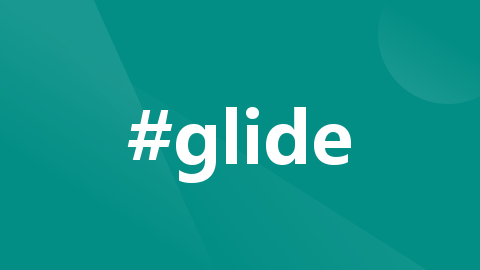
[GLIDE] Towards Photorealistic Image Generation and Editing with Text-Guided Diffusion Models

1、目的
综合所有的text prompts来生成photorealistic images
2、方法
Guided Language to Image Diffusion for Generation and Editing (GLIDE)
1)模型
-> 3.5B参数text-conditional diffusion model,64 x 64 resolution
-> 1.5B参数text-conditional upsampling diffusion model,256 x 256 resolution
-> noised 64 x 64 ViT-L CLIP model
2)text condition
-> Classifier-free guidance
将text编码为K tokens送入Transformer模型:作为class embedding;token embeddings的最后一层K个feature vectors映射后并联到扩散模型的每个attention层
优于CLIP guidance
-> CLIP Guidance
将classifier guidance中的梯度替换为image和caption的encoding的点积对图像的导数,即
需要在noised图像上训练CLIP,得到noised CLIP models
3)对模型进行fine-tune,来执行image inpainting
-> 增加4个通道:随机擦除图像区域后的RGB通道 + mask
-> 新通道参数初始化为0
-> 对于upsampling模型,gt仅包括高分辨率unmasked region
-> 可以让使用者迭代生成结果,直到和复杂的prompts匹配
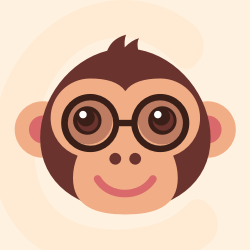



更多推荐
所有评论(0)