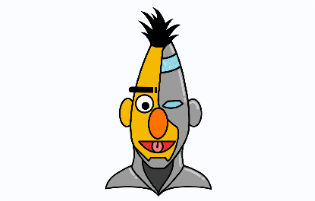
【adapter-transformers】:Installation & QuickStart(一、安装与快速启动)
transformers
huggingface/transformers: 是一个基于 Python 的自然语言处理库,它使用了 PostgreSQL 数据库存储数据。适合用于自然语言处理任务的开发和实现,特别是对于需要使用 Python 和 PostgreSQL 数据库的场景。特点是自然语言处理库、Python、PostgreSQL 数据库。
项目地址:https://gitcode.com/gh_mirrors/tra/transformers

·
【要求】:
adapter-transformers是Huggingface的transformers库的直接替代品。它目前支持Python 3.8+和PyTorch 1.12.1+。因此必须先安装PyTorch。
一、安装(使用pip)
pip install adapter-transformers
二、快速启动(使用训预练的适配器进行推理)
下面的例子展示了如何使用带有适配器的预训练的Transformer模型。我们的目标是预测给定句子的情感。
我们在这个例子中使用BERT,所以我们首先使用BertAdapterModel类从HuggingFace的模型中心加载一个预训练的BertTokenizer来编码输入句子和一个预训练的BERT -base uncase检查点:
import os
import torch
from transformers import BertTokenizer
from transformers.adapters import BertAdapterModel, AutoAdapterModel
# Load pre-trained BERT tokenizer from HuggingFace
tokenizer = BertTokenizer.from_pretrained('bert-base-uncased')
# An input sentence
sentence = "It's also, clearly, great fun."
# Tokenize the input sentence and create a PyTorch input tensor
input_data = tokenizer(sentence, return_tensors="pt")
# Load pre-trained BERT model from HuggingFace Hub
# The `BertAdapterModel` class is specifically designed for working with adapters
# It can be used with different prediction heads
model = BertAdapterModel.from_pretrained('bert-base-uncased')
加载模型后,我们现在添加一个预训练的任务适配器,它对AdapterHub中的任务很有用。
在这种情况下,对于情感分类,我们使用在SST-2数据集上训练的适配器。与适配器一起加载的任务预测头( task prediction head)为我们的句子提供了一个类标签:
# Load pre-trained task adapter from Adapter Hub
# This method call will also load a pre-trained classification head for the adapter task
adapter_name = model.load_adapter("sentiment/sst-2@ukp", config='pfeiffer')
# Activate the adapter we just loaded, so that it is used in every forward pass
model.set_active_adapters(adapter_name)
# Predict output tensor
outputs = model(**input_data)
# Retrieve the predicted class label
predicted = torch.argmax(outputs[0]).item()
assert predicted == 1
为了保存我们预先训练的模型和适配器,我们可以像下面这样轻松地存储和重新加载它们:
# For the sake of this demonstration an example path for loading and storing is given below
example_path = os.path.join(os.getcwd(), "adapter-quickstart")
# Save model
model.save_pretrained(example_path)
# Save adapter
model.save_adapter(example_path, adapter_name)
# Load model, similar to HuggingFace's AutoModel class,
# you can also use AutoAdapterModel instead of BertAdapterModel
model = AutoAdapterModel.from_pretrained(example_path)
model.load_adapter(example_path)
最后,如果我们已经完成了适配器的工作,我们可以通过停用和删除适配器来将基本Transformer恢复到其原始形式:
# Deactivate all adapters
model.set_active_adapters(None)
# Delete the added adapter
model.delete_adapter(adapter_name)
原文链接:Installation — adapter-transformers documentation (adapterhub.ml)
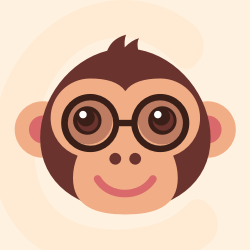



huggingface/transformers: 是一个基于 Python 的自然语言处理库,它使用了 PostgreSQL 数据库存储数据。适合用于自然语言处理任务的开发和实现,特别是对于需要使用 Python 和 PostgreSQL 数据库的场景。特点是自然语言处理库、Python、PostgreSQL 数据库。
最近提交(Master分支:2 个月前 )
33868a05
* [i18n-HI] Translated accelerate page to Hindi
* Update docs/source/hi/accelerate.md
Co-authored-by: K.B.Dharun Krishna <kbdharunkrishna@gmail.com>
* Update docs/source/hi/accelerate.md
Co-authored-by: K.B.Dharun Krishna <kbdharunkrishna@gmail.com>
* Update docs/source/hi/accelerate.md
Co-authored-by: K.B.Dharun Krishna <kbdharunkrishna@gmail.com>
* Update docs/source/hi/accelerate.md
Co-authored-by: K.B.Dharun Krishna <kbdharunkrishna@gmail.com>
---------
Co-authored-by: Kay <kay@Kays-MacBook-Pro.local>
Co-authored-by: K.B.Dharun Krishna <kbdharunkrishna@gmail.com> 6 天前
e2ac16b2
* rework converter
* Update modular_model_converter.py
* Update modular_model_converter.py
* Update modular_model_converter.py
* Update modular_model_converter.py
* cleaning
* cleaning
* finalize imports
* imports
* Update modular_model_converter.py
* Better renaming to avoid visiting same file multiple times
* start converting files
* style
* address most comments
* style
* remove unused stuff in get_needed_imports
* style
* move class dependency functions outside class
* Move main functions outside class
* style
* Update modular_model_converter.py
* rename func
* add augmented dependencies
* Update modular_model_converter.py
* Add types_to_file_type + tweak annotation handling
* Allow assignment dependency mapping + fix regex
* style + update modular examples
* fix modular_roberta example (wrong redefinition of __init__)
* slightly correct order in which dependencies will appear
* style
* review comments
* Performance + better handling of dependencies when they are imported
* style
* Add advanced new classes capabilities
* style
* add forgotten check
* Update modeling_llava_next_video.py
* Add prority list ordering in check_conversion as well
* Update check_modular_conversion.py
* Update configuration_gemma.py 6 天前
更多推荐
所有评论(0)