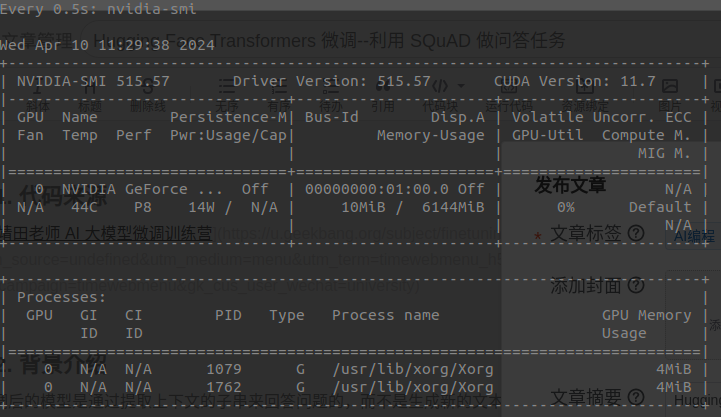
Hugging Face Transformers 微调--利用 SQuAD 做问答任务
transformers
huggingface/transformers: 是一个基于 Python 的自然语言处理库,它使用了 PostgreSQL 数据库存储数据。适合用于自然语言处理任务的开发和实现,特别是对于需要使用 Python 和 PostgreSQL 数据库的场景。特点是自然语言处理库、Python、PostgreSQL 数据库。
项目地址:https://gitcode.com/gh_mirrors/tra/transformers

·
1. 代码来源
2. 背景介绍
微调后的模型是通过提取上下文的子串来回答问题的,而不是生成新的文本,也不能连续对话。
3. 代码整理
from datasets import load_dataset
from datasets import load_metric
from transformers import AutoTokenizer
from transformers import AutoModelForQuestionAnswering, TrainingArguments, Trainer
from transformers import default_data_collator
import torch
import collections
import numpy as np
from tqdm.auto import tqdm
# The maximum length of a feature (question and context)
max_length = 384
# The authorized overlap between two part of the context when splitting it is needed.
doc_stride = 120
def prepare_train_features(examples):
# 一些问题的左侧可能有很多空白字符,这对我们没有用,而且会导致上下文的截断失败
# (标记化的问题将占用大量空间)。因此,我们删除左侧的空白字符。
examples["question"] = [q.lstrip() for q in examples["question"]]
# 使用截断和填充对我们的示例进行标记化,但保留溢出部分,使用步幅(stride)。
# 当上下文很长时,这会导致一个示例可能提供多个特征,其中每个特征的上下文都与前一个特征的上下文有一些重叠。
tokenized_examples = tokenizer(
examples["question" if pad_on_right else "context"],
examples["context" if pad_on_right else "question"],
truncation="only_second" if pad_on_right else "only_first",
max_length=max_length,
stride=doc_stride,
return_overflowing_tokens=True,
return_offsets_mapping=True,
padding="max_length",
)
# 由于一个示例可能给我们提供多个特征(如果它具有很长的上下文),我们需要一个从特征到其对应示例的映射。这个键就提供了这个映射关系。
sample_mapping = tokenized_examples.pop("overflow_to_sample_mapping")
# 偏移映射将为我们提供从令牌到原始上下文中的字符位置的映射。这将帮助我们计算开始位置和结束位置。
offset_mapping = tokenized_examples.pop("offset_mapping")
