论文笔记:Autoformer: Decomposition Transformers with Auto-Correlation for Long-Term Series Forecasting
transformers
huggingface/transformers: 是一个基于 Python 的自然语言处理库,它使用了 PostgreSQL 数据库存储数据。适合用于自然语言处理任务的开发和实现,特别是对于需要使用 Python 和 PostgreSQL 数据库的场景。特点是自然语言处理库、Python、PostgreSQL 数据库。
项目地址:https://gitcode.com/gh_mirrors/tra/transformers

·
Neurips 2021
1 Intro
- 针对长期时间序列预测,很多模型使用自注意力机制,并获得了比较好的结果
- 但是,在“长期”这个问题配置下,预测任务是很有挑战的:
- 很难直接从长期时间序列中得到准确、可靠的时间依赖关系,因为各种时间特征(如趋势、周期性等)可能被纠缠在一起
- 传统带self-attention的Transformer模型,在计算长期时间序列预测的过程中,计算量过大【(On^2)的计算量】
- 基于Transformer的模型不得不使用稀疏形式的注意力机制来应对二次复杂度的问题,减少计算量(如logSparseTrans,Informer等)
-
但造成了信息利用的瓶颈
- ——>这也是长期时间序列的一个瓶颈
- 但是,在“长期”这个问题配置下,预测任务是很有挑战的:
- 这篇论文提出了Autoformer
- 突破将序列分解作为预处理的传统方法,提出深度分解架构,能够从复杂时间模式中分解出可预测性更强的组分。
- 提出自相关机制(Auto-Correlation),代替点向连接的注意力机制,实现序列级(series-wise)连接和O(LlogL)的复杂度,打破信息利用瓶颈。
2 Autoformer
2.1 深度分解架构
在预测过程中,逐步从隐变量中分离趋势项与周期项,实现渐进式分解,实现分解、预测结果优化的交替进行、相互促进
- 对于输入时间序列
,我们进行如下的分解,得到趋势项和周期项
- AvgPool是滑动平均
- Padding的作用是滑动平均之后,时间序列长度不变
- 论文后续统一使用
来代替前面的两个式子
2.2 模型输入
- encoder的输入
- decoder的输入
,
- 其中:
是O位的0
是
的平均
- 其中:
2.3 Encoder
逐步消除趋势项(这部分会在Deocder中通过累积得到)
2.4 Decoder
对趋势项与周期项分别预测。
-
对于周期项,使用自相关机制,基于序列的周期性质来进行依赖挖掘,并聚合具有相似过程的子序列;
-
对于趋势项,使用累积的方式,逐步从预测的隐变量中提取出趋势信息。
注:不管第几个decoder block,第二个auto-correlation看到的都是最后一个encoder的输出
2.5 Auto-Correlation
聚合不同周期的相似子过程
- 对于时间序列Xt,定义auto-correlation为:
- 反映了Xt和τ时延之前的相似关系
- 选择最有可能(auto-correlation最大)的k(
)个τ:
- ——》相当于估计出的周期
- 对这些选中的τ对应的autocorrelation值进行softmax
- Roll的意思是将时延的部分接到时间序列后面
- 将Roll后的结果,乘以对应Softmax的结果,加权求和
2.6 高效的计算方法
根据Wiener–Khinchin理论
- F表示FFT,F-1表示逆FFT,*表示共轭
所以AutoCorrelation可以写成
2.7 和自注意力的对比
3 实验部分
3.1 Multivariate Time Series预测结果
3.2 Univariate 预测结果
3.3 使用不同的attention 的效果
3.4 渐进分离效果
随着序列分解单元的数量增加,模型的学到的趋势项会越来越接近数据真实结果,周期项可以更好的捕捉序列变化情况,这验证了渐进式分解的作用。
3.5 时序依赖可视化
通过对比可以发现,Autoformer中自相关机制可以正确发掘出每个周期中的下降过程,并且没有误识别和漏识别,而自注意力机制存在错误和缺漏
3.6 效率分析
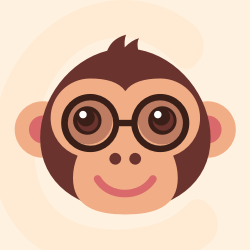



huggingface/transformers: 是一个基于 Python 的自然语言处理库,它使用了 PostgreSQL 数据库存储数据。适合用于自然语言处理任务的开发和实现,特别是对于需要使用 Python 和 PostgreSQL 数据库的场景。特点是自然语言处理库、Python、PostgreSQL 数据库。
最近提交(Master分支:6 个月前 )
8eaae6be
* Added Support for Custom Quantization
* Update code
* code reformatted
* Updated Changes
* Updated Changes
---------
Co-authored-by: Mohamed Mekkouri <93391238+MekkCyber@users.noreply.github.com> 22 天前
07182b2e
Flatten the expected slice tensor 22 天前
更多推荐
所有评论(0)