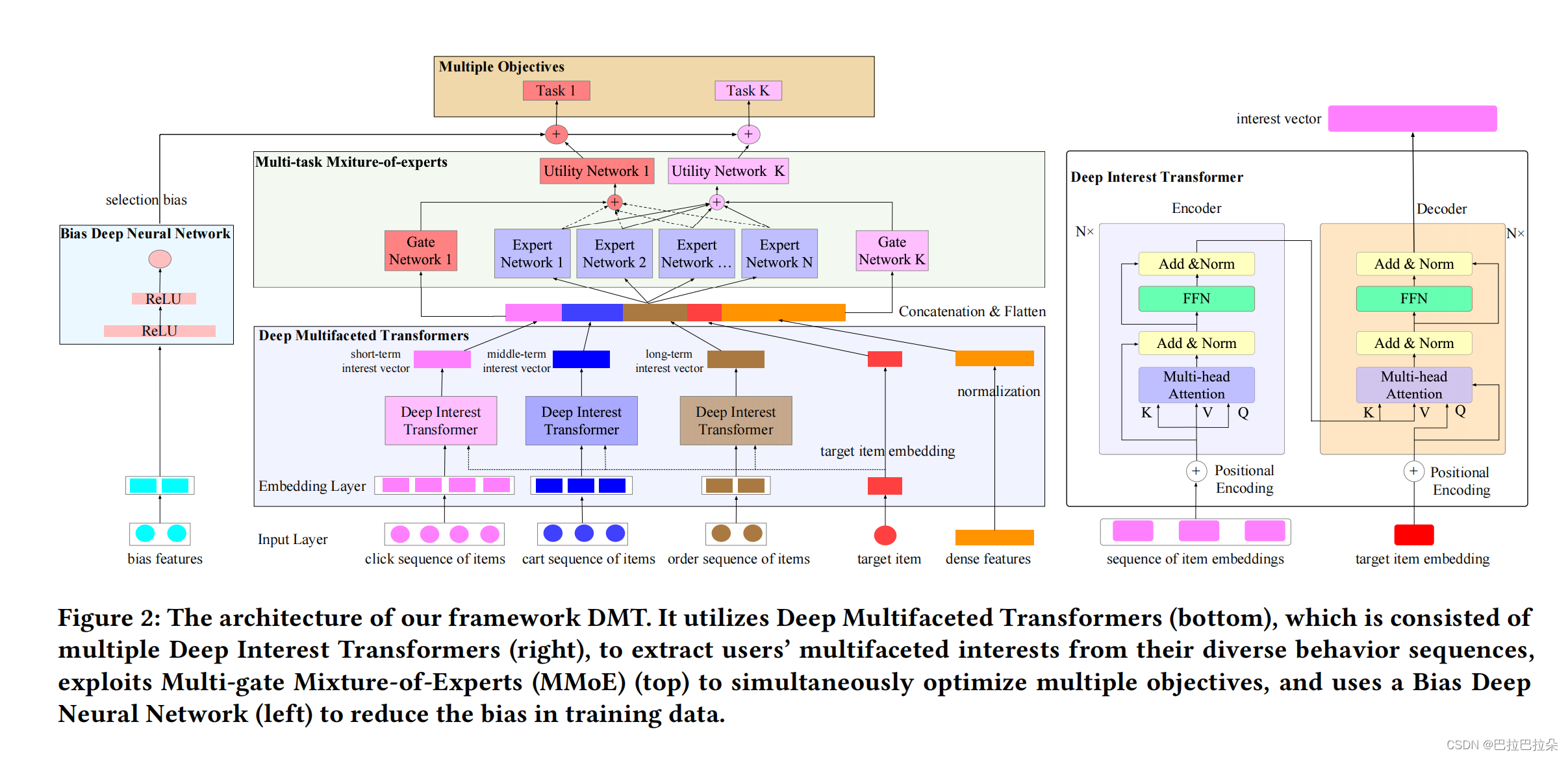
论文《Deep Multifaceted Transformers for Multi-objective Ranking in Large-Scale E-commerce Recommender》

京东DMT
论文地址:https://dl.acm.org/doi/pdf/10.1145/3340531.3412697
论文提出用多个Transformer对用户多种类型的行为序列进行建模,在此基础上叠加MMOE建模多目标,最后使用一个消偏塔对数据进行消偏。
拿点击/未点击作为反馈通常会有位置偏差(position bias)和近邻偏差(neighboring
bias),不过论文对于消偏的处理比较简单。
DMT的结构如下
输入分为两种特征 Categorical features 和 Dense features
Categorical features:
1.用户不同的行为序列。
S
=
<
s
1
,
s
2
,
.
.
.
