampled_char = reverse_target_char_index[sampled_token_index]
decoded_sentence += sampled_char
# Exit condition: either hit max length
# or find stop character.
if (sampled_char == '\n' or
len(decoded_sentence) > max_decoder_seq_length):
stop_condition = True
# Update the target sequence (of length 1).
target_seq = np.zeros((1, 1, num_decoder_tokens))
target_seq[0, 0, sampled_token_index] = 1.
# Update states
states_value = [h, c]
return decoded_sentence
for seq_index in range(100):
# Take one sequence (part of the training set)
# for trying out decoding.
input_seq = encoder_input_data[seq_index: seq_index + 1]
decoded_sentence = decode_sequence(input_seq)
print('-')
print('Input sentence:', input_texts[seq_index])
print('Decoded sentence:', decoded_sentence)
翻译结果输出如下,是不是很好玩?
Input sentence: Let's go!
Decoded sentence: 我們開始吧!
-
Input sentence: Look out!
Decoded sentence: 当心!
-
Input sentence: She runs.
Decoded sentence: 她在行走。
-
Input sentence: Stand up.
Decoded sentence: 起立。
-
Input sentence: They won.
Decoded sentence: 他们赢了。
-
Input sentence: Tom died.
Decoded sentence: 汤姆去世了。
-
Input sentence: Tom quit.
Decoded sentence: 汤姆不干了。
-
Input sentence: Tom swam.
Decoded sentence: 汤姆游泳了。
-
Input sentence: Trust me.
Decoded sentence: 相信我。
-
Input sentence: Try hard.
Decoded sentence: 努力。
前情提要
Tensorflow快餐教程(1) - 30行代码搞定手写识别: https://blog.csdn.net/lusing/article/details/79965160
Tensorflow快餐教程(2) - 标量计算: https://blog.csdn.net/lusing/article/details/79980808
Tensorflow快餐教程(3) - 向量: https://blog.csdn.net/lusing/article/details/80054716
Tensorflow快餐教程(4) - 矩阵: https://blog.csdn.net/lusing/article/details/80071169
Tensorflow快餐教程(5) - 范数: https://blog.csdn.net/lusing/article/details/80082235
Tensorflow快餐教程(6) - 矩阵分解: https://blog.csdn.net/lusing/article/details/80113583
Tensorflow快餐教程(7) - 梯度下降: https://blog.csdn.net/lusing/article/details/80178069
Tensorflow快餐教程(8) - 深度学习简史:https://blog.csdn.net/lusing/article/details/80182063
Tensorflow快餐教程(9) - 卷积: https://blog.csdn.net/lusing/article/details/80199015
Tensorflow快餐教程(10) - 循环神经网络: https://blog.csdn.net/lusing/article/details/80246226
Tensorflow快餐教程(11) - 不懂机器学习就只调API行不行?: https://mp.csdn.net/mdeditor/80369607
Tensorflow快餐教程(12) - 用机器写莎士比亚的戏剧: https://blog.csdn.net/lusing/article/details/80502056
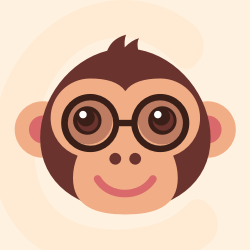



更多推荐
所有评论(0)