tensorflow通过提取Mfcc特征+卷积神经网络来实现语音分类

对于商业需求,还有很多需要改进的地方,大家多交流
准备工作
一、python+pycharm+tensorflow的下载与安装以及配置
忠告:不要下载tensorflow2.0以上的版本,因为tensorflow1.0版本的很多方法tensorflow2.0都不用了,而且网上有关的资料都是tensorflow1.版本,tensorflow2.0的资料很少,你报错的话,百度出来的博客都是让你改成低版本的;
4、下载pycharm软件,这个软件是python语言编写的软件,建议下载破解版本的
6、如何查看安装的tensorflow的版本
https://blog.csdn.net/qq_37591637/article/details/102782233
7、所有模块的安装都在这个里面进行
第一步、激活环境,只要第一次打开这个窗口就要输入activate tensorflow
第二步、做其他操作,由于大家刚开始都没有安装numpy等等模块,运行程序会报错,举个例子你就明白了
运行程序,报错,没有tensorflow_datasets这个模块,怎么办?我们需要安装
pip install tensorflow-datasets
注意:如果安装不成功,多半是因为与国外服务器连接信号差,连接不上,可以试试从国内下载,点解决方案
二、文件部分
足够多的.wav文件,如果.wav文件少的话。就会导致训练的模型太过于苛刻,那么测试其他文件的时候准确率就很低了;
网上很多资源下载都要6.9G,下载网速很慢;而且训练起来也要十几天的时间!
我的训练文件只有四类,每类里面100个文件;本次语音识别的文件在这里
https://download.csdn.net/download/qq_37591637/12106150
原理介绍
1、训练文件,一般是.wav文件,为什么是.wav文件,因为.wav文件占存储空间小,而且无损坏丢失;
2、无论是语音识别还是语音分类,想要训练精度高的模型,你要知道以下的名词是干什么用的,你不用推算原理!
mfcc特征、加窗、分帧、快速傅里叶变换,卷积层,全连接层,池化层,随机梯度下降算法
3、是不是觉得复杂,我先给你捋一下整个流程,然后我们一个个的来把复杂的原理简单深入了解一下;
3.1、读取大批的语音文件,获取文件的路径以及标签
作用:获取文件路径是肯定的,不然怎么处理文件;标签对应的是这个文件的内容,不然怎么知道我训练的模型准不准确呢?
3.2、对语音文件进行提取mfcc特征
作用:每个人的发音的声道形状是不变的,映射到语音文件上,是包络;怎么获取包络,就是提取Mfcc特征的过程,这个过程包括:预加重、分帧、加窗、快速傅里叶变换、逆快速傅里叶变换等等;
3.3、开始创建模型,卷积层,池化层,全连接层,激活层,以及损失函数;我们通过这些层以后,会得到关于这个音频文件的预测内容,然后与标签比较,由于大批量的文件不断的比较,如果误差大,模型会反向传输调整这些层的参数,直到准确率达到95%多。
3.4、模型训练好以后,可以指定保存位置;然后加载这个模型预测测试文件的内容!
逐步介绍每一个名词,每个名词都是一个篇幅,点进去查看
1、分帧
tensorflow语音识别之还原分帧的原理,让你拨开云雾见真相
2、加窗
3、快速傅里叶变换
tensorflow语音识别之史上最强教程让你快速理解什么是快速傅里叶变换
4、mfcc的来龙去脉
tensorflow语音识别之搞清楚梅尔频率倒谱系数(MFCC)的来龙去脉
5、卷积层
tensorflow入门之卷积层究竟是谁啥,卷积究竟做什么操作?
tensorflow入门之32个卷积核是不是1个同样的滤波器执行32次
6、池化层是干嘛的
tensorflow入门之池化层究竟干什么操作?有什么用
7、全连接层
tensorflow之博客史上最直白最简单教程 手把手帮你解刨并透彻理解全连接层与卷积层
8、什么是交叉滴
9、激励层作用
tensorflow入门之ReLU激励层有什么用处?
