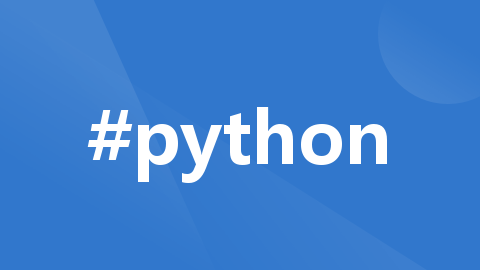
【学习总结】Python transformers AutoTokenizer encode 出现的 101 和 102
transformers
huggingface/transformers: 是一个基于 Python 的自然语言处理库,它使用了 PostgreSQL 数据库存储数据。适合用于自然语言处理任务的开发和实现,特别是对于需要使用 Python 和 PostgreSQL 数据库的场景。特点是自然语言处理库、Python、PostgreSQL 数据库。
项目地址:https://gitcode.com/gh_mirrors/tra/transformers

·
1. 代码展示:
from transformers import AutoTokenizer, AutoModel
model_name = "bert-base-chinese"
tokenizer = AutoTokenizer.from_pretrained(model_name)
model = AutoModel.from_pretrained(model_name)
print(len(tokenizer.vocab.keys()))
sequence = "法国的首都是巴黎"
print(tokenizer.vocab["法"])
tokens = tokenizer.tokenize(sequence)
print(tokens)
token_ids = tokenizer.convert_tokens_to_ids(tokens)
print(token_ids)
token_ids_s2e = tokenizer.encode(sequence)
print(token_ids_s2e)
输出结果:
21128
3791
['法', '国', '的', '首', '都', '是', '巴', '黎']
[3791, 1744, 4638, 7674, 6963, 3221, 2349, 7944]
[101, 3791, 1744, 4638, 7674, 6963, 3221, 2349, 7944, 102]
token_ids_s2e 中多了 101 和 102
sequence1 = tokenizer.decode(token_ids)
print(sequence1)
sequence2 = tokenizer.decode(token_ids_s2e)
print(sequence2)
输出结果:
法 国 的 首 都 是 巴 黎
[CLS] 法 国 的 首 都 是 巴 黎 [SEP]
101 代表 CLS,是文本的开头
102 代表 SEP,是文本的分隔符
2. 编解码多段文本
sequence_batch = ["法国的首都是巴黎","美国的首都是华盛顿特区" ]
token_ids_batch = tokenizer.encode(sequence_batch)
print(token_ids_batch)
sequence_batch = tokenizer.decode(token_ids_batch)
print(sequence_batch)
输出结果:
[101, 3791, 1744, 4638, 7674, 6963, 3221, 2349, 7944, 102, 5401, 1744, 4638, 7674, 6963, 3221, 1290, 4670, 7561, 4294, 1277, 102]
[CLS] 法 国 的 首 都 是 巴 黎 [SEP] 美 国 的 首 都 是 华 盛 顿 特 区 [SEP]
3. 实际操作
embedding_batch = tokenizer("法国的首都是巴黎","美国的首都是华盛顿特区")
print(embedding_batch)
输出:
{'input_ids': [101, 3791, 1744, 4638, 7674, 6963, 3221, 2349, 7944, 102, 5401, 1744, 4638, 7674, 6963, 3221, 1290, 4670, 7561, 4294, 1277, 102], 'token_type_ids': [0, 0, 0, 0, 0, 0, 0, 0, 0, 0, 1, 1, 1, 1, 1, 1, 1, 1, 1, 1, 1, 1], 'attention_mask': [1, 1, 1, 1, 1, 1, 1, 1, 1, 1, 1, 1, 1, 1, 1, 1, 1, 1, 1, 1, 1, 1]}
优化代码
for key, value in embedding_batch.items():
print(f"{key}: {value}\n")
输出:
input_ids: [101, 3791, 1744, 4638, 7674, 6963, 3221, 2349, 7944, 102, 5401, 1744, 4638, 7674, 6963, 3221, 1290, 4670, 7561, 4294, 1277, 102]
token_type_ids: [0, 0, 0, 0, 0, 0, 0, 0, 0, 0, 1, 1, 1, 1, 1, 1, 1, 1, 1, 1, 1, 1]
attention_mask: [1, 1, 1, 1, 1, 1, 1, 1, 1, 1, 1, 1, 1, 1, 1, 1, 1, 1, 1, 1, 1, 1]
编码后返回结果是:
input_ids: token_ids
token_type_ids: token_id 归属的句子编号
attention_mask: 指示哪些token需要被关注(注意力机制)
4. 查看词表
from itertools import islice
# 使用 islice 查看词表部分内容
for key, value in islice(tokenizer.vocab.items(), 30,40):
print(f"{key}: {value}")
输出结果:
叼: 1388
赓: 6607
##禀: 17937
骡: 7751
ing: 10139
滙: 4002
##楼: 16574
##部: 20013
##针: 20208
##酥: 20046
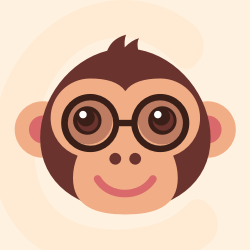



huggingface/transformers: 是一个基于 Python 的自然语言处理库,它使用了 PostgreSQL 数据库存储数据。适合用于自然语言处理任务的开发和实现,特别是对于需要使用 Python 和 PostgreSQL 数据库的场景。特点是自然语言处理库、Python、PostgreSQL 数据库。
最近提交(Master分支:2 个月前 )
33868a05
* [i18n-HI] Translated accelerate page to Hindi
* Update docs/source/hi/accelerate.md
Co-authored-by: K.B.Dharun Krishna <kbdharunkrishna@gmail.com>
* Update docs/source/hi/accelerate.md
Co-authored-by: K.B.Dharun Krishna <kbdharunkrishna@gmail.com>
* Update docs/source/hi/accelerate.md
Co-authored-by: K.B.Dharun Krishna <kbdharunkrishna@gmail.com>
* Update docs/source/hi/accelerate.md
Co-authored-by: K.B.Dharun Krishna <kbdharunkrishna@gmail.com>
---------
Co-authored-by: Kay <kay@Kays-MacBook-Pro.local>
Co-authored-by: K.B.Dharun Krishna <kbdharunkrishna@gmail.com> 2 小时前
e2ac16b2
* rework converter
* Update modular_model_converter.py
* Update modular_model_converter.py
* Update modular_model_converter.py
* Update modular_model_converter.py
* cleaning
* cleaning
* finalize imports
* imports
* Update modular_model_converter.py
* Better renaming to avoid visiting same file multiple times
* start converting files
* style
* address most comments
* style
* remove unused stuff in get_needed_imports
* style
* move class dependency functions outside class
* Move main functions outside class
* style
* Update modular_model_converter.py
* rename func
* add augmented dependencies
* Update modular_model_converter.py
* Add types_to_file_type + tweak annotation handling
* Allow assignment dependency mapping + fix regex
* style + update modular examples
* fix modular_roberta example (wrong redefinition of __init__)
* slightly correct order in which dependencies will appear
* style
* review comments
* Performance + better handling of dependencies when they are imported
* style
* Add advanced new classes capabilities
* style
* add forgotten check
* Update modeling_llava_next_video.py
* Add prority list ordering in check_conversion as well
* Update check_modular_conversion.py
* Update configuration_gemma.py 9 小时前
更多推荐
所有评论(0)