Tensorflow在训练好的模型上进行测试
tensorflow
一个面向所有人的开源机器学习框架
项目地址:https://gitcode.com/gh_mirrors/te/tensorflow

·
Tensorflow可以使用训练好的模型对新的数据进行测试,有两种方法:第一种方法是调用模型和训练在同一个py文件中,中情况比较简单;第二种是训练过程和调用模型过程分别在两个py文件中。本文将讲解第二种方法。
模型的保存
tensorflow提供可保存训练模型的接口,使用起来也不是很难,直接上代码讲解:
#网络结构
w1 = tf.Variable(tf.truncated_normal([in_units, h1_units], stddev=0.1))
b1 = tf.Variable(tf.zeros([h1_units]))
y = tf.nn.softmax(tf.matmul(w1, x) + b1)
tf.add_to_collection('network-output', y)
x = tf.placeholder(tf.float32, [None, in_units], name='x')
y_ = tf.placeholder(tf.float32, [None, 10], name='y_')
#损失函数与优化函数
cross_entropy = tf.reduce_mean(-tf.reduce_sum(y_ * tf.log(y), reduction_indices=[1]))
train_step = tf.train.AdamOptimizer(rate).minimize(cross_entropy)
saver = tf.train.Saver()
with tf.Session() as sess:
sess.run(init)
saver.save(sess,"save/model.ckpt")
train_step.run({x: train_x, y_: train_y})
以上代码就完成了模型的保存,值得注意的是下面这行代码
tf.add_to_collection('network-output', y)
这行代码保存了神经网络的输出,这个在后面使用导入模型过程中起到关键作用。
模型的导入
模型训练并保存后就可以导入来评估模型在测试集上的表现,网上很多文章只用简单的四则运算来做例子,让人看的头大。还是先上代码:
with tf.Session() as sess:
saver = tf.train.import_meta_graph('./model.ckpt.meta')
saver.restore(sess, './model.ckpt')# .data文件
pred = tf.get_collection('network-output')[0]
graph = tf.get_default_graph()
x = graph.get_operation_by_name('x').outputs[0]
y_ = graph.get_operation_by_name('y_').outputs[0]
y = sess.run(pred, feed_dict={x: test_x, y_: test_y})
讲解一下关键的代码,首先是pred = tf.get_collection('pred_network')[0]
,这行代码获得训练过程中网络输出的“接口”,简单理解就是,通过tf.get_collection()
这个方法获取了整个网络结构。获得网络结构后我们就需要喂它对应的数据y = sess.run(pred, feed_dict={x: test_x, y_: test_y})
在训练过程中我们的输入是
x = tf.placeholder(tf.float32, [None, in_units], name='x')
y_ = tf.placeholder(tf.float32, [None, 10], name='y_')
因此导入模型后所需的输入也要与之对应可使用以下代码获得:
x = graph.get_operation_by_name('x').outputs[0]
y_ = graph.get_operation_by_name('y_').outputs[0]
使用模型的最后一步就是输入测试集,然后按照训练好的网络进行评估
sess.run(pred, feed_dict={x: test_x, y_: test_y})
理解下这行代码,sess.run()
的函数原型为
run(fetches, feed_dict=None, options=None, run_metadata=None)
Tensorflow对 feed_dict 执行fetches操作,因此在导入模型后的运算就是,按照训练的网络计算测试输入的数据。
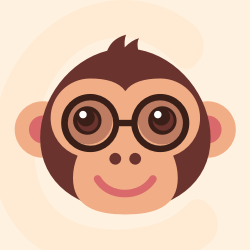



一个面向所有人的开源机器学习框架
最近提交(Master分支:2 个月前 )
a49e66f2
PiperOrigin-RevId: 663726708
2 个月前
91dac11a
This test overrides disabled_backends, dropping the default
value in the process.
PiperOrigin-RevId: 663711155
2 个月前
更多推荐
所有评论(0)