10、其他
tensorflow入门之tf.nn.dropout()函数的用法
代码部分
#!/usr/bin/python3
# -*- coding: utf-8 -*-
import tensorflow as tf
import scipy.io.wavfile as wav
from python_speech_features import mfcc,delta
import os
import numpy as np
import sklearn.preprocessing
path_film = os.path.abspath('.')
path = path_film + "/data/ddd/"
test_path = path_film + "/data/test_data/"
#使用one-hot编码,将离散特征的取值扩展到了欧式空间
#全局one-hot编码空间
label_binarizer = ""
def def_one_hot(x):
if label_binarizer == "":
binarizer = sklearn.preprocessing.LabelBinarizer()
else:
binarizer = label_binarizer
##这里有问题
y = binarizer.fit_transform(x)
return y
def read_wav_path(path):
map_path = []
labels = []
for x in os.listdir(path):
[map_path, labels] = circle(path, x, map_path, labels)
return map_path, labels
##循环遍历
def circle(path,x,map_path,labels):
if os.path.isfile(str(path) + str(x)):
map_path.append(str(path) + str(x))
else:
for y in os.listdir(str(path) + str(x)+"/"):
labels.append(x)
circle(str(path) + str(x)+"/",y,map_path,labels)
return map_path,labels
def def_wav_read_mfcc(file_name):
fs, audio = wav.read(file_name)
processed_audio = mfcc(audio, samplerate=fs)
return processed_audio
def find_matrix_max_shape(audio):
##这个里面的wav文件的长度不一,现在选出其中最大行列的h(高度),l(长度)
h, l = 0, 0
for a in audio:
a, b = np.array(a).shape
if a > h:
h=a
if b > l:
l=b
return h, l
def matrix_make_up(audio):
##找出wav文件中宽高最大的参数h,l
h, l = find_matrix_max_shape(audio)
##对于长度不够的,会填充0
new_audio = []
for aa in audio:
a, b = np.array(aa).shape
zeros_matrix = np.zeros([h, l],np.int8)
for i in range(a):
for j in range(b):
zeros_matrix[i, j]=zeros_matrix[i,j]+aa[i,j]
new_audio.append(zeros_matrix)
return new_audio,h,l
def read_wav_matrix(path):
##获取该路径下面的文件以及标签
map_path, labels = read_wav_path(path)
##添加文件夹的名称在里面
##提取mfcc的特征
audio = []
for idx, folder in enumerate(map_path):
processed_audio_delta = def_wav_read_mfcc(folder)
audio.append(processed_audio_delta)
##提取mfcc的特征
##统一长度的wav文件
x_data,h,l = matrix_make_up(audio)
##统一长度的wav文件
##音频文件数字化
x_data = np.array(x_data)
##标签变成热码
x_label = np.array(def_one_hot(labels))
return x_data, x_label, h, l
#初始化权值
def weight_variable(shape,name):
initial = tf.truncated_normal(shape,stddev=0.01)#生成一个截断的正态分布
return tf.Variable(initial,name=name)
#初始化偏置
def bias_variable(shape,name):
initial = tf.constant(0.01,shape=shape)
return tf.Variable(initial,name=name)
#卷积层
def conv2d(x,W):
return tf.nn.conv2d(x,W,strides=[1,1,1,1],padding='SAME')
#池化层
def max_pool_2x2(x):
return tf.nn.max_pool(x,ksize=[1,2,2,1],strides=[1,2,2,1],padding='SAME')
def xunlianlo(path):
x_train, y_train, h, l = read_wav_matrix(path)
m,n = y_train.shape
# 定义两个placeholder
x = tf.placeholder(tf.float32, [None, h, l], name='x-input')
y = tf.placeholder(tf.float32, [None, n], name='y-input')
# 改变x的格式转为4D的向量[batch, in_height, in_width, in_channels]`
x_image = tf.reshape(x, [-1, h, l, 1], name='x_image')
# 初始化第一个卷积层的权值和偏置
W_conv1 = weight_variable([5, 5, 1, 32], name='W_conv1') # 5*5的采样窗口,32个卷积核从3个平面抽取特征
b_conv1 = bias_variable([32], name='b_conv1') # 每一个卷积核一个偏置值
# 把x_image和权值向量进行卷积,再加上偏置值,然后应用于relu激活函数
conv2d_1 = conv2d(x_image, W_conv1) + b_conv1
h_conv1 = tf.nn.leaky_relu(conv2d_1)
h_pool1 = max_pool_2x2(h_conv1) # 进行max-pooling
# 初始化第二个卷积层的权值和偏置
W_conv2 = weight_variable([5, 5, 32, 64], name='W_conv2') # 5*5的采样窗口,64个卷积核从32个平面抽取特征
b_conv2 = bias_variable([64], name='b_conv2') # 每一个卷积核一个偏置值
# 把h_pool1和权值向量进行卷积,再加上偏置值,然后应用于relu激活函数
conv2d_2 = conv2d(h_pool1, W_conv2) + b_conv2
h_conv2 = tf.nn.leaky_relu(conv2d_2)
h_pool2 = max_pool_2x2(h_conv2) # 进行max-pooling
# 初始化第一个全连接层的权值
W_fc1 = weight_variable([25 * 4 * 64, 10], name='W_fc1') # 上一场有75*75*64个神经元,全连接层有1024个神经元
b_fc1 = bias_variable([10], name='b_fc1') # 1024个节点
# 把池化层2的输出扁平化为1维
h_pool2_flat = tf.reshape(h_pool2, [-1, 25 * 4 * 64], name='h_pool2_flat')
# 求第一个全连接层的输出
wx_plus_b1 = tf.matmul(h_pool2_flat, W_fc1) + b_fc1
h_fc1 = tf.nn.leaky_relu(wx_plus_b1)
# keep_prob用来表示神经元的输出概率
keep_prob = tf.placeholder(tf.float32, name='keep_prob')
h_fc1_drop = tf.nn.dropout(h_fc1, keep_prob, name='h_fc1_drop')
print("..................................................")