,
s
T
>
S = <s_1, s_2, ..., s_T>
S=<s1,s2,...,sT>,其中
s
t
=
(
t
i
,
p
i
)
s_t=(t_i,p_i)
st=(ti,pi)表示用户在时刻
t
i
t_i
ti交互的物料
p
i
p_i
pi,论文用到了点击click序列
S
c
S_c
Sc,加入购物车cart序列
S
a
S_a
Sa,购买order序列
S
o
S_o
So
2. Embedding Layer,对每个物料,使用物料id
p
i
p_i
pi,类目id
c
i
c_i
ci,品牌id
b
i
b_i
bi,商铺id
s
i
s_i
si,分别映射成低维向量
e
p
i
,
e
c
i
,
e
b
i
,
e
s
i
e_{p_i}, e_{c_i}, e_{b_i}, e_{s_i}
epi,eci,ebi,esi,然后concat起来,形成向量
e
i
e_i
ei
Dense features: 归一化处理
1.item profile features (e.g.,number of clicks, CTR, CVR, rating) ,
2. profile features (e.g., purchase power, preferred categories and brands),
3. user-item matching features (e.g., whether the item matches the user’s gender or age)
4. user-item interaction features (e.g., number of clicks on the category of the item within a time window)
Deep Multifaceted Transformers Layer
分别用3个Transformer来对点击、加入购物车、购买行为序列进行建模。encoder中,用序列的item-Embedding作为self-attention的输入,decoder中,使用target item的Embedding作为query,encoder输出的结果作为key和value。
Multi-gate Mixture-of-Experts Layers
专家网络输出
e
1
(
x
)
,
e
2
(
x
)
,
.
.
.
,
e
N
(
x
)
e_1(x),e_2(x),...,e_N(x)
e1(x),e2(x),...,eN(x),每个任务的门控网络
N
N
G
k
(
x
)
NNG^k(x)
NNGk(x)学习各个专家的权重
w
k
w^k
wk,根据权重得到专家结果的加权和,然后送入到一个功能网络中得到任务在MMOE层的输出。
w
k
=
s
o
f
t
m
a
x
(
N
N
G
k
(
x
)
)
w^k = softmax(NNG^k(x))
wk=softmax(NNGk(x))
f
k
(
x
)
=
∑
i
=
1
N
w
i
k
e
i
(
x
)
f^k(x) = \sum_{i=1}^N w_i^k e_i(x)
fk(x)=i=1∑Nwikei(x)
u
k
=
N
N
U
k
(
f
k
(
x
)
)
u_k = NN_U^k(f^k(x))
uk=NNUk(fk(x))
Bias Deep Neural Network
专门搭了一个bais塔,输入都是偏差相关的特征,对于位置偏差就是展示位置索引编号或者网页索引编号;对于近邻偏差,输入就是目标物料的类目和邻近K个物料的类目。
biase塔的输出
y
b
=
N
N
B
(
x
b
)
y_b = NN_B(x_b)
yb=NNB(xb)
Model Training and Prediction
模型输出
y
k
y_k
yk
都是分类任务,使用交叉熵损失函数
y
k
=
σ
(
u
k
+
y
b
)
y_k = \sigma (u_k+y_b)
yk=σ(uk+yb)
L
k
=
−
1
N
∑
i
=
1
N
y
i
log
(
y
k
)
+
(
1
−
y
i
)
log
(
1
−
y
k
)
L_k = - \frac 1 N \sum_{i=1}^N y_i \log(y_k) + (1-y_i) \log (1-y_k)
Lk=−N1i=1∑Nyilog(yk)+(1−yi)log(1−yk)
L
=
∑
i
=
1
K
λ
k
L
k
L = \sum_{i=1}^K \lambda_k L_k
L=i=1∑KλkLk
上面是训练阶段,预测阶段,任务k输
y
^
k
\hat y_k
y^k,score由不同任务预估分加权得到,权重离线搜参得到
y
^
k
=
σ
(
u
k
)
\hat y_k = \sigma (u_k)
y^k=σ(uk)
y
^
=
∑
k
=
1
K
w
k
y
^
k
∑
k
=
1
K
w
k
\hat y = \frac {\sum_{k=1}^Kw_k \hat y_k} {\sum_{k=1}^Kw_k}
y^=∑k=1Kwk∑k=1Kwky^k
EXPERIMENTAL
其实这个论文是对Transformer和MMOE以及消偏做了组合,不同的Transformer对不同种类的序列分别处理,能拿到一个比较好的这个序列的Embedding结果,这种组合竟然可以很好地work,说明几个基础组件还是非常有效的。
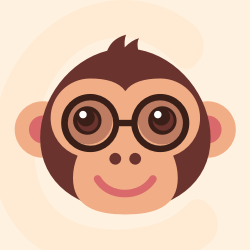



更多推荐
所有评论(0)