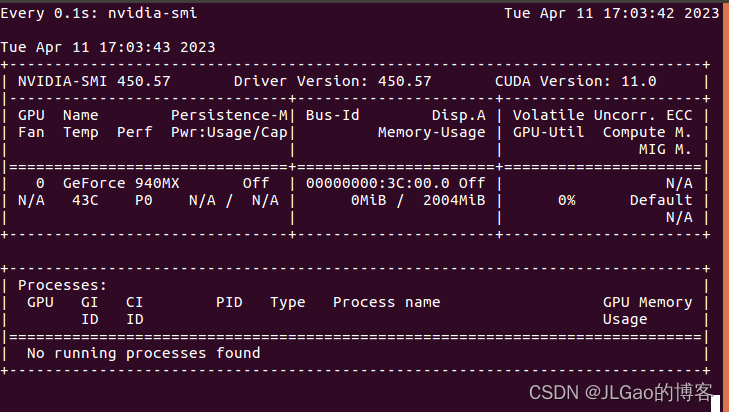
【环境搭建:onnx模型部署】onnxruntime-gpu安装与测试(python)(2)
onnxruntime
microsoft/onnxruntime: 是一个用于运行各种机器学习模型的开源库。适合对机器学习和深度学习有兴趣的人,特别是在开发和部署机器学习模型时需要处理各种不同框架和算子的人。特点是支持多种机器学习框架和算子,包括 TensorFlow、PyTorch、Caffe 等,具有高性能和广泛的兼容性。
项目地址:https://gitcode.com/gh_mirrors/on/onnxruntime

·
ONNX模型部署环境创建
1. onnxruntime 安装
onnx 模型在 CPU 上进行推理,在conda环境中直接使用pip安装即可
pip install onnxruntime
2. onnxruntime-gpu 安装
想要 onnx 模型在 GPU 上加速推理,需要安装 onnxruntime-gpu 。有两种思路:
- 依赖于 本地主机 上已安装的 cuda 和 cudnn 版本
- 不依赖于 本地主机 上已安装的 cuda 和 cudnn 版本
要注意:onnxruntime-gpu, cuda, cudnn三者的版本要对应,否则会报错 或 不能使用GPU推理。
onnxruntime-gpu, cuda, cudnn版本对应关系详见: 官网
2.1 方法一:onnxruntime-gpu依赖于本地主机上cuda和cudnn
- 查看已安装 cuda 和 cudnn 版本
# cuda version
cat /usr/local/cuda/version.txt
# cudnn version
cat /usr/local/cuda/include/cudnn.h | grep CUDNN_MAJOR -A 2
