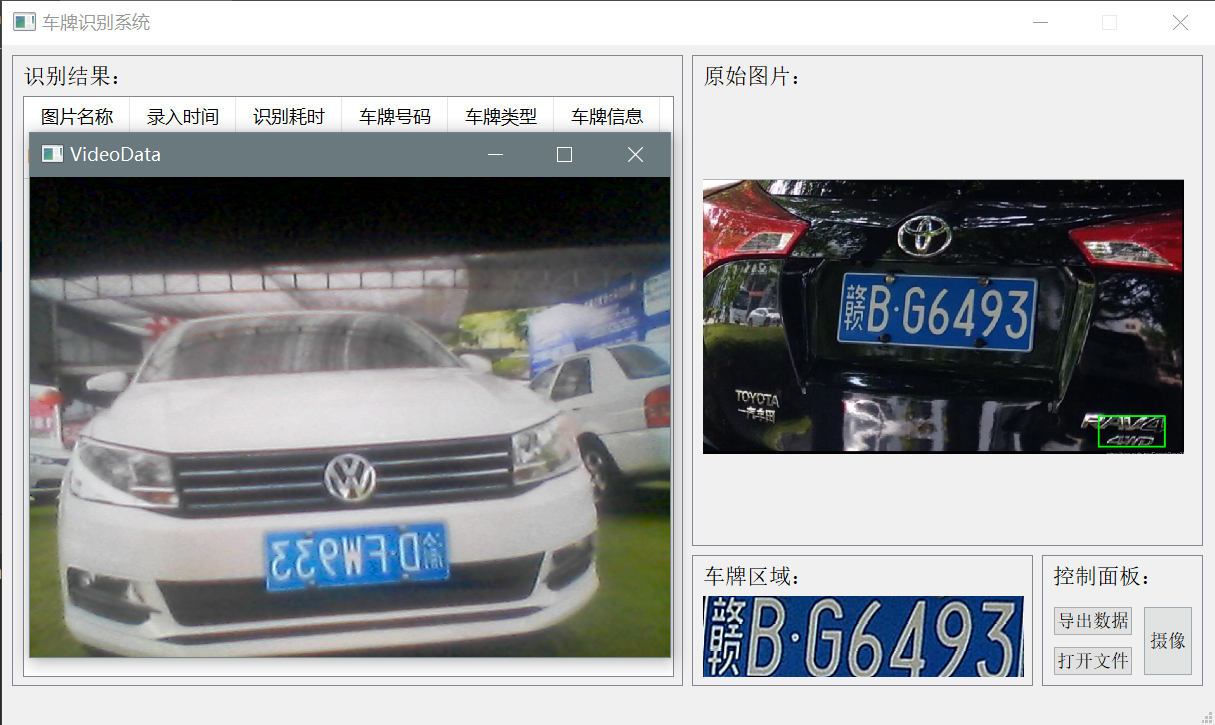
基于Python_opencv的车牌识别系统

基于python_opencv的车牌识别系统
一、说明
根据现有的车牌识别系统,本人对代码进行了优化,原有功能:
1、对图片中的车牌号进行识别,并对车牌所属地可视化
2、将识别出的车牌号、车牌所属地等信息导出Excel表格
3、根据QtDesinger设计GUI界面,将程序系统化
添加功能:调用摄像头实时识别捕捉到的车牌信息,并可视化
链接: 最新代码传送门
下图分别是调用摄像头和直接识别图像的画面:
二、具体实现流程
整个项目为模块化处理,按文件分为:
- Recognition.py(识别模块)
- UI_main(主函数及UI模块)
- SVM训练模块
- 路由配置模块
Recognition模块
此模块问本项目的核心,主要包含的功能有:
1、读取图像
使用cv2.imdecode()
函数将图片文件转换成流数据,赋值到内存缓存中,便于后续图像操作。使用cv2.resize()
函数对读取的图像进行缩放,以免图像过大导致识别耗时过长。
def __imreadex(self, filename):
return cv2.imdecode(np.fromfile(filename, dtype=np.uint8), cv2.IMREAD_COLOR)
def __point_limit(self, point):
if point[0] < 0:
point[0] = 0
if point[1] < 0:
point[1] = 0
2、图像预处理
def __preTreatment(self, car_pic):
if type(car_pic) == type("openc"):
img = self.__imreadex(car_pic)
else:
img = car_pic
pic_hight, pic_width = img.shape[:2]
if pic_width > self.MAX_WIDTH:
resize_rate = self.MAX_WIDTH / pic_width
img = cv2.resize(img, (self.MAX_WIDTH, int(pic_hight * resize_rate)),
interpolation=cv2.INTER_AREA) # 图片分辨率调整
# cv2.imshow('Image', img)
3、利用投影法,根据设定的阈值和图片直方图,找出波峰,用于分隔字符,得到逐个字符图片
def __find_waves(self, threshold, histogram):
up_point = -1 # 上升点
is_peak = False
if histogram[0] > threshold:
up_point = 0
is_peak = True
wave_peaks = []
for i, x in enumerate(histogram):
if is_peak and x < threshold:
if i - up_point > 2:
is_peak = False
wave_peaks.append((up_point, i))
elif not is_peak and x >= threshold:
is_peak = True
up_point = i
if is_peak and up_point != -1 and i - up_point >
wave_peaks.append((up_point, i))
return wave_peaks
def __seperate_card(self, img, waves):
part_cards = []
for wave in waves:
part_cards.append(img[:, wave[0]:wave[1]])
return part_cards
4、高斯去噪
使用cv2.GaussianBlur()
进行高斯去噪。使cv2.morphologyEx()
函数进行开运算,再使用cv2.addWeighted()
函数将运算结果与原图像做一次融合,从而去掉孤立的小点,毛刺等噪声。
if blur > 0:
img = cv2.GaussianBlur(img, (blur, blur), 0)
oldimg = img
img = cv2.cvtColor(img, cv2.COLOR_BGR2GRAY)
kernel = np.ones((20, 20), np.uint8)
img_opening = cv2.morphologyEx(img, cv2.MORPH_OPEN, kernel) # 开运算
img_opening = cv2.addWeighted(img, 1, img_opening, -1, 0); # 与上一次开运算结果融合
5、排除不是车牌的矩形区域
car_contours = []
for cnt in contours:
# 框选 生成最小外接矩形 返回值(中心(x,y), (宽,高), 旋转角度)
rect = cv2.minAreaRect(cnt)
# print('宽高:',rect[1])
area_width, area_height = rect[1]
# 选择宽大于高的区域
if area_width < area_height:
area_width, area_height = area_height, area_width
wh_ratio = area_width / area_height
# print('宽高比:',wh_ratio)
# 要求矩形区域长宽比在2到5.5之间,2到5.5是车牌的长宽比,其余的矩形排除
if wh_ratio > 2 and wh_ratio < 5.5:
car_contours.append(rect)
# box = cv2.boxPoints(rect)
# box = np.int0(box)
# 框出所有可能的矩形
# oldimg = cv2.drawContours(img, [box], 0, (0, 0, 255), 2)
# cv2.imshow("Test",oldimg )
6、分割字符并识别车牌文字
使用cv2.threshold()
函数进行二值化处理,再使用cv2.Canny()
