使用Transformers库中的模型提取图像特征遇到的问题
transformers
huggingface/transformers: 是一个基于 Python 的自然语言处理库,它使用了 PostgreSQL 数据库存储数据。适合用于自然语言处理任务的开发和实现,特别是对于需要使用 Python 和 PostgreSQL 数据库的场景。特点是自然语言处理库、Python、PostgreSQL 数据库。
项目地址:https://gitcode.com/gh_mirrors/tra/transformers

·
最近做一个图像相关的项目,需要提取图像特征,在使用Transformers库中的深度神经网络模型提取图像特征的过程中,遇到一些问题,记录一下。
下面是图像特征提取的简化代码及相应的中间输出,使用的是OpenAI的CLIP模型:
import os
import torch
import numpy as np
from PIL import Image
from transformers import AutoProcessor, CLIPModel
device = torch.device('cuda' if torch.cuda.is_available() else "cpu")
processor = AutoProcessor.from_pretrained('openai/clip-vit-base-patch32')
model = CLIPModel.from_pretrained('openai/clip-vit-base-patch32').to(device)
img_dir = '/dir/to/img'
img_paths = [os.path.join(img_dir, filename) for filename in os.listdir(img_dir)]
# ['/dir/to/img/a.tif', '/dir/to/img/b.jpg', '/dir/to/img/c.jpg', '/dir/to/img/d.tif']
pilimgs = [Image.open(img) for img in img_paths]
# [<PIL.TiffImagePlugin.TiffImageFile image mode=CMYK size=3008x2000>, <PIL.JpegImagePlugin.JpegImageFile image mode=RGB size=3702x2592>, <PIL.JpegImagePlugin.JpegImageFile image mode=RGB size=3024x1084>, <PIL.TiffImagePlugin.TiffImageFile image mode=CMYK size=3872x2592>]
nparrs = [np.array(img) for img in pilimgs]
# [arr.shape for arr in nparrs]
# [(2000, 3008, 4), (2592, 3702, 3), (1084, 3024, 3), (2592, 3872, 4)]
with torch.no_grad():
inputs = processor(images=nparrs, return_tensors='pt')
image_feature = model.get_image_features(**inputs)
通过中间输出可以看到,使用PIL.Image
读取图片得到的对象的mode
属性是不一样的,tif图片对应的mode
为CMYK,jpg图片对应的mode
为RGB,将这些对象转化为ndarray
后,能够看到tif图片的通道数为4,而jpg图片的通道数为3,图片数据的这些差异会导致使用模型批量提取图片特征时出现问题,而且输入的数据类型和输入图片的顺序不同,出现的问题也不一样。
不同的模型输入及对应的结果
- 直接输入
PIL.Image.Image
对象列表(如下所示),不会报错
with torch.no_grad():
inputs = processor(images=pilimgs, return_tensors='pt')
image_feature = model.get_image_features(**inputs)
直接传入PIL.Image.Image
对象列表,无论列表中是相同mode的图片对象还是不同mode的图片对象,模型都能正确处理,不会报错。但是实际情况下,一般不会直接传入PIL.Image.Image
列表,因为通常需要处理大量图片,在这种情况下,大量使用Image.open而没有进行正确的后续处理很容易造成内存泄漏。可以配合with
语句将图片转化为ndarray
:
with open(img_path, 'rb') as f:
img = Image.open(f)
arr = np.array(img)
- 输入
np.ndarray
列表,列表中含有通道数为4的图片且第一个元素的通道数为4或列表中图片的通道数都为4
出现错误ValueError: Unable to infer channel dimension format
- 输入
np.ndarray
列表,列表中图片的通道数不同且第一个元素的通道数为3
出现错误ValueError: mean must have 4 elements if it is an iterable, got 3
- 输入
np.ndarray
列表,列表中图片的通道数都为3,成功执行
可以看到,当传入给模型的数据类型为np.ndarray
(或torch.Tensor
)时,存在通道数为4(mode
为CMYK或其他)的图片都会导致模型处理出现异常,因此需要将这些图片转化为RGB模式,也就是通道数为3:
with open(img_path, 'rb') as f:
img = Image.open(f)
if img.mode != 'RGB':
img = img.convert('RGB')
arr = np.array(img)
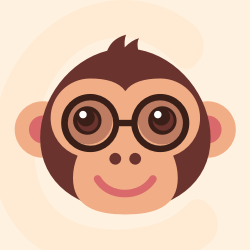



huggingface/transformers: 是一个基于 Python 的自然语言处理库,它使用了 PostgreSQL 数据库存储数据。适合用于自然语言处理任务的开发和实现,特别是对于需要使用 Python 和 PostgreSQL 数据库的场景。特点是自然语言处理库、Python、PostgreSQL 数据库。
最近提交(Master分支:2 个月前 )
6ba31a8a
* bookmark
* Bookmark
* Bookmark
* Actually implement
* Pass in kwarg explicitly
* Adjust for if we do or don't have labels
* Bookmark fix for od
* bookmark
* Fin
* closer
* Negate accelerate grad accum div
* Fixup not training long enough
* Add in compute_loss to take full model output
* Document
* compute_loss -> compute_loss_fn
* Add a test
* Refactor
* Refactor
* Uncomment tests
* Update tests/trainer/test_trainer.py
Co-authored-by: Daniel Han <danielhanchen@gmail.com>
---------
Co-authored-by: Daniel Han <danielhanchen@gmail.com> 11 小时前
7a06d07e
* Support Llama 3.2 conversion (text models)
Co-authored-by: Omar Sanseviero <osanseviero@gmail.com>
* Fix rope factor
* Update chat template
Initialize from a well-known template.
The guidance is that the changes should be applied to 3.1 models as
well.
* Remove import
* Support Llama Guard 3 conversion
* Tokenizer details
* Fix eos added token in base models
* Fix generation config for base models
* Specify revision for known tokenizers
* Style
* Reuse chat templates for older models
* Improve error when converting tokenizer < Llama 3
---------
Co-authored-by: Omar Sanseviero <osanseviero@gmail.com> 11 小时前
更多推荐
所有评论(0)