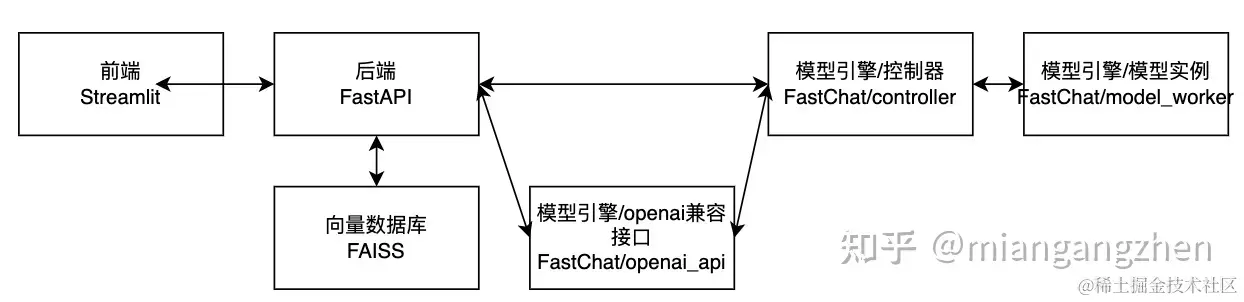
Langchain-chatchat: Langchain源码debug
Langchain-Chatchat
Langchain-Chatchat(原Langchain-ChatGLM)基于 Langchain 与 ChatGLM 等语言模型的本地知识库问答 | Langchain-Chatchat (formerly langchain-ChatGLM), local knowledge based LLM (like ChatGLM) QA app with langchain
项目地址:https://gitcode.com/gh_mirrors/la/Langchain-Chatchat

·
一、代码结构
- configs/ 配置文件路径
- server/ api服务、大模型服务等服务程序等核心代码
- webui_pages/ webui服务
- startup.py 启动脚本
│ .gitignore
│ CONTRIBUTING.md
│ init_database.py 用于初始化知识库
│ LICENSE
│ README.md
│ release.py
│ requirements.txt
│ requirements_api.txt
│ requirements_webui.txt
│ shutdown_all.sh 一键停止脚本,kill掉启动的服务
│ startup.py 一键启动
│ webui.py ui界面启动
├─chains
│ llmchain_with_history.py
│
├─common
│ __init__.py
│
├─configs
│ model_config.py.example 模型配置文件,配置使用的LLM和Emebdding模型;
│ server_config.py.example
│ __init__.py
├─embeddings
│ __init__.py
├─knowledge_base
│ └─samples 知识库
│ ├─content
│ │ test.txt 知识库上传的文档
│ └─vector_store 向量化后的知识
│ index.faiss
│ index.pkl
│
├─nltk_data Natural Language Toolkit (NLTK)是一个广泛使用的Python自然语言处理工具库
├─server
│ │ api.py 用于启动API服务
│ │ api_allinone_stale.py
│ │ llm_api.py 用于启动LLM
│ │ llm_api_shutdown.py
│ │ llm_api_stale.py
│ │ utils.py
│ │ webui_allinone_stale.py
│ │
│ ├─chat
│ │ chat.py 用于与LLM模型对话
│ │ knowledge_base_chat.py 用于与知识库对话
│ │ openai_chat.py
│ │ search_engine_chat.py 用于搜索引擎对话
│ │ utils.py
│ │ __init__.py
│ │
│ ├─db 知识库的数据库
│ │ │ base.py
│ │ │ session.py
│ │ │ __init__.py
│ │ │
│ │ ├─models
│ │ │ base.py 数据库表的基础属性
│ │ │ knowledge_base_model.py 知识库模型的表字段
│ │ │ knowledge_file_model.py 知识库文件的表字段
│ │ │ __init__.py
│ │ │
│ │ └─repository
│ │ knowledge_base_repository.py
│ │ knowledge_file_repository.py
│ │ __init__.py
│ │
│ ├─knowledge_base
│ │ │ kb_api.py 知识库API,创建、删除知识库;
│ │ │ kb_doc_api.py 知识库文件API,搜索、删除、更新、上传文档,重建向量库;
│ │ │ migrate.py 初始化 or 迁移重建知识库;
│ │ │ utils.py 提供了加载Embedding、获取文件加载器、文件转text的函数,可设置文本分割器;
│ │ │ __init__.py
│ │ │
│ │ └─kb_service
│ │ base.py 向量库的抽象类
│ │ default_kb_service.py
│ │ faiss_kb_service.py faiss向量库子类
│ │ milvus_kb_service.py
│ │ pg_kb_service.py
│ │ __init__.py
│ │
│ └─static
│
├─tests
│ └─api
│ test_kb_api.py 测试知识库API
│ test_stream_chat_api.py 测试对话API
│
├─text_splitter 各种文本分割器
│ ali_text_splitter.py 达摩院的文档分割
│ chinese_text_splitter.py 中文文本分割
│ zh_title_enhance.py 中文标题增强:判断是否是标题,然后在下一段文字的开头加入提示语与标题建立关联。
│ __init__.py
│
└─webui_pages UI界面构建
│ utils.py 简化api调用
│ __init__.py
│
├─dialogue
│ dialogue.py 问答功能,LLM问答和知识库问答,还有搜索引擎问答;
│ __init__.py
│
├─knowledge_base
│ knowledge_base.py 知识库管理界面构建
│ __init__.py
│
└─model_config
model_config.py 模型配置页面,TODO。应该是可以在界面上手动选择采用哪个LLM和Embedding模型。
