tensorflow 卷积:设定特定卷积核
tensorflow
一个面向所有人的开源机器学习框架
项目地址:https://gitcode.com/gh_mirrors/te/tensorflow

·
镜像问题:pytorch 自定义卷积核进行卷积--卷积核订制
有时想用tensorflow的conv2d的卷积操作,实现一些特定的滤波操作,如patch求和、计算梯度等,这时可以通过设计特定的卷积核来实现功能。
先看tf.nn.conv2d的各个参数:
tf.nn.conv2d (input, filter, strides, padding, use_cudnn_on_gpu=None, data_format=None, name=None)
参数:
input : tf张量,shape=[N,H,W,C] ,dtype=tf.float32(最好)
filter: 卷积核,tf张量,dtype=tf.float32(与input保持一致),
shape=[filter_height,filter_weight,input_channel=C,output_channels ],
其中 filter_height 为卷积核高度,
filter_weight 为卷积核宽度,
in_channel 是图像通道数 ,和 input 的 in_channel 要保持一致,
out_channel 是卷积核的个数,代表输出的feature_map的通道数。
strides: 卷积时在图像每一维的步长,这是一个一维的向量,[ 1, strides, strides, 1],
第一位和最后一位固定必须是1,最后一个维度步长必须是1,是因为卷积是逐个通道进行的。
padding: string类型,值为“SAME” 和 “VALID”,表示的是卷积的形式,是否考虑边界。
"SAME"是考虑边界,不足的时候用0去填充周围,
"VALID"则不考虑
use_cudnn_on_gpu: bool类型,是否使用cudnn加速,默认为true
主要需要注意的是:input,filter,strides的shape及各维度的含义。尤其filter各维度的含义与input是不同的。stride是4个维度。
示例:
"""
脚本功能:将256*256的图像不重叠的划分为64*64的patch,计算每个patch中的像素值之和
实现:设计64*64的全1卷积核,以stride=64移动,不添加0 padding
"""
feature_map = tf.ones([1,256,256,1],dtype=tf.float32)
filter = tf.ones((64,64,1,1),dtype=tf.float32)
padding = "VALID"
result = tf.nn.conv2d(feature_map, filter, strides=(1,64,64,1), padding=padding)
print(result.shape)
with tf.Session() as sess:
print(sess.run(result))
返回结果:
(1, 4, 4, 1)
[[[[4096.]
[4096.]
[4096.]
[4096.]]
[[4096.]
[4096.]
[4096.]
[4096.]]
[[4096.]
[4096.]
[4096.]
[4096.]]
[[4096.]
[4096.]
[4096.]
[4096.]]]]
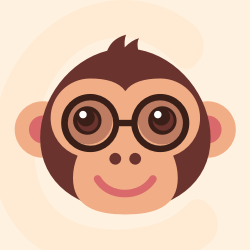



一个面向所有人的开源机器学习框架
最近提交(Master分支:2 个月前 )
a49e66f2
PiperOrigin-RevId: 663726708
2 个月前
91dac11a
This test overrides disabled_backends, dropping the default
value in the process.
PiperOrigin-RevId: 663711155
2 个月前
更多推荐
所有评论(0)