- 根据 onnxruntime-gpu, cuda, cudnn 三者对应关系,安装相应的 onnxruntime-gpu 即可。
## cuda==10.2
## cudnn==8.0.3
## onnxruntime-gpu==1.5.0 or 1.6.0
pip install onnxruntime-gpu==1.6.0
2.2 方法二:onnxruntime-gpu不依赖于本地主机上cuda和cudnn
在 conda 环境中安装,不依赖于 本地主机 上已安装的 cuda 和 cudnn 版本,灵活方便。这里,先说一下已经测试通过的组合:
- python3.6, cudatoolkit10.2.89, cudnn7.6.5, onnxruntime-gpu1.4.0
- python3.8, cudatoolkit11.3.1, cudnn8.2.1, onnxruntime-gpu1.14.1
如果需要其他的版本, 可以根据 onnxruntime-gpu, cuda, cudnn 三者对应关系自行组合测试。
下面,从创建conda环境,到实现在GPU上加速onnx模型推理进行举例。
2.2.1 举例:创建onnxruntime-gpu==1.14.1的conda环境
## 创建conda环境
conda create -n torch python=3.8
## 激活conda环境
source activate torch
conda install pytorch==1.10.0 torchvision==0.11.0 torchaudio==0.10.0 cudatoolkit=11.3 -c pytorch -c conda-forge
conda install cudnn==8.2.1
pip install onnxruntime-gpu==1.14.1
## pip install ... (根据需求,安装其他的包)
2.2.2 举例:实例测试
- 打开终端,输入 watch -n 0.1 nvidia-smi, 实时查看gpu使用情况
- 代码测试,摘取API
import numpy as np
import torch
import onnxruntime
MODEL_FILE = '.model.onnx'
DEVICE_NAME = 'cuda' if torch.cuda.is_available() else 'cpu'
DEVICE_INDEX = 0
DEVICE=f'{DEVICE\_NAME}:{DEVICE\_INDEX}'
# A simple model to calculate addition of two tensors
def model():
class Model(torch.nn.Module):
def \_\_init\_\_(self):
**自我介绍一下,小编13年上海交大毕业,曾经在小公司待过,也去过华为、OPPO等大厂,18年进入阿里一直到现在。**
**深知大多数Python工程师,想要提升技能,往往是自己摸索成长或者是报班学习,但对于培训机构动则几千的学费,着实压力不小。自己不成体系的自学效果低效又漫长,而且极易碰到天花板技术停滞不前!**
**因此收集整理了一份《2024年Python开发全套学习资料》,初衷也很简单,就是希望能够帮助到想自学提升又不知道该从何学起的朋友,同时减轻大家的负担。**
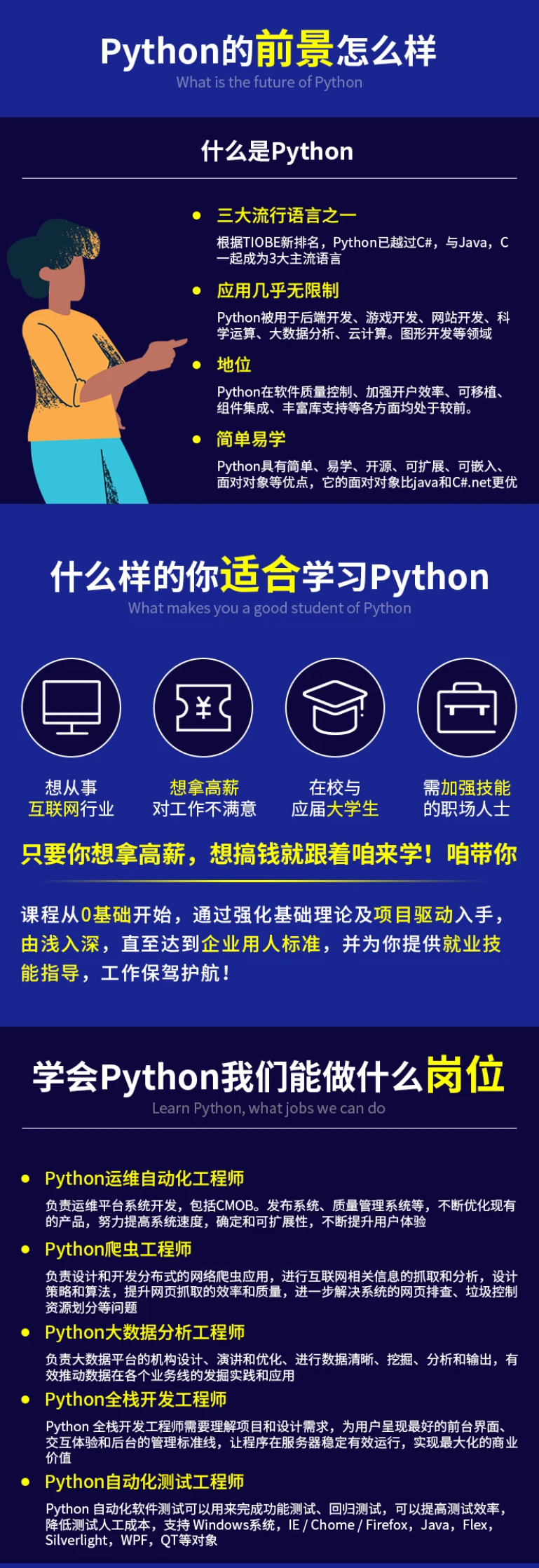
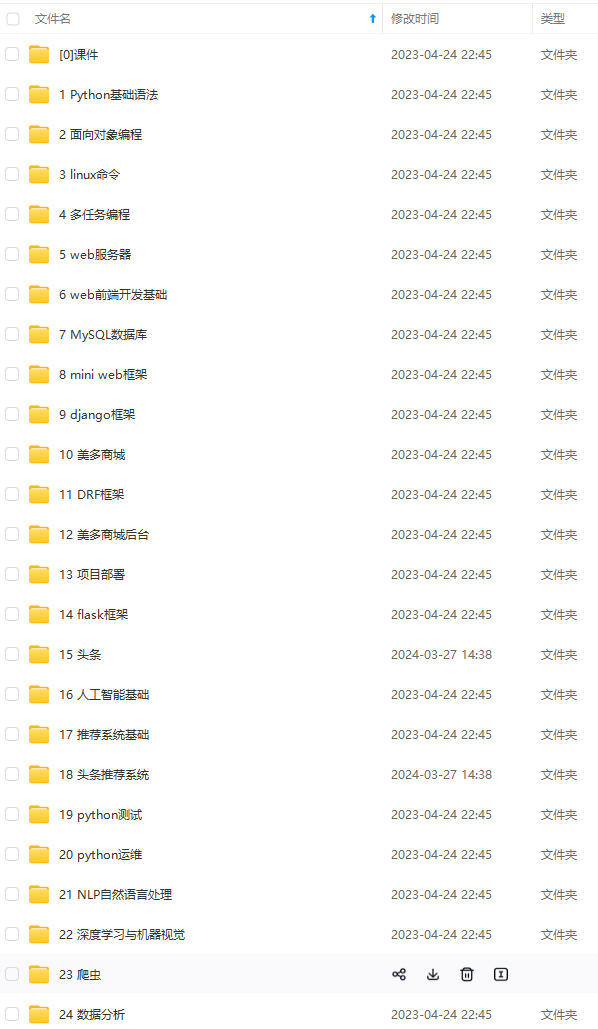