__init__.py
二、页面对话框调用链路
页面输入问题是,调用的接口为:
app.post("/chat/chat",
tags=["Chat"],
summary="与llm模型对话(通过LLMChain)",
)(chat)
对应的chat 方法是:
async def chat(query: str = Body(..., description="用户输入", examples=["恼羞成怒"]),
conversation_id: str = Body("", description="对话框ID"),
history_len: int = Body(-1, description="从数据库中取历史消息的数量"),
history: Union[int, List[History]] = Body([],
description="历史对话,设为一个整数可以从数据库中读取历史消息",
examples=[[
{"role": "user",
"content": "我们来玩成语接龙,我先来,生龙活虎"},
{"role": "assistant", "content": "虎头虎脑"}]]
),
stream: bool = Body(False, description="流式输出"),
model_name: str = Body(LLM_MODELS[0], description="LLM 模型名称。"),
temperature: float = Body(TEMPERATURE, description="LLM 采样温度", ge=0.0, le=1.0),
max_tokens: Optional[int] = Body(None, description="限制LLM生成Token数量,默认None代表模型最大值"),
# top_p: float = Body(TOP_P, description="LLM 核采样。勿与temperature同时设置", gt=0.0, lt=1.0),
prompt_name: str = Body("default", description="使用的prompt模板名称(在configs/prompt_config.py中配置)"),
):
async def chat_iterator() -> AsyncIterable[str]:
nonlocal history, max_tokens
callback = AsyncIteratorCallbackHandler()
callbacks = [callback]
memory = None
if conversation_id:
message_id = add_message_to_db(chat_type="llm_chat", query=query, conversation_id=conversation_id)
# 负责保存llm response到message db
conversation_callback = ConversationCallbackHandler(conversation_id=conversation_id, message_id=message_id,
chat_type="llm_chat",
query=query)
callbacks.append(conversation_callback)
if isinstance(max_tokens, int) and max_tokens <= 0:
max_tokens = None
model = get_ChatOpenAI(
model_name=model_name,
temperature=temperature,
max_tokens=max_tokens,
callbacks=callbacks,
)
if history: # 优先使用前端传入的历史消息
history = [History.from_data(h) for h in history]
prompt_template = get_prompt_template("llm_chat", prompt_name)
input_msg = History(role="user", content=prompt_template).to_msg_template(False)
chat_prompt = ChatPromptTemplate.from_messages(
[i.to_msg_template() for i in history] + [input_msg])
elif conversation_id and history_len > 0: # 前端要求从数据库取历史消息
# 使用memory 时必须 prompt 必须含有memory.memory_key 对应的变量
prompt = get_prompt_template("llm_chat", "with_history")
chat_prompt = PromptTemplate.from_template(prompt)
# 根据conversation_id 获取message 列表进而拼凑 memory
memory = ConversationBufferDBMemory(conversation_id=conversation_id,
llm=model,
message_limit=history_len)
else:
prompt_template = get_prompt_template("llm_chat", prompt_name)
input_msg = History(role="user", content=prompt_template).to_msg_template(False)
chat_prompt = ChatPromptTemplate.from_messages([input_msg])
chain = LLMChain(prompt=chat_prompt, llm=model, memory=memory)