# 让我们为这些示例进行标记!
tokenized_examples["start_positions"] = []
tokenized_examples["end_positions"] = []
for i, offsets in enumerate(offset_mapping):
# 我们将使用 CLS 特殊 token 的索引来标记不可能的答案。
input_ids = tokenized_examples["input_ids"][i]
# tokenizer.cls_token_id是特殊标记(通常是"[CLS]")在词汇表中的索引
cls_index = input_ids.index(tokenizer.cls_token_id)
# 获取与该示例对应的序列(以了解上下文和问题是什么)。
sequence_ids = tokenized_examples.sequence_ids(i)
# 一个示例可以提供多个跨度,这是包含此文本跨度的示例的索引。
sample_index = sample_mapping[i]
answers = examples["answers"][sample_index]
# 如果没有给出答案,则将cls_index设置为答案。判断语句检查答案的长度是否为零,即是否存在答案
if len(answers["answer_start"]) == 0:
tokenized_examples["start_positions"].append(cls_index)
tokenized_examples["end_positions"].append(cls_index)
else:
# 答案在文本中的开始和结束字符索引。
start_char = answers["answer_start"][0]
end_char = start_char + len(answers["text"][0])
# 当前跨度在文本中的开始令牌索引。
token_start_index = 0
while sequence_ids[token_start_index] != (1 if pad_on_right else 0):
token_start_index += 1
# 当前跨度在文本中的结束令牌索引。
token_end_index = len(input_ids) - 1
while sequence_ids[token_end_index] != (1 if pad_on_right else 0):
token_end_index -= 1
# 检测答案是否超出跨度(在这种情况下,该特征的标签将使用CLS索引)。
if not (offsets[token_start_index][0] <= start_char and offsets[token_end_index][1] >= end_char):
tokenized_examples["start_positions"].append(cls_index)
tokenized_examples["end_positions"].append(cls_index)
else:
# 否则,将token_start_index和token_end_index移到答案的两端。
# 注意:如果答案是最后一个单词(边缘情况),我们可以在最后一个偏移之后继续。
while token_start_index < len(offsets) and offsets[token_start_index][0] <= start_char:
token_start_index += 1
tokenized_examples["start_positions"].append(token_start_index - 1)
while offsets[token_end_index][1] >= end_char:
token_end_index -= 1
tokenized_examples["end_positions"].append(token_end_index + 1)
return tokenized_examples
def prepare_validation_features(examples):
# 一些问题的左侧有很多空白,这些空白并不有用且会导致上下文截断失败(分词后的问题会占用很多空间)。
# 因此我们移除这些左侧空白
examples["question"] = [q.lstrip() for q in examples["question"]]
# 使用截断和可能的填充对我们的示例进行分词,但使用步长保留溢出的令牌。这导致一个长上下文的示例可能产生
# 几个特征,每个特征的上下文都会稍微与前一个特征的上下文重叠。
tokenized_examples = tokenizer(
examples["question" if pad_on_right else "context"],
examples["context" if pad_on_right else "question"],
truncation="only_second" if pad_on_right else "only_first",
max_length=max_length,
stride=doc_stride,
return_overflowing_tokens=True,
return_offsets_mapping=True,
padding="max_length",
)
# 由于一个示例在上下文很长时可能会产生几个特征,我们需要一个从特征映射到其对应示例的映射。这个键就是为了这个目的。
sample_mapping = tokenized_examples.pop("overflow_to_sample_mapping")
# 我们保留产生这个特征的示例ID,并且会存储偏移映射。
tokenized_examples["example_id"] = []
for i in range(len(tokenized_examples["input_ids"])):
# 获取与该示例对应的序列(以了解哪些是上下文,哪些是问题)。
sequence_ids = tokenized_examples.sequence_ids(i)
context_index = 1 if pad_on_right else 0
# 一个示例可以产生几个文本段,这里是包含该文本段的示例的索引。
sample_index = sample_mapping[i]
tokenized_examples["example_id"].append(examples["id"][sample_index])
# 将不属于上下文的偏移映射设置为None,以便容易确定一个令牌位置是否属于上下文。
tokenized_examples["offset_mapping"][i] = [
(o if sequence_ids[k] == context_index else None)
for k, o in enumerate(tokenized_examples["offset_mapping"][i])
]
return tokenized_examples
def postprocess_qa_predictions(examples, features, raw_predictions, n_best_size=20, max_answer_length=30):
all_start_logits, all_end_logits = raw_predictions
# 构建一个从示例到其对应特征的映射。
example_id_to_index = {k: i for i, k in enumerate(examples["id"])}
features_per_example = collections.defaultdict(list)
for i, feature in enumerate(features):
features_per_example[example_id_to_index[feature["example_id"]]].append(i)
# 我们需要填充的字典。
predictions = collections.OrderedDict()
# 日志记录。
print(f"正在后处理 {len(examples)} 个示例的预测,这些预测分散在 {len(features)} 个特征中。")
