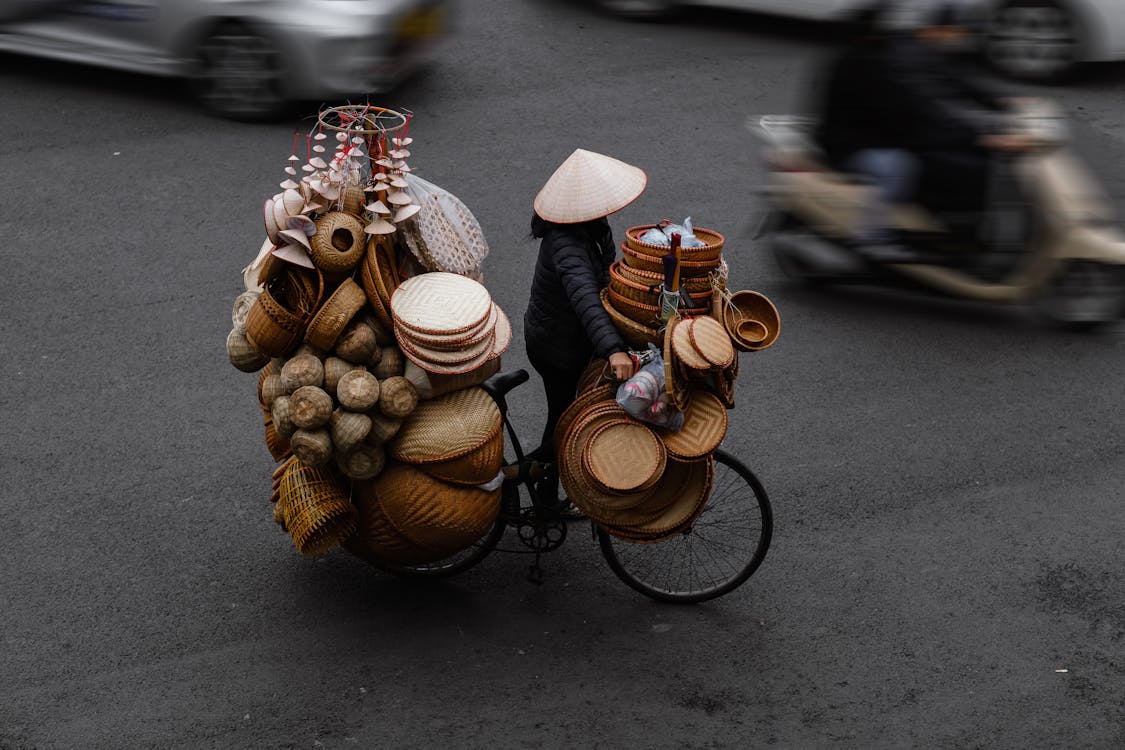
利用SHAP算法解释BERT模型的输出
bert
TensorFlow code and pre-trained models for BERT
项目地址:https://gitcode.com/gh_mirrors/be/bert

·
1 何为SHAP?
传统的 feature importance 只告诉哪个特征重要,但并不清楚该特征如何影响预测结果。SHAP 算法的最大优势是能反应每一个样本中特征的影响力,且可表现出影响的正负性。SHAP算法的主要思想为:控制变量法,如果某个特征出现或不出现,直接影响分类结果,那么该特征一定是比较重要的。因此,可以通过计算该特征出现或不出现的各种情况,来计算其对于分类结果的贡献度。在 SHAP 算法中用沙普利(Shapley )值表示不同特征对于预测结果的贡献度。Shapley 值是博弈论中使用的一种方法,它涉及公平地将收益和成本分配给在联盟中工作的行动者,由于每个行动者对联盟的贡献是不同的,Shapley 值保证每个行动者根据贡献的多少获得公平的份额。
2 代码实现
接下来展示如何用 SHAP 来解释基于 BERT 的文本分类任务,直接从 SHAP官网上扒下来代码:
import nlp
import numpy as np
import scipy as sp
import torch
import transformers
from transformers import BertTokenizer, BertForSequenceClassification
import shap
# load a BERT sentiment analysis model
tokenizer = transformers.DistilBertTokenizerFast.from_pretrained(
"distilbert-base-uncased"
)
model = transformers.DistilBertForSequenceClassification.from_pretrained(
"distilbert-base-uncased-finetuned-sst-2-english"
).cuda()
# define a prediction function
def f(x):
tv = torch.tensor(
[
tokenizer.encode(v, padding="max_length", max_length=512, truncation=True) for v in x
]
).cuda()
outputs = model(tv)
outputs = outputs[0].detach().cpu().numpy()
scores = (np.exp(outputs).T / np.exp(outputs).sum(-1)).T
val = sp.special.logit(scores[:, 1]) # use one vs rest logit units
return val
# build an explainer using a token masker
explainer = shap.Explainer(f, tokenizer)
# explain the model's predictions on IMDB reviews
imdb_train = nlp.load_dataset("imdb")["train"]
shap_values = explainer(imdb_train[:10], fixed_context=1, batch_size=2)
执行下列代码,用于展示数据集中第3个样本中不同特征对于预测结果的贡献度/值:
#plot the first sentence's explanation
shap.plots.text(shap_values[3])
shap.plots.bar(shap_values.abs.sum(0))
运行得到下列输出:
shap.plots.bar(shap_values.abs.max(0))
3 参考:
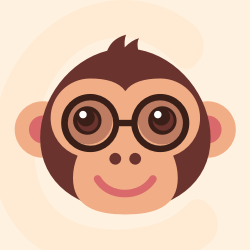



TensorFlow code and pre-trained models for BERT
最近提交(Master分支:24 天前 )
eedf5716
Add links to 24 smaller BERT models. 4 年前
8028c045 - 4 年前
更多推荐
所有评论(0)