# Begin a task that runs in the background.
task = asyncio.create_task(wrap_done(
chain.acall({"input": query}),
callback.done),
)
if stream:
async for token in callback.aiter():
# Use server-sent-events to stream the response
yield json.dumps(
{"text": token, "message_id": message_id},
ensure_ascii=False)
else:
answer = ""
async for token in callback.aiter():
answer += token
yield json.dumps(
{"text": answer, "message_id": message_id},
ensure_ascii=False)
await task
return StreamingResponse(chat_iterator(), media_type="text/event-stream")
调用链路是: 1.chat接口 2.chat_iterator() 3.StreamingResponse
使用postman模拟用户页面问答的过程:
chat方法中调用了add_message_to_db方法:
@with_session
def add_message_to_db(session, conversation_id: str, chat_type, query, response="", message_id=None,
metadata: Dict = {}):
"""
新增聊天记录
"""
if not message_id:
message_id = uuid.uuid4().hex
m = MessageModel(id=message_id, chat_type=chat_type, query=query, response=response,
conversation_id=conversation_id,
meta_data=metadata)
session.add(m)
session.commit()
return m.id
kb_config.py 数据库保存配置如下:
# 知识库默认存储路径
KB_ROOT_PATH = os.path.join(os.path.dirname(os.path.dirname(__file__)), "knowledge_base")
if not os.path.exists(KB_ROOT_PATH):
os.mkdir(KB_ROOT_PATH)
# 数据库默认存储路径。
# 如果使用sqlite,可以直接修改DB_ROOT_PATH;如果使用其它数据库,请直接修改SQLALCHEMY_DATABASE_URI。
DB_ROOT_PATH = os.path.join(KB_ROOT_PATH, "info.db")
SQLALCHEMY_DATABASE_URI = f"sqlite:///{DB_ROOT_PATH}"
查看sqlite的数据表和结构:
from sqlalchemy import create_engine
from sqlalchemy.orm import sessionmaker
# 创建数据库连接
engine = create_engine('sqlite:///info.db')
# 创建Session类,用于与数据库进行交互
Session = sessionmaker(bind=engine)
# 创建Session实例
session = Session()
try:
# 开始事务
with session.begin():
# 执行SQL语句
tables = session.execute('SELECT name FROM sqlite_master WHERE type="table"')
# 打印所有表名
for table in tables:
print(table[0])
messages = session.execute("SELECT * FROM message limit 2")
# 处理查询结果
for row in messages:
print(row)
# 提交事务
session.commit()
except:
# 回滚事务
session.rollback()
raise
finally:
# 关闭连接
session.close()
##原文链接:https://blog.csdn.net/weixin_62650212/article/details/130212100
#四张表: conversation message knowledge_base knowledge_file file_doc summary_chunk
SQLite对应的实体类定义:server.db.models 目录下:
class MessageModel(Base):
"""
聊天记录模型
"""
__tablename__ = 'message'
id = Column(String(32), primary_key=True, comment='聊天记录ID')
conversation_id = Column(String(32), default=None, index=True, comment='对话框ID')
# chat/agent_chat等
chat_type = Column(String(50), comment='聊天类型')
query = Column(String(4096), comment='用户问题')
response = Column(String(4096), comment='模型回答')
# 记录知识库id等,以便后续扩展
meta_data = Column(JSON, default={})
# 满分100 越高表示评价越好
feedback_score = Column(Integer, default=-1, comment='用户评分')
feedback_reason = Column(String(255), default="", comment='用户评分理由')
create_time = Column(DateTime, default=func.now(), comment='创建时间')
def __repr__(self):
return f"<message(id='{self.id}', conversation_id='{self.conversation_id}', chat_type='{self.chat_type}', query='{self.query}', response='{self.response}',meta_data='{self.meta_data}',feedback_score='{self.feedback_score}',feedback_reason='{self.feedback_reason}', create_time='{self.create_time}')>"
如何系统的去学习大模型LLM ?
作为一名热心肠的互联网老兵,我意识到有很多经验和知识值得分享给大家,也可以通过我们的能力和经验解答大家在人工智能学习中的很多困惑,所以在工作繁忙的情况下还是坚持各种整理和分享。
但苦于知识传播途径有限,很多互联网行业朋友无法获得正确的资料得到学习提升,故此将并将重要的 AI大模型资料
包括AI大模型入门学习思维导图、精品AI大模型学习书籍手册、视频教程、实战学习等录播视频免费分享出来。
😝有需要的小伙伴,可以V扫描下方二维码免费领取🆓
一、全套AGI大模型学习路线
AI大模型时代的学习之旅:从基础到前沿,掌握人工智能的核心技能!