# 遍历所有示例!
for example_index, example in enumerate(tqdm(examples)):
# 这些是与当前示例关联的特征的索引。
feature_indices = features_per_example[example_index]
min_null_score = None # 仅在squad_v2为True时使用。
valid_answers = []
context = example["context"]
# 遍历与当前示例关联的所有特征。
for feature_index in feature_indices:
# 我们获取模型对这个特征的预测。
start_logits = all_start_logits[feature_index]
end_logits = all_end_logits[feature_index]
# 这将允许我们将logits中的某些位置映射到原始上下文中的文本跨度。
offset_mapping = features[feature_index]["offset_mapping"]
# 更新最小空预测。
cls_index = features[feature_index]["input_ids"].index(tokenizer.cls_token_id)
feature_null_score = start_logits[cls_index] + end_logits[cls_index]
if min_null_score is None or min_null_score < feature_null_score:
min_null_score = feature_null_score
# 浏览所有的最佳开始和结束logits,为 `n_best_size` 个最佳选择。
start_indexes = np.argsort(start_logits)[-1: -n_best_size - 1: -1].tolist()
end_indexes = np.argsort(end_logits)[-1: -n_best_size - 1: -1].tolist()
for start_index in start_indexes:
for end_index in end_indexes:
# 不考虑超出范围的答案,原因是索引超出范围或对应于输入ID的部分不在上下文中。
if (
start_index >= len(offset_mapping)
or end_index >= len(offset_mapping)
or offset_mapping[start_index] is None
or offset_mapping[end_index] is None
):
continue
# 不考虑长度小于0或大于max_answer_length的答案。
if end_index < start_index or end_index - start_index + 1 > max_answer_length:
continue
start_char = offset_mapping[start_index][0]
end_char = offset_mapping[end_index][1]
valid_answers.append(
{
"score": start_logits[start_index] + end_logits[end_index],
"text": context[start_char: end_char]
}
)
if len(valid_answers) > 0:
best_answer = sorted(valid_answers, key=lambda x: x["score"], reverse=True)[0]
else:
# 在极少数情况下我们没有一个非空预测,我们创建一个假预测以避免失败。
best_answer = {"text": "", "score": 0.0}
# 选择我们的最终答案:最佳答案或空答案(仅适用于squad_v2)
if not squad_v2:
predictions[example["id"]] = best_answer["text"]
else:
answer = best_answer["text"] if best_answer["score"] > min_null_score else ""
predictions[example["id"]] = answer
return predictions
# 1. 加载数据
squad_v2 = False
datasets = load_dataset("squad_v2" if squad_v2 else "squad")
# 2. 预处理数据
model_checkpoint = "distilbert-base-uncased"
tokenizer = AutoTokenizer.from_pretrained(model_checkpoint)
import transformers
assert isinstance(tokenizer, transformers.PreTrainedTokenizerFast)
"""
关于填充的策略
对于没有超过最大长度的文本,填充补齐长度。
对于需要左侧填充的模型,交换 question 和 context 顺序"""
pad_on_right = tokenizer.padding_side == "right"
"""
使用 datasets.map 方法将 prepare_train_features 应用于所有训练、验证和测试数据:
batched: 批量处理数据。
remove_columns: 因为预处理更改了样本的数量,所以在应用它时需要删除旧列。
load_from_cache_file:是否使用datasets库的自动缓存
datasets 库针对大规模数据,实现了高效缓存机制,能够自动检测传递给 map 的函数是否已更改(因此需要不使用缓存数据)
如果在调用 map 时设置 load_from_cache_file=False,可以强制重新应用预处理。"""
tokenized_datasets = datasets.map(prepare_train_features,
batched=True,
remove_columns=datasets["train"].column_names)
# 3. 模型微调
"""
警告通知我们正在丢弃一些权重(vocab_transform 和 vocab_layer_norm 层),
并随机初始化其他一些权重(pre_classifier 和 classifier 层)。