函数找到各区域边缘,使用cv2.morphologyEx()
和cv2.morphologyEx()
两个函数分别进行一次开运算(先腐蚀运算,再膨胀运算)和一个闭运算(先膨胀运算,再腐蚀运算),去掉较小区域,同时填平小孔,弥合小裂缝。将车牌位置凸显出来
def __identification(self, card_imgs, colors,model,modelchinese):
# 识别车牌中的字符
result = {}
predict_result = []
roi = None
card_color = None
for i, color in enumerate(colors):
if color in ("blue", "yellow", "green"):
card_img = card_imgs[i]
# old_img = card_img
# 做一次锐化处理
kernel = np.array([[0, -1, 0], [-1, 5, -1], [0, -1, 0]], np.float32) # 锐化
card_img = cv2.filter2D(card_img, -1, kernel=kernel)
# cv2.imshow("custom_blur", card_img)
# RGB转GARY
gray_img = cv2.cvtColor(card_img, cv2.COLOR_BGR2GRAY)
# cv2.imshow('gray_img', gray_img)
# 黄、绿车牌字符比背景暗、与蓝车牌刚好相反,所以黄、绿车牌需要反向
if color == "green" or color == "yellow":
gray_img = cv2.bitwise_not(gray_img)
# 二值化
ret, gray_img = cv2.threshold(gray_img, 0, 255, cv2.THRESH_BINARY + cv2.THRESH_OTSU)
# cv2.imshow('gray_img', gray_img)
# 查找水平直方图波峰
x_histogram = np.sum(gray_img, axis=1)
# 最小值
x_min = np.min(x_histogram)
# 均值
x_average = np.sum(x_histogram) / x_histogram.shape[0]
x_threshold = (x_min + x_average) / 2
wave_peaks = self.__find_waves(x_threshold, x_histogram)
if len(wave_peaks) == 0:
continue
# 认为水平方向,最大的波峰为车牌区域
wave = max(wave_peaks, key=lambda x: x[1] - x[0])
gray_img = gray_img[wave[0]:wave[1]]
# cv2.imshow('gray_img', gray_img)
# 查找垂直直方图波峰
row_num, col_num = gray_img.shape[:2]
# 去掉车牌上下边缘1个像素,避免白边影响阈值判断
gray_img = gray_img[1:row_num - 1]
# cv2.imshow('gray_img', gray_img)
y_histogram = np.sum(gray_img, axis=0)
y_min = np.min(y_histogram)
y_average = np.sum(y_histogram) / y_histogram.shape[0]
y_threshold = (y_min + y_average) / 5 # U和0要求阈值偏小,否则U和0会被分成两半
wave_peaks = self.__find_waves(y_threshold, y_histogram)
# print(wave_peaks)
# for wave in wave_peaks:
# cv2.line(card_img, pt1=(wave[0], 5), pt2=(wave[1], 5), color=(0, 0, 255), thickness=2)
# 车牌字符数应大于6
if len(wave_peaks) <= 6:
# print(wave_peaks)
continue
wave = max(wave_peaks, key=lambda x: x[1] - x[0])
max_wave_dis = wave[1] - wave[0]
# 判断是否是左侧车牌边缘
if wave_peaks[0][1] - wave_peaks[0][0] < max_wave_dis / 3 and wave_peaks[0][0] == 0:
wave_peaks.pop(0)
# 组合分离汉字
cur_dis = 0
for i, wave in enumerate(wave_peaks):
if wave[1] - wave[0] + cur_dis > max_wave_dis * 0.6:
break
else:
cur_dis += wave[1] - wave[0]
if i > 0:
wave = (wave_peaks[0][0], wave_peaks[i][1])
wave_peaks = wave_peaks[i + 1:]
wave_peaks.insert(0, wave)
# 去除车牌上的分隔点
point = wave_peaks[2]
if point[1] - point[0] < max_wave_dis / 3:
point_img = gray_img[:, point[0]:point[1]]
if np.mean(point_img) < 255 / 5:
wave_peaks.pop(2)
if len(wave_peaks) <= 6:
# print("peak less 2:", wave_peaks)
continue
# print(wave_peaks)
# 分割牌照字符
part_cards = self.__seperate_card(gray_img, wave_peaks)
# 分割输出
#for i, part_card in enumerate(part_cards):