二、640套AI大模型报告合集
这套包含640份报告的合集,涵盖了AI大模型的理论研究、技术实现、行业应用等多个方面。无论您是科研人员、工程师,还是对AI大模型感兴趣的爱好者,这套报告合集都将为您提供宝贵的信息和启示。
三、AI大模型经典PDF籍
随着人工智能技术的飞速发展,AI大模型已经成为了当今科技领域的一大热点。这些大型预训练模型,如GPT-3、BERT、XLNet等,以其强大的语言理解和生成能力,正在改变我们对人工智能的认识。 那以下这些PDF籍就是非常不错的学习资源。
四、AI大模型商业化落地方案
阶段1:AI大模型时代的基础理解
- 目标:了解AI大模型的基本概念、发展历程和核心原理。
- 内容:
- L1.1 人工智能简述与大模型起源
- L1.2 大模型与通用人工智能
- L1.3 GPT模型的发展历程
- L1.4 模型工程
- L1.4.1 知识大模型
- L1.4.2 生产大模型
- L1.4.3 模型工程方法论
- L1.4.4 模型工程实践
- L1.5 GPT应用案例
阶段2:AI大模型API应用开发工程
- 目标:掌握AI大模型API的使用和开发,以及相关的编程技能。
- 内容:
- L2.1 API接口
- L2.1.1 OpenAI API接口
- L2.1.2 Python接口接入
- L2.1.3 BOT工具类框架
- L2.1.4 代码示例
- L2.2 Prompt框架
- L2.2.1 什么是Prompt
- L2.2.2 Prompt框架应用现状
- L2.2.3 基于GPTAS的Prompt框架
- L2.2.4 Prompt框架与Thought
- L2.2.5 Prompt框架与提示词
- L2.3 流水线工程
- L2.3.1 流水线工程的概念
- L2.3.2 流水线工程的优点
- L2.3.3 流水线工程的应用
- L2.4 总结与展望
阶段3:AI大模型应用架构实践
- 目标:深入理解AI大模型的应用架构,并能够进行私有化部署。
- 内容:
- L3.1 Agent模型框架
- L3.1.1 Agent模型框架的设计理念
- L3.1.2 Agent模型框架的核心组件
- L3.1.3 Agent模型框架的实现细节
- L3.2 MetaGPT
- L3.2.1 MetaGPT的基本概念
- L3.2.2 MetaGPT的工作原理
- L3.2.3 MetaGPT的应用场景
- L3.3 ChatGLM
- L3.3.1 ChatGLM的特点
- L3.3.2 ChatGLM的开发环境
- L3.3.3 ChatGLM的使用示例
- L3.4 LLAMA
- L3.4.1 LLAMA的特点
- L3.4.2 LLAMA的开发环境
- L3.4.3 LLAMA的使用示例
- L3.5 其他大模型介绍
阶段4:AI大模型私有化部署
- 目标:掌握多种AI大模型的私有化部署,包括多模态和特定领域模型。
- 内容:
- L4.1 模型私有化部署概述
- L4.2 模型私有化部署的关键技术
- L4.3 模型私有化部署的实施步骤
- L4.4 模型私有化部署的应用场景
学习计划:
- 阶段1:1-2个月,建立AI大模型的基础知识体系。
- 阶段2:2-3个月,专注于API应用开发能力的提升。
- 阶段3:3-4个月,深入实践AI大模型的应用架构和私有化部署。
- 阶段4:4-5个月,专注于高级模型的应用和部署。
这份完整版的大模型 LLM 学习资料已经上传CSDN,朋友们如果需要可以微信扫描下方CSDN官方认证二维码免费领取【保证100%免费
】
😝有需要的小伙伴,可以Vx扫描下方二维码免费领取🆓
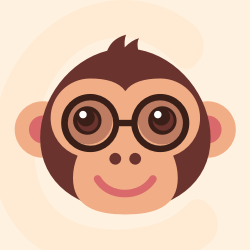



Langchain-Chatchat(原Langchain-ChatGLM)基于 Langchain 与 ChatGLM 等语言模型的本地知识库问答 | Langchain-Chatchat (formerly langchain-ChatGLM), local knowledge based LLM (like ChatGLM) QA app with langchain
最近提交(Master分支:4 个月前 )
86d4e825
6 个月前
10eb8e93
6 个月前
更多推荐
所有评论(0)