# 初始化第二个全连接层
W_fc2 = weight_variable([10, n], name='W_fc2')
b_fc2 = bias_variable([n], name='b_fc2')
wx_plus_b2 = tf.matmul(h_fc1_drop, W_fc2) + b_fc2
# 计算输出
prediction = tf.nn.leaky_relu(wx_plus_b2)
tf.add_to_collection('predictions', prediction)
p = tf.nn.softmax(wx_plus_b2)
tf.add_to_collection('p', p)
# 交叉熵代价函数
cross_entropy = tf.reduce_mean(tf.nn.softmax_cross_entropy_with_logits(labels=y, logits=prediction),
name='cross_entropy')
# 使用AdamOptimizer进行优化
train_step = tf.train.AdamOptimizer(1e-5).minimize(cross_entropy)
# 求准确率
# 结果存放在一个布尔列表中
correct_prediction = tf.equal(tf.argmax(prediction, 1), tf.argmax(y, 1)) # argmax返回一维张量中最大的值所在的位置
# 求准确率
accuracy = tf.reduce_mean(tf.cast(correct_prediction, tf.float32))
#保存模型使用环境
saver = tf.train.Saver()
with tf.Session() as sess:
sess.run(tf.global_variables_initializer())
# 创建一个协调器,管理线程
coord = tf.train.Coordinator()
# 启动QueueRunner, 此时文件名队列已经进队
threads = tf.train.start_queue_runners(sess=sess, coord=coord)
for i in range(50000):
# 训练模型
sess.run(train_step, feed_dict={x: x_train, y: y_train, keep_prob: 1.0})
train_acc = sess.run(accuracy, feed_dict={x: x_train, y: y_train, keep_prob: 1.0})
print("训练第 " + str(i) + " 次, 训练集准确率= " + str(train_acc))
if train_acc>=0.98:
print("模型训练成功了")
saver.save(sess, 'nn/my_net.ckpt')
break;
# 通知其他线程关闭
coord.request_stop()
# 其他所有线程关闭之后,这一函数才能返回
coord.join(threads)
def test_main(test_path):
# 本地情况下生成数据
x_test, y_test, h, l = read_wav_matrix(test_path)
m,n = y_test.shape
# 迭代网络
with tf.Session() as sess:
# 保存模型使用环境
saver = tf.train.import_meta_graph("nn/my_net.ckpt.meta")
saver.restore(sess, 'nn/my_net.ckpt')
predictions = tf.get_collection('predictions')[0]
p = tf.get_collection('p')[0]
graph = tf.get_default_graph()
input_x = graph.get_operation_by_name('x-input').outputs[0]
keep_prob = graph.get_operation_by_name('keep_prob').outputs[0]
for i in range(m):
result = sess.run(predictions, feed_dict={input_x: np.array([x_test[i]]),keep_prob:1.0})
haha = sess.run(p, feed_dict={input_x: np.array([x_test[i]]), keep_prob: 1.0})
print("实际 :"+str(np.argmax(y_test[i]))+" ,预测: "+str(np.argmax(result))+" ,预测可靠度: "+str(np.max(haha)))
if __name__ == '__main__':
xunlianlo(path)##训练模型的方法
##test_main(test_path)##测试的方法
训练模型
是xunlianlo(path)方法,注释掉test_main(test_path)方法
测试文件
是test_main(test_path)方法,注释掉xunlianlo(path)方法
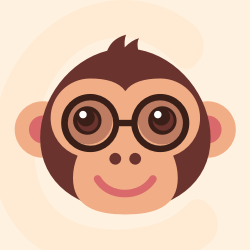



更多推荐
所有评论(0)