# cv2.imshow(str(i), part_card)
# 识别
for i, part_card in enumerate(part_cards):
# 可能是固定车牌的铆钉
if np.mean(part_card) < 255 / 5:
continue
part_card_old = part_card
w = abs(part_card.shape[1] - self.SZ) // 2
# 边缘填充
part_card = cv2.copyMakeBorder(part_card, 0, 0, w, w, cv2.BORDER_CONSTANT, value=[0, 0, 0])
# cv2.imshow('part_card', part_card)
# 图片缩放(缩小)
part_card = cv2.resize(part_card, (self.SZ, self.SZ), interpolation=cv2.INTER_AREA)
# cv2.imshow('part_card', part_card)
part_card = SVM_Train.preprocess_hog([part_card])
if i == 0: # 识别汉字
resp = self.modelchinese.predict(part_card) # 匹配样本
charactor = self.provinces[int(resp[0]) - self.PROVINCE_START]
# print(charactor)
else: # 识别字母
resp = self.model.predict(part_card) # 匹配样本
charactor = chr(resp[0])
# print(charactor)
# 判断最后一个数是否是车牌边缘,假设车牌边缘被认为是1
if charactor == "1" and i == len(part_cards) - 1:
if color == 'blue' and len(part_cards) > 7:
if part_card_old.shape[0] / part_card_old.shape[1] >= 7: # 1太细,认为是边缘
continue
elif color == 'blue' and len(part_cards) > 7:
if part_card_old.shape[0] / part_card_old.shape[1] >= 7: # 1太细,认为是边缘
continue
elif color == 'green' and len(part_cards) > 8:
if part_card_old.shape[0] / part_card_old.shape[1] >= 7: # 1太细,认为是边缘
continue
predict_result.append(charactor)
roi = card_img # old_img
card_color = color
break
return predict_result, roi, card_color # 识别到的字符、定位的车牌图像、车牌颜色
UI_main模块
此模块主要包含UI界面的设计的控件,图片识别的入口函数,摄像头识别入口函数,Excel表格生成函数:
1、UI界面主类
class Ui_MainWindow(object):
def __init__(self):
self.RowLength = 0
self.Data = [['文件名称', '录入时间', '车牌号码', '车牌类型', '识别耗时', '车牌信息']]
def setupUi(self, MainWindow):
MainWindow.setObjectName("MainWindow")
MainWindow.resize(1213, 680)
MainWindow.setFixedSize(1213, 680) # 设置窗体固定大小
MainWindow.setToolButtonStyle(QtCore.Qt.ToolButtonIconOnly)
self.centralwidget = QtWidgets.QWidget(MainWindow) #图片区域
self.centralwidget.setObjectName("centralwidget")
self.scrollArea = QtWidgets.QScrollArea(self.centralwidget)
self.scrollArea.setGeometry(QtCore.QRect(690, 10, 511, 491))
self.scrollArea.setWidgetResizable(False)
self.scrollArea.setObjectName("scrollArea")
self.scrollAreaWidgetContents = QtWidgets.QWidget()
self.scrollAreaWidgetContents.setGeometry(QtCore.QRect(10, 10, 509, 489))
self.scrollAreaWidgetContents.setObjectName("scrollAreaWidgetContents")
self.label_0 = QtWidgets.QLabel(self.scrollAreaWidgetContents)
self.label_0.setGeometry(QtCore.QRect(10, 10, 111, 20))
font = QtGui.QFont()
font.setPointSize(11)
self.label_0.setFont(font)
self.label_0.setObjectName("label_0")
self.label = QtWidgets.QLabel(self.scrollAreaWidgetContents)
self.label.setGeometry(QtCore.QRect(10, 40, 481, 441))
self.label.setObjectName("label")
self.label.setAlignment(Qt.AlignCenter)
self.scrollArea.setWidget(self.scrollAreaWidgetContents)
