OpenMMlab导出DETR模型并用onnxruntime和tensorrt推理
onnxruntime
microsoft/onnxruntime: 是一个用于运行各种机器学习模型的开源库。适合对机器学习和深度学习有兴趣的人,特别是在开发和部署机器学习模型时需要处理各种不同框架和算子的人。特点是支持多种机器学习框架和算子,包括 TensorFlow、PyTorch、Caffe 等,具有高性能和广泛的兼容性。
项目地址:https://gitcode.com/gh_mirrors/on/onnxruntime

·
onnxruntime推理
使用mmdeploy导出onnx模型:
from mmdeploy.apis import torch2onnx
from mmdeploy.backend.sdk.export_info import export2SDK
img = 'demo.JPEG'
work_dir = './work_dir/onnx/detr'
save_file = './end2end.onnx'
deploy_cfg = 'mmdeploy/configs/mmdet/detection/detection_onnxruntime_dynamic.py'
model_cfg = 'mmdetection/configs/detr/detr_r50_8xb2-150e_coco.py'
model_checkpoint = 'checkpoints/detr_r50_8xb2-150e_coco_20221023_153551-436d03e8.pth'
device = 'cpu'
# 1. convert model to onnx
torch2onnx(img, work_dir, save_file, deploy_cfg, model_cfg, model_checkpoint, device)
# 2. extract pipeline info for sdk use (dump-info)
export2SDK(deploy_cfg, model_cfg, work_dir, pth=model_checkpoint, device=device)
onnx模型过于复杂无法通过netron可视化(强行打开会巨卡),因此通过onnx的python包来解析onnx模型,只需确定模型的输入输出即可:
import onnx
model = onnx.load("./work_dir/onnx/detr/end2end.onnx")
print(model.graph.input)
print(model.graph.output)
打印如下:
[name: "input"
type {
tensor_type {
elem_type: 1
shape {
dim {
dim_param: "batch"
}
dim {
dim_value: 3
}
dim {
dim_param: "height"
}
dim {
dim_param: "width"
}
}
}
}
]
[name: "dets"
type {
tensor_type {
elem_type: 1
shape {
dim {
dim_param: "batch"
}
dim {
dim_param: "num_dets"
}
dim {
dim_value: 5
}
}
}
}
, name: "labels"
type {
tensor_type {
elem_type: 7
shape {
dim {
dim_param: "batch"
}
dim {
dim_param: "num_dets"
}
}
}
}
]
手动编写onnxruntime推理脚本:
import cv2
import numpy as np
import onnxruntime
class_names = ['person', 'bicycle', 'car', 'motorcycle', 'airplane', 'bus', 'train', 'truck', 'boat', 'traffic light',
'fire hydrant', 'stop sign', 'parking meter', 'bench', 'bird', 'cat', 'dog', 'horse', 'sheep', 'cow',
'elephant', 'bear', 'zebra', 'giraffe', 'backpack', 'umbrella', 'handbag', 'tie', 'suitcase', 'frisbee',
'skis', 'snowboard', 'sports ball', 'kite', 'baseball bat', 'baseball glove', 'skateboard', 'surfboard',
'tennis racket', 'bottle', 'wine glass', 'cup', 'fork', 'knife', 'spoon', 'bowl', 'banana', 'apple',
'sandwich', 'orange', 'broccoli', 'carrot', 'hot dog', 'pizza', 'donut', 'cake', 'chair', 'couch',
'potted plant', 'bed', 'dining table', 'toilet', 'tv', 'laptop', 'mouse', 'remote', 'keyboard', 'cell phone',
'microwave', 'oven', 'toaster', 'sink', 'refrigerator', 'book', 'clock', 'vase', 'scissors', 'teddy bear',
'hair drier', 'toothbrush'] #coco80类别
input_shape = (800, 1344)
confidence_threshold = 0.2
def filter_box(outputs): #删除置信度小于confidence_threshold的BOX
flag = outputs[0][..., 4] > confidence_threshold
boxes = outputs[0][flag]
class_ids = outputs[1][flag].reshape(-1, 1)
output = np.concatenate((boxes, class_ids), axis=1)
return output
def letterbox(im, new_shape=(416, 416), color=(114, 114, 114)):
# Resize and pad image while meeting stride-multiple constraints
shape = im.shape[:2] # current shape [height, width]
# Scale ratio (new / old)
r = min(new_shape[0] / shape[0], new_shape[1] / shape[1])
# Compute padding
new_unpad = int(round(shape[1] * r)), int(round(shape[0] * r))
dw, dh = (new_shape[1] - new_unpad[0])/2, (new_shape[0] - new_unpad[1])/2 # wh padding
top, bottom = int(round(dh - 0.1)), int(round(dh + 0.1))
left, right = int(round(dw - 0.1)), int(round(dw + 0.1))
if shape[::-1] != new_unpad: # resize
im = cv2.resize(im, new_unpad, interpolation=cv2.INTER_LINEAR)
im = cv2.copyMakeBorder(im, top, bottom, left, right, cv2.BORDER_CONSTANT, value=color) # add border
return im
def scale_boxes(input_shape, boxes, shape):