在微调模型情况下是绝对正常的,因为我们正在删除用于预训练模型的掩码语言建模任务的头部,并用一个新的头部替换它,
对于这个新头部,我们没有预训练的权重,所以库会警告我们在用它进行推理之前应该对这个模型进行微调,而这正是我们要做的事情。
"""
model_dir="fine_model/distilbert-base-uncased-squad"
model = AutoModelForQuestionAnswering.from_pretrained(model_checkpoint)
batch_size = 12
training_args = TrainingArguments(
output_dir=model_dir,
evaluation_strategy = "epoch",
per_device_train_batch_size=batch_size,
per_device_eval_batch_size=batch_size,
num_train_epochs=1,#200
weight_decay=0.01,
)
#数据整理器将训练数据整理为批次数据,用于模型训练时的批次处理。使用默认的 default_data_collator
data_collator = default_data_collator
trainer = Trainer(
model,
training_args,
train_dataset=tokenized_datasets["train"],
eval_dataset=tokenized_datasets["validation"],
data_collator=data_collator,
tokenizer=tokenizer,
)
trainer.train()
# 4.保存模型
model_to_save = trainer.save_model(model_dir)
# 5.模型评估
validation_features = datasets["validation"].map(
prepare_validation_features,
batched=True,
remove_columns=datasets["validation"].column_names
)
raw_predictions = trainer.predict(validation_features)
validation_features.set_format(type=validation_features.format["type"], columns=list(validation_features.features.keys()))
examples = datasets["validation"]
features = validation_features
example_id_to_index = {k: i for i, k in enumerate(examples["id"])}
features_per_example = collections.defaultdict(list)
for i, feature in enumerate(features):
features_per_example[example_id_to_index[feature["example_id"]]].append(i)
# 6. 结果后处理
final_predictions = postprocess_qa_predictions(datasets["validation"], validation_features, raw_predictions.predictions)
metric = load_metric("squad_v2" if squad_v2 else "squad")
if squad_v2:
formatted_predictions = [{"id": k, "prediction_text": v, "no_answer_probability": 0.0} for k, v in final_predictions.items()]
else:
formatted_predictions = [{"id": k, "prediction_text": v} for k, v in final_predictions.items()]
references = [{"id": ex["id"], "answers": ex["answers"]} for ex in datasets["validation"]]
metric.compute(predictions=formatted_predictions, references=references)
4. 代码补充
4.1 模型训练结束后,查看评估结果
#从 trainer 对象的评估数据加载器中获取一个批次的数据
for batch in trainer.get_eval_dataloader():
break
# 将批次的数据转移到指定的设备上。
# 它使用了字典推导式,遍历 batch 中的每个键值对,并将对应的值(张量)通过 .to() 方法移动到 trainer.args.device 指定的设备上。
batch = {k: v.to(trainer.args.device) for k, v in batch.items()}
# 上下文管理器用于关闭梯度计算,以节省内存和加速推理。
# 使用 trainer.model 对输入 batch 进行前向推理
with torch.no_grad():
output = trainer.model(**batch)
# 获取了推理的输出,并使用 .keys() 方法获取输出的所有键
print(output)
print(output.keys())
print(output.start_logits.shape, output.end_logits.shape)
print(output.start_logits.argmax(dim=-1), output.end_logits.argmax(dim=-1))
输出结果:
QuestionAnsweringModelOutput(loss=tensor(1.4979), start_logits=tensor([[ -8.4187, -10.6108, -10.1990, ..., -11.0045, -10.9862, -10.9882],
[ -8.8760, -10.5771, -10.3793, ..., -10.9571, -10.9342, -10.9335],
[ -6.8497, -8.1159, -9.3868, ..., -10.6954, -10.6619, -10.6703],
...,