self.scrollArea_2 = QtWidgets.QScrollArea(self.centralwidget)
self.scrollArea_2.setGeometry(QtCore.QRect(10, 10, 671, 631))
self.scrollArea_2.setWidgetResizable(True)
self.scrollArea_2.setObjectName("scrollArea_2")
self.scrollAreaWidgetContents_1 = QtWidgets.QWidget()
self.scrollAreaWidgetContents_1.setGeometry(QtCore.QRect(0, 0, 669, 629))
self.scrollAreaWidgetContents_1.setObjectName("scrollAreaWidgetContents_1")
self.label_1 = QtWidgets.QLabel(self.scrollAreaWidgetContents_1)
self.label_1.setGeometry(QtCore.QRect(10, 10, 111, 20))
font = QtGui.QFont()
font.setPointSize(11)
self.label_1.setFont(font)
self.label_1.setObjectName("label_1")
self.tableWidget = QtWidgets.QTableWidget(self.scrollAreaWidgetContents_1) #设置布局
self.tableWidget.setGeometry(QtCore.QRect(10, 40, 651, 581)) # 581))
self.tableWidget.setObjectName("tableWidget")
self.tableWidget.setColumnCount(6)
self.tableWidget.setColumnWidth(0, 106) # 设置1列的宽度
self.tableWidget.setColumnWidth(1, 106) # 设置2列的宽度
self.tableWidget.setColumnWidth(2, 106) # 设置3列的宽度
self.tableWidget.setColumnWidth(3, 106) # 设置4列的宽度
self.tableWidget.setColumnWidth(4, 106) # 设置5列的宽度
self.tableWidget.setColumnWidth(5, 106) # 设置6列的宽度
self.tableWidget.setHorizontalHeaderLabels(["图片名称", "录入时间", "识别耗时", "车牌号码", "车牌类型", "车牌信息"])
self.tableWidget.setRowCount(self.RowLength)
self.tableWidget.verticalHeader().setVisible(False) # 隐藏垂直表头
self.tableWidget.setEditTriggers(QAbstractItemView.NoEditTriggers)
self.tableWidget.raise_()
self.scrollArea_2.setWidget(self.scrollAreaWidgetContents_1)
self.scrollArea_3 = QtWidgets.QScrollArea(self.centralwidget)
self.scrollArea_3.setGeometry(QtCore.QRect(690, 510, 341, 131))
self.scrollArea_3.setWidgetResizable(True)
self.scrollArea_3.setObjectName("scrollArea_3")
self.scrollAreaWidgetContents_3 = QtWidgets.QWidget()
self.scrollAreaWidgetContents_3.setGeometry(QtCore.QRect(0, 0, 339, 129))
self.scrollAreaWidgetContents_3.setObjectName("scrollAreaWidgetContents_3")
self.label_2 = QtWidgets.QLabel(self.scrollAreaWidgetContents_3)
self.label_2.setGeometry(QtCore.QRect(10, 10, 111, 20))
font = QtGui.QFont()
font.setPointSize(11)
self.label_2.setFont(font)
self.label_2.setObjectName("label_2")
self.label_3 = QtWidgets.QLabel(self.scrollAreaWidgetContents_3)
self.label_3.setGeometry(QtCore.QRect(10, 40, 321, 81))
self.label_3.setObjectName("label_3")
self.scrollArea_3.setWidget(self.scrollAreaWidgetContents_3)
self.scrollArea_4 = QtWidgets.QScrollArea(self.centralwidget)
self.scrollArea_4.setGeometry(QtCore.QRect(1040, 510, 161, 131))
self.scrollArea_4.setWidgetResizable(True)
self.scrollArea_4.setObjectName("scrollArea_4")
self.scrollAreaWidgetContents_4 = QtWidgets.QWidget()
self.scrollAreaWidgetContents_4.setGeometry(QtCore.QRect(0, 0, 159, 129))
self.scrollAreaWidgetContents_4.setObjectName("scrollAreaWidgetContents_4")