# Rescale boxes (xyxy) from input_shape to shape
gain = min(input_shape[0] / shape[0], input_shape[1] / shape[1]) # gain = old / new
pad = (input_shape[1] - shape[1] * gain) / 2, (input_shape[0] - shape[0] * gain) / 2 # wh padding
boxes[..., [0, 2]] -= pad[0] # x padding
boxes[..., [1, 3]] -= pad[1] # y padding
boxes[..., :4] /= gain
boxes[..., [0, 2]] = boxes[..., [0, 2]].clip(0, shape[1]) # x1, x2
boxes[..., [1, 3]] = boxes[..., [1, 3]].clip(0, shape[0]) # y1, y2
return boxes
def draw(image, box_data):
box_data = scale_boxes(input_shape, box_data, image.shape)
boxes = box_data[...,:4].astype(np.int32)
scores = box_data[...,4]
classes = box_data[...,5].astype(np.int32)
for box, score, cl in zip(boxes, scores, classes):
top, left, right, bottom = box
cv2.rectangle(image, (top, left), (right, bottom), (255, 0, 0), 1)
cv2.putText(image, '{0} {1:.2f}'.format(class_names[cl], score), (top, left), cv2.FONT_HERSHEY_SIMPLEX, 0.6, (0, 0, 255), 1)
if __name__=="__main__":
image = cv2.imread('bus.jpg')
input = letterbox(image, input_shape)
input = input[:, :, ::-1].transpose(2, 0, 1).astype(dtype=np.float32) #BGR2RGB和HWC2CHW
input[0,:] = (input[0,:] - 123.675) / 58.395
input[1,:] = (input[1,:] - 116.28) / 57.12
input[2,:] = (input[2,:] - 103.53) / 57.375
input = np.expand_dims(input, axis=0)
onnx_session = onnxruntime.InferenceSession('../work_dir/onnx/detr/end2end.onnx', providers=['CPUExecutionProvider'])
input_name = []
for node in onnx_session.get_inputs():
input_name.append(node.name)
output_name = []
for node in onnx_session.get_outputs():
output_name.append(node.name)
inputs = {}
for name in input_name:
inputs[name] = input
outputs = onnx_session.run(None, inputs)
boxes = filter_box(outputs)
draw(image, boxes)
cv2.imwrite('result.jpg', image)
使用mmdeploy的推理接口:
from mmdeploy.apis import inference_model
model_cfg = 'mmdetection/configs/detr/detr_r50_8xb2-150e_coco.py'
deploy_cfg = 'mmdeploy/configs/mmdet/detection/detection_onnxruntime_dynamic.py'
img = 'bus.jpg'
backend_files = ['work_dir/onnx/detr/end2end.onnx']
device = 'cpu'
result = inference_model(model_cfg, deploy_cfg, backend_files, img, device)
print(result)
或者
from mmdeploy_runtime import Detector
import cv2
# 读取图片
img = cv2.imread('bus.jpg')
# 创建检测器
detector = Detector(model_path='work_dir/onnx/detr', device_name='cpu')
# 执行推理
bboxes, labels, _ = detector(img)
# 使用阈值过滤推理结果,并绘制到原图中
indices = [i for i in range(len(bboxes))]
for index, bbox, label_id in zip(indices, bboxes, labels):
[left, top, right, bottom], score = bbox[0:4].astype(int), bbox[4]
if score < 0.3:
continue
cv2.rectangle(img, (left, top), (right, bottom), (0, 255, 0))
cv2.imwrite('output_detection.png', img)
tensorrt推理
使用mmdeploy导出engine模型:
from mmdeploy.apis import torch2onnx
from mmdeploy.backend.tensorrt.onnx2tensorrt import onnx2tensorrt
from mmdeploy.backend.sdk.export_info import export2SDK
import os
img = 'bus.jpg'
work_dir = './work_dir/trt/detr'
save_file = './end2end.onnx'
deploy_cfg = 'mmdeploy/configs/mmdet/detection/detection_tensorrt_static-800x1344.py'
model_cfg = 'mmdetection/configs/detr/detr_r50_8xb2-150e_coco.py'
model_checkpoint = 'checkpoints/detr_r50_8xb2-150e_coco_20221023_153551-436d03e8.pth'
device = 'cuda'
torch2onnx(img, work_dir, save_file, deploy_cfg, model_cfg, model_checkpoint, device)
# 2. convert IR to tensorrt
onnx_model = os.path.join(work_dir, save_file)
save_file = 'end2end.engine'
model_id = 0
device = 'cuda'
onnx2tensorrt(work_dir, save_file, model_id, deploy_cfg, onnx_model, device)
# 3. extract pipeline info for sdk use (dump-info)
export2SDK(deploy_cfg, model_cfg, work_dir, pth=model_checkpoint, device=device)
手动编写tensorrt推理脚本:
import cv2
import numpