TensorFlow的变量管理:变量作用域与重用机制(variable_scope)

构建复杂深度学习模型时,出现大量重复结构与变量名的情况十分常见。为便于对这些变量进行管理,TensorFlow 给出的解决方案是变量的作用域与重用机制。简单来说,在不同的变量作用域下,相同的变量名是可以接受的,且他们之间并不会相互影响;在同一个变量作用域下,如果要接受相同的变量名,则要求设定重用规则。
TensorFlow的变量作用域与重用机制依赖于以下两个方法:
tf.get_variable(
name, shape=None, dtype=None, initializer=None, regularizer=None,
trainable=None, collections=None, caching_device=None, partitioner=None,
validate_shape=True, use_resource=None, custom_getter=None, constraint=None,
synchronization=tf.VariableSynchronization.AUTO,
aggregation=tf.compat.v1.VariableAggregation.NONE
)
建立或返回一个给定名称的变量
tf.variable_scope(
name_or_scope, default_name=None, values=None, initializer=None,
regularizer=None, caching_device=None, partitioner=None, custom_getter=None,
reuse=None, dtype=None, use_resource=None, constraint=None,
auxiliary_name_scope=True
)
管理传递给tf.get_variable()的变量名组成的命名空间
使用tf.get_variable()新建的变量与tf.Variable()新建的变量本身并无太大区别,tf.Variable也同样受variable_scope的影响。但tf.get_variable()还会搜索是否有同名的变量,以检查可能的冲突,tf.Variable()则不会管那么多。此外,结合命名空间(name_scope)来看,tf.Variable()创建变量的名字会受到name_scope的影响,而tf.get_variable()则不会。即:
with tf.name_scope("foo"):
# tf.Variable函数生成变量时受tf.name_scope影响,所以变量名为"foo/a:0"
a = tf.Variable([1], name='a')
print(a.name)
# foo/a: 0
# tf.get_variable函数不受tf.name_scope函数的影响,所以变量名为"b:0"
b = tf.get_variable("b", [1])
print(b.name)
# b:0
这里所说的name_scope,与variable_scope较为相似,但是name_scope不会对变量的作用域造成实际的改变,而只是加了一个范围,使得使用TensorBoard进行计算图可视化时更加直观。最常用的一个用法是,划分"input"、"loss_function"、"train_step"等name_scope,以便在可视化时清楚地看到整个pipeline中的各个步骤。
tf.variable_scope(scope_name)会管理在名为scope_name的域(scope)下传递给tf.get_variable的所有变量名,根据规则确定这些变量是否进行复用。
举一例用法如下:
with tf.variable_scope("foo"):
with tf.variable_scope("bar"):
v = tf.get_variable("v", [1])
assert v.name == "foo/bar/v:0"
这边新建了两层的变量作用域,分别是"foo"和"bar",在其下使用tf.get_variable定义了一个变量v。
tf.variable_scope()最重要的参数是reuse,有None,tf.AUTO_REUSE与True三个选项。此外,TensorFlow也支持捕获某一已经定义的变量作用域,并重新设置进行复用。
- reuse的默认选项是None,此时会继承父scope的reuse标志。
- 自动复用(设置reuse为tf.AUTO_REUSE),如果变量存在则复用,不存在则创建。这是最安全的用法,在使用新推出的EagerMode时reuse将被强制为tf.AUTO_REUSE选项。用法如下:
def foo(): with tf.variable_scope("foo", reuse=tf.AUTO_REUSE): v = tf.get_variable("v", [1]) return v v1 = foo() # Creates v. v2 = foo() # Gets the same, existing v. assert v1 == v2
- 复用(设置reuse=True):
with tf.variable_scope("foo"): v = tf.get_variable("v", [1]) with tf.variable_scope("foo", reuse=True): v1 = tf.get_variable("v", [1]) assert v1 == v
- 捕获某一域并设置复用(scope.reuse_variables()):
with tf.variable_scope("foo") as scope: v = tf.get_variable("v", [1]) scope.reuse_variables() v1 = tf.get_variable("v", [1]) assert v1 == v
当存在以下情况时,TensorFlow会抛出错误:
1)非复用的scope下再次定义已存在的变量;或
2)定义了复用但无法找到已定义的变量:
with tf.variable_scope("foo"):
v = tf.get_variable("v", [1])
v1 = tf.get_variable("v", [1])
# Raises ValueError("... v already exists ...").
with tf.variable_scope("foo", reuse=True):
v = tf.get_variable("v", [1])
# Raises ValueError("... v does not exists ...").
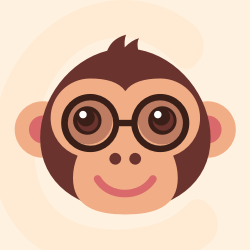



更多推荐
所有评论(0)