self.pushButton_2 = QtWidgets.QPushButton(self.scrollAreaWidgetContents_4)
self.pushButton_2.setGeometry(QtCore.QRect(10, 50, 80, 30))
self.pushButton_2.setObjectName("pushButton_2")
self.pushButton = QtWidgets.QPushButton(self.scrollAreaWidgetContents_4)
self.pushButton.setGeometry(QtCore.QRect(10, 90, 80, 30))
self.pushButton.setObjectName("pushButton")
self.pushButton_3 = QtWidgets.QPushButton(self.scrollAreaWidgetContents_4)
self.pushButton_3.setGeometry(QtCore.QRect(100, 50, 50, 70))
self.pushButton_3.setObjectName("pushButton_3")
self.label_4 = QtWidgets.QLabel(self.scrollAreaWidgetContents_4)
self.label_4.setGeometry(QtCore.QRect(10, 10, 111, 20))
font = QtGui.QFont()
font.setPointSize(11)
self.label_4.setFont(font)
self.label_4.setObjectName("label_4")
self.scrollArea_4.setWidget(self.scrollAreaWidgetContents_4)
MainWindow.setCentralWidget(self.centralwidget)
self.statusbar = QtWidgets.QStatusBar(MainWindow)
self.statusbar.setObjectName("statusbar")
MainWindow.setStatusBar(self.statusbar)
self.retranslateUi(MainWindow)
QtCore.QMetaObject.connectSlotsByName(MainWindow)
self.retranslateUi(MainWindow)
QtCore.QMetaObject.connectSlotsByName(MainWindow)
self.pushButton.clicked.connect(self.__openimage) # 设置点击事件
self.pushButton_2.clicked.connect(self.__writeFiles) # 设置点击事件
self.pushButton_3.clicked.connect(self.__openVideo) #设置事件
self.retranslateUi(MainWindow)
QtCore.QMetaObject.connectSlotsByName(MainWindow)
self.ProjectPath = os.getcwd() # 获取当前工程文件位置
def retranslateUi(self, MainWindow):
_translate = QtCore.QCoreApplication.translate
MainWindow.setWindowTitle(_translate("MainWindow", "车牌识别系统"))
self.label_0.setText(_translate("MainWindow", "原始图片:"))
self.label.setText(_translate("MainWindow", ""))
self.label_1.setText(_translate("MainWindow", "识别结果:"))
self.label_2.setText(_translate("MainWindow", "车牌区域:"))
self.label_3.setText(_translate("MainWindow", ""))
self.pushButton.setText(_translate("MainWindow", "打开文件"))
self.pushButton_2.setText(_translate("MainWindow", "导出数据"))
self.pushButton_3.setText(_translate("MainWindow", "摄像"))
self.label_4.setText(_translate("MainWindow", "控制面板:"))
self.scrollAreaWidgetContents_1.show()
2、识别入口函数
def __vlpr(self, path):
PR = PlateRecognition()
result = PR.VLPR(path)
return result
3、写入及导出Excel表格文件
def __show(self, result, FileName):
# 显示表格
self.RowLength = self.RowLength + 1
if self.RowLength > 18:
self.tableWidget.setColumnWidth(5, 157)
self.tableWidget.setRowCount(self.RowLength)
self.tableWidget.setItem(self.RowLength - 1, 0, QTableWidgetItem(FileName))
self.tableWidget.setItem(self.RowLength - 1, 1, QTableWidgetItem(result['InputTime']))
self.tableWidget.setItem(self.RowLength - 1, 2, QTableWidgetItem(str(result['UseTime']) + '秒'))