[ -9.4415, -9.5207, -9.7684, ..., -10.7376, -10.7485, -10.6934],
[ -8.4753, -9.9112, -10.2897, ..., -10.8912, -10.8770, -10.8730],
[ -7.2636, -9.4387, -10.3689, ..., -10.9233, -10.9299, -10.9402]]), end_logits=tensor([[ -7.6437, -9.5591, -9.1688, ..., -10.1736, -10.2071, -10.2030],
[ -8.3957, -9.7669, -9.5673, ..., -10.2761, -10.3054, -10.3011],
[ -7.1422, -9.7213, -9.8176, ..., -10.5448, -10.5825, -10.5864],
...,
[ -9.4451, -10.4809, -10.2968, ..., -10.5505, -10.5421, -10.6084],
[ -7.9945, -9.5457, -8.9810, ..., -10.3400, -10.3655, -10.3765],
[ -6.7163, -9.9266, -9.5512, ..., -10.3350, -10.3313, -10.3238]]), hidden_states=None, attentions=None)
odict_keys(['loss', 'start_logits', 'end_logits'])
(torch.Size([8, 384]), torch.Size([8, 384]))
(tensor([ 46, 57, 78, 43, 118, 107, 72, 35]),
tensor([ 47, 58, 81, 44, 118, 110, 75, 37]))
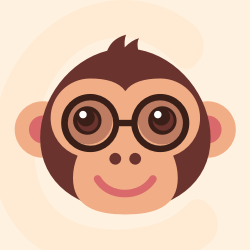



huggingface/transformers: 是一个基于 Python 的自然语言处理库,它使用了 PostgreSQL 数据库存储数据。适合用于自然语言处理任务的开发和实现,特别是对于需要使用 Python 和 PostgreSQL 数据库的场景。特点是自然语言处理库、Python、PostgreSQL 数据库。
最近提交(Master分支:2 个月前 )
33868a05
* [i18n-HI] Translated accelerate page to Hindi
* Update docs/source/hi/accelerate.md
Co-authored-by: K.B.Dharun Krishna <kbdharunkrishna@gmail.com>
* Update docs/source/hi/accelerate.md
Co-authored-by: K.B.Dharun Krishna <kbdharunkrishna@gmail.com>
* Update docs/source/hi/accelerate.md
Co-authored-by: K.B.Dharun Krishna <kbdharunkrishna@gmail.com>
* Update docs/source/hi/accelerate.md
Co-authored-by: K.B.Dharun Krishna <kbdharunkrishna@gmail.com>
---------
Co-authored-by: Kay <kay@Kays-MacBook-Pro.local>
Co-authored-by: K.B.Dharun Krishna <kbdharunkrishna@gmail.com> 3 小时前
e2ac16b2
* rework converter
* Update modular_model_converter.py
* Update modular_model_converter.py
* Update modular_model_converter.py
* Update modular_model_converter.py
* cleaning
* cleaning
* finalize imports
* imports
* Update modular_model_converter.py
* Better renaming to avoid visiting same file multiple times
* start converting files
* style
* address most comments
* style
* remove unused stuff in get_needed_imports
* style
* move class dependency functions outside class
* Move main functions outside class
* style
* Update modular_model_converter.py
* rename func
* add augmented dependencies
* Update modular_model_converter.py
* Add types_to_file_type + tweak annotation handling
* Allow assignment dependency mapping + fix regex
* style + update modular examples
* fix modular_roberta example (wrong redefinition of __init__)
* slightly correct order in which dependencies will appear
* style
* review comments
* Performance + better handling of dependencies when they are imported
* style
* Add advanced new classes capabilities
* style
* add forgotten check
* Update modeling_llava_next_video.py
* Add prority list ordering in check_conversion as well
* Update check_modular_conversion.py
* Update configuration_gemma.py 9 小时前
更多推荐
所有评论(0)