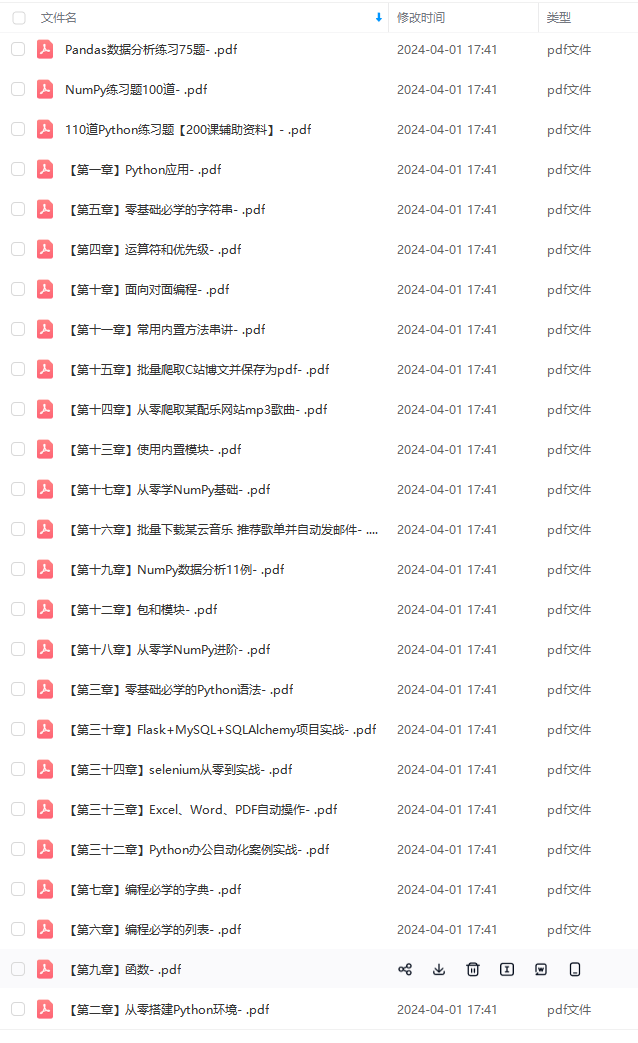
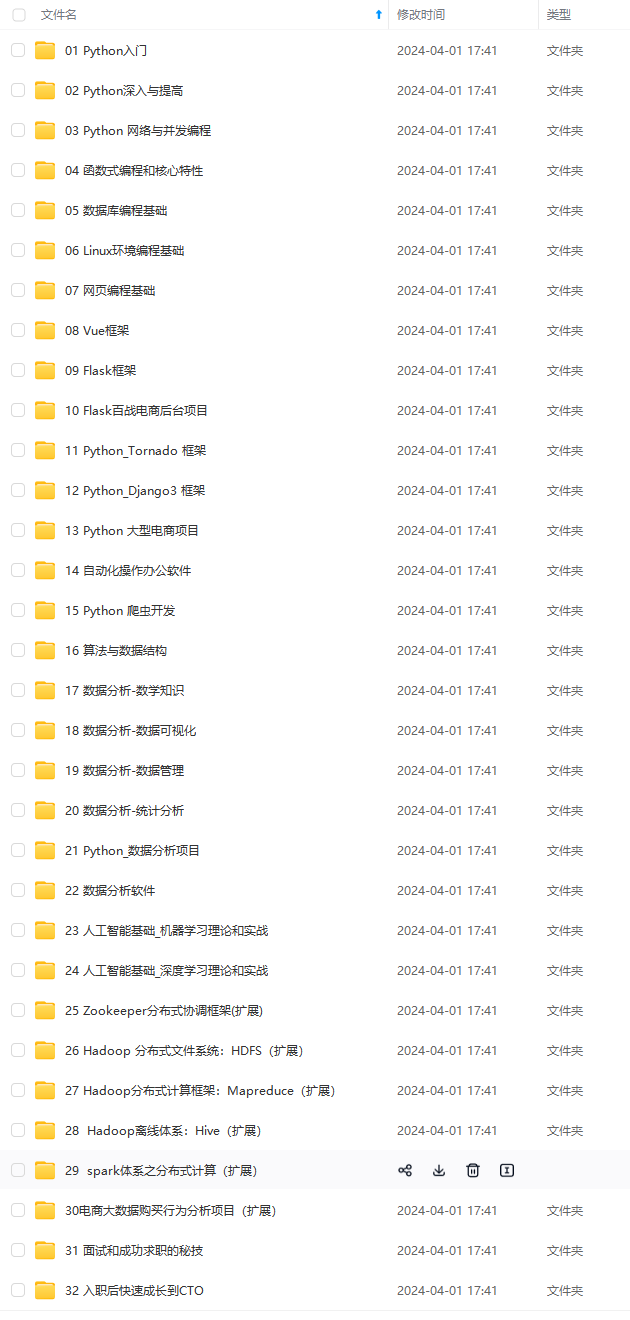
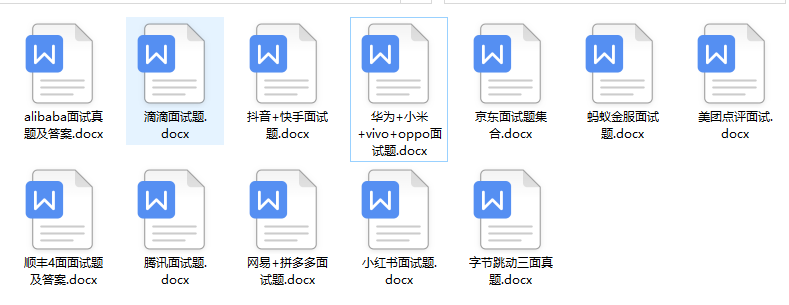
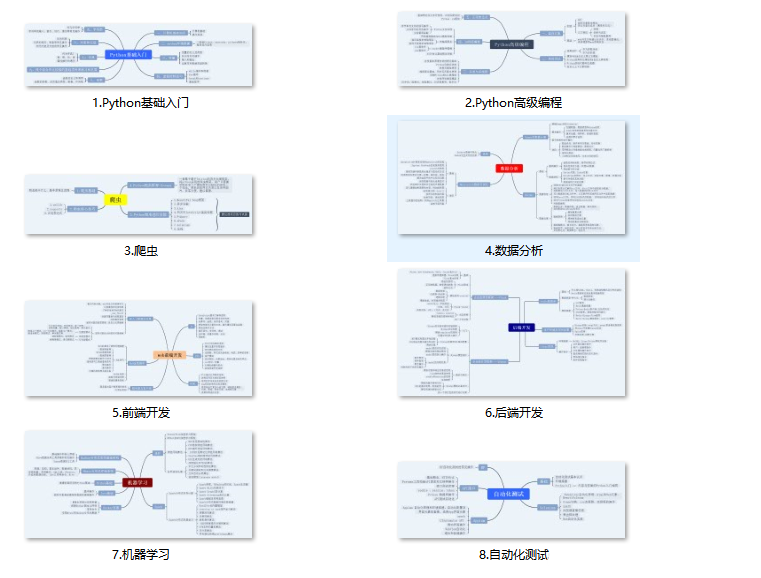
**既有适合小白学习的零基础资料,也有适合3年以上经验的小伙伴深入学习提升的进阶课程,基本涵盖了95%以上前端开发知识点,真正体系化!**
**由于文件比较大,这里只是将部分目录大纲截图出来,每个节点里面都包含大厂面经、学习笔记、源码讲义、实战项目、讲解视频,并且后续会持续更新**
**如果你觉得这些内容对你有帮助,可以扫码获取!!!(备注Python)**
习提升的进阶课程,基本涵盖了95%以上前端开发知识点,真正体系化!**
**由于文件比较大,这里只是将部分目录大纲截图出来,每个节点里面都包含大厂面经、学习笔记、源码讲义、实战项目、讲解视频,并且后续会持续更新**
**如果你觉得这些内容对你有帮助,可以扫码获取!!!(备注Python)**
<img src="https://img-community.csdnimg.cn/images/fd6ebf0d450a4dbea7428752dc7ffd34.jpg" alt="img" style="zoom:50%;" />
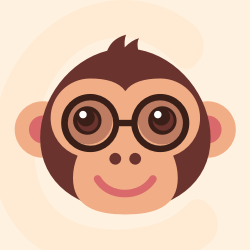



microsoft/onnxruntime: 是一个用于运行各种机器学习模型的开源库。适合对机器学习和深度学习有兴趣的人,特别是在开发和部署机器学习模型时需要处理各种不同框架和算子的人。特点是支持多种机器学习框架和算子,包括 TensorFlow、PyTorch、Caffe 等,具有高性能和广泛的兼容性。
最近提交(Master分支:4 个月前 )
aedb49be
### Description
<!-- Describe your changes. -->
Changed all support tensor type from ir 9 to ir 10.
### Motivation and Context
<!-- - Why is this change required? What problem does it solve?
- If it fixes an open issue, please link to the issue here. -->
- See issue https://github.com/microsoft/onnxruntime/issues/23205
Co-authored-by: Yueqing Zhang <yueqingz@amd.com> 3 小时前
bc91f5c7
### Description
<!-- Describe your changes. -->
For legacy jetson users who use jetpack 5.x, the latest TRT version is
8.5.
Add version check to newer trt features to fix build on jetpack 5.x
(cuda11.8+gcc11 are required)
### Motivation and Context
<!-- - Why is this change required? What problem does it solve?
- If it fixes an open issue, please link to the issue here. --> 15 小时前
更多推荐
所有评论(0)