self.tableWidget.setItem(self.RowLength - 1, 3, QTableWidgetItem(result['Number']))
self.tableWidget.setItem(self.RowLength - 1, 4, QTableWidgetItem(result['Type']))
if result['Type'] == '蓝色牌照':
self.tableWidget.item(self.RowLength - 1, 4).setBackground(QBrush(QColor(3, 128, 255)))
elif result['Type'] == '绿色牌照':
self.tableWidget.item(self.RowLength - 1, 4).setBackground(QBrush(QColor(98, 198, 148)))
elif result['Type'] == '黄色牌照':
self.tableWidget.item(self.RowLength - 1, 4).setBackground(QBrush(QColor(242, 202, 9)))
self.tableWidget.setItem(self.RowLength - 1, 5, QTableWidgetItem(result['From']))
# 显示识别到的车牌位置
size = (int(self.label_3.width()), int(self.label_3.height()))
shrink = cv2.resize(result['Picture'], size, interpolation=cv2.INTER_AREA)
shrink = cv2.cvtColor(shrink, cv2.COLOR_BGR2RGB)
self.QtImg = QtGui.QImage(shrink[:], shrink.shape[1], shrink.shape[0], shrink.shape[1] * 3,
QtGui.QImage.Format_RGB888)
self.label_3.setPixmap(QtGui.QPixmap.fromImage(self.QtImg))
def __writexls(self, DATA, path):
wb = xlwt.Workbook();
ws = wb.add_sheet('Data');
for i, Data in enumerate(DATA):
for j, data in enumerate(Data):
ws.write(i, j, data)
wb.save(path)
QMessageBox.information(None, "成功", "数据已保存!", QMessageBox.Yes)
def __writecsv(self, DATA, path):
f = open(path, 'w')
# DATA.insert(0, ['文件名称','录入时间', '车牌号码', '车牌类型', '识别耗时', '车牌信息'])
for data in DATA:
f.write((',').join(data) + '\n')
f.close()
QMessageBox.information(None, "成功", "数据已保存!", QMessageBox.Yes)
def __writeFiles(self):
path, filetype = QFileDialog.getSaveFileName(None, "另存为", self.ProjectPath,
"Excel 工作簿(*.xls);;CSV (逗号分隔)(*.csv)")
if path == "": # 未选择
return
if filetype == 'Excel 工作簿(*.xls)':
self.__writexls(self.Data, path)
elif filetype == 'CSV (逗号分隔)(*.csv)': #逗号分隔开
self.__writecsv(self.Data, path)
4、图片识别入口
def __openimage(self):
path, filetype = QFileDialog.getOpenFileName(None, "选择文件", self.ProjectPath,
"JPEG Image (*.jpg);;PNG Image (*.png);;JFIF Image (*.jfif)") # ;;All Files (*)
if path == "": # 未选择文件
return
filename = path.split('/')[-1]
# 尺寸适配
size = cv2.imdecode(np.fromfile(path, dtype=np.uint8), cv2.IMREAD_COLOR).shape
if size[0] / size[1] > 1.0907:
w = size[1] * self.label.height() / size[0]
h = self.label.height()
jpg = QtGui.QPixmap(path).scaled(w, h)
elif size[0] / size[1] < 1.0907:
w = self.label.width()
h = size[0] * self.label.width() / size[1]
jpg = QtGui.QPixmap(path).scaled(w, h)
else:
jpg = QtGui.QPixmap(path).scaled(self.label.width(), self.label.height())
self.label.setPixmap(jpg) #保存jpg
result = self.__vlpr(path) #识别
if result is not None:
self.Data.append(
[filename, result['InputTime'], result['Number'], result['Type'], str(result['UseTime']) + '秒',
result['From']])
self.__show(result, filename)
else:
QMessageBox.warning(None, "Error", "无法识别此图像!", QMessageBox.Yes)
5、摄像头识别入口
def __openVideo(self):
cap = cv2.VideoCapture(0)
while True:
success, img = cap.read()
img1 = cv2.flip(img, 1)
cv2.imshow("VideoData", img1)
k = cv2.waitKey(1)
if cv2.getWindowProperty('VideoData', cv2.WND_PROP_VISIBLE) < 1:
break
elif k == ord("s"):
cv2.imwrite("index2.jpg", img1) #读取摄像头
cv2.destroyAllWindows()
cap.release()
6、重写MainWindow窗口
class MainWindow(QtWidgets.QMainWindow):
def closeEvent(self, event):
reply = QtWidgets.QMessageBox.question(self, '提示',
"是否要退出程序?\n提示:退出后将丢失所有识别数据",
QtWidgets.QMessageBox.Yes | QtWidgets.QMessageBox.No,
QtWidgets.QMessageBox.No)
if reply == QtWidgets.QMessageBox.Yes:
event.accept()
else:
event.ignore()
SVM训练模块
此模块主要用于对模型的准确性进行训练,包含字符中英文、数字的识别、图片尺寸的训练,最后将模型保存在svmchinese.dat中:
import cv2
import os
import numpy as np
from numpy.linalg import norm
from args import args
class StatModel(object):
def load(self, fn):
self.model = self.model.load(fn)
def save(self, fn):
self.model.save(fn)
class SVM(StatModel):
def __init__(self, C=1, gamma=0.5):
self.model = cv2.ml.SVM_create()
self.model.setGamma(gamma)
self.model.setC(C)
self.model.setKernel(cv2.ml.SVM_RBF)
self.model.setType(cv2.ml.SVM_C_SVC)
# 不能保证包括所有省份
# 训练svm
def train(self, samples, responses):
self.model.train(samples, cv2.ml.ROW_SAMPLE, responses)
# 字符识别
def predict(self, samples):
r = self.model.predict(samples)
return r[1].ravel()
# 定义参数
SZ = args.Size # 训练图片长宽
MAX_WIDTH = args.MAX_WIDTH # 原始图片最大宽度
Min_Area = args.Min_Area # 车牌区域允许最大面积
PROVINCE_START = args.PROVINCE_START
provinces = args.provinces
# 来自opencv的sample,用于svm训练
def deskew(img):
m = cv2.moments(img)
if abs(m['mu02']) < 1e-2:
return img.copy()
skew = m['mu11'] / m['mu02']
M = np.float32([[1, skew, -0.5 * SZ * skew], [0, 1, 0]])
img = cv2.warpAffine(img, M, (SZ, SZ), flags=cv2.WARP_INVERSE_MAP | cv2.INTER_LINEAR)
return img
# 来自opencv的sample,用于svm训练
def preprocess_hog(digits):
samples = []
for img in digits:
gx = cv2.Sobel(img, cv2.CV_32F, 1, 0)
gy = cv2.Sobel(img, cv2.CV_32F, 0, 1)
mag, ang = cv2.cartToPolar(gx, gy)
bin_n = 16
bin = np.int32(bin_n * ang / (2 * np.pi))
bin_cells = bin[:10, :10], bin[10:, :10], bin[:10, 10:], bin[10:, 10:]
mag_cells = mag[:10, :10], mag[10:, :10], mag[:10, 10:], mag[10:, 10:]
hists = [np.bincount(b.ravel(), m.ravel(), bin_n) for b, m in zip(bin_cells, mag_cells)]
hist = np.hstack(hists)
# transform to Hellinger kernel
eps = 1e-7
hist /= hist.sum() + eps
hist = np.sqrt(hist)
hist /= norm(hist) + eps
samples.append(hist)
return np.float32(samples)
def train_svm(path):
# 识别英文字母和数字
Model = SVM(C=1, gamma=0.5)
# 识别中文
Modelchinese = SVM(C=1, gamma=0.5)
# 英文字母和数字部分训练
chars_train = []
chars_label = []
for root, dirs, files in os.walk(os.path.join(path,'chars')):
if len(os.path.basename(root)) > 1:
continue
root_int = ord(os.path.basename(root))
for filename in files:
print('input:{}'.format(filename))
filepath = os.path.join(root, filename)
digit_img = cv2.imread(filepath)
digit_img = cv2.cvtColor(digit_img, cv2.COLOR_BGR2GRAY)
chars_train.append(digit_img)
chars_label.append(root_int)
chars_train = list(map(deskew, chars_train))
chars_train = preprocess_hog(chars_train)
chars_label = np.array(chars_label)
Model.train(chars_train, chars_label)
if not os.path.exists("svm.dat"):
# 保存模型
Model.save("svm.dat")
else:
# 更新模型
os.remove("svm.dat")
Model.save("svm.dat")
# 中文部分训练
chars_train = []
chars_label = []
for root, dirs, files in os.walk(os.path.join(path,'charsChinese')):
if not os.path.basename(root).startswith("zh_"):
continue
pinyin = os.path.basename(root)
index = provinces.index(pinyin) + PROVINCE_START + 1 # 1是拼音对应的汉字
for filename in files:
print('input:{}'.format(filename))
filepath = os.path.join(root, filename)
digit_img = cv2.imread(filepath)
digit_img = cv2.cvtColor(digit_img, cv2.COLOR_BGR2GRAY)
chars_train.append(digit_img)
chars_label.append(index)
chars_train = list(map(deskew, chars_train))
chars_train = preprocess_hog(chars_train)
chars_label = np.array(chars_label)
Modelchinese.train(chars_train, chars_label)
if not os.path.exists("svmchinese.dat"):
# 保存模型
Modelchinese.save("svmchinese.dat")
else:
# 更新模型
os.remove("svmchinese.dat")
Modelchinese.save("svmchinese.dat")
if __name__ == '__main__':
train_svm('train')
print('完成')
路由配置模块
from _collections import OrderedDict
# 导入Flask类
from flask import Flask, request, jsonify
from json_utils import jsonify
import numpy as np
import cv2
import time
from collections import OrderedDict
from Recognition import PlateRecognition
# 实例化
app = Flask(__name__)
PR = PlateRecognition()
# 设置编码-否则返回数据中文时候-乱码
app.config['JSON_AS_ASCII'] = False
# route()方法用于设定路由;类似spring路由配置
@app.route('/', methods=['POST']) # 在线识别
def forecast():
# 获取输入数据
stat = time.time()
file = request.files['image']
img_bytes = file.read()
image = np.asarray(bytearray(img_bytes), dtype="uint8")
image = cv2.imdecode(image, cv2.IMREAD_COLOR)
RES = PR.VLPR(image)
if RES is not None:
result = OrderedDict(
Error=0,
Errmsg='success',
InputTime=RES['InputTime'],
UseTime='{:.2f}'.format(time.time() - stat), # RES['UseTime'],
Number=RES['Number'],
From=RES['From'],
Type=RES['Type'],
List=RES['List'])
else:
result = OrderedDict(
Error=1,
Errmsg='unsuccess')
return jsonify(result)
if __name__ == '__main__':
# app.run(host, port, debug, options)
# 默认值:host=127.0.0.1(localhost), port=5000, debug=false
app.run()
# 本地路由地址,局域网下的主机均可通过该地址完成POST请求
# app.run(host='192.168.1.100' )
# 部署到服务器
# from waitress import serve
# serve(app, host=' IP ', port=5000)
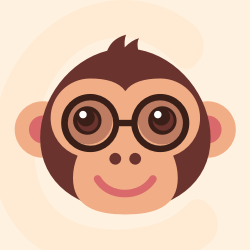



更多推荐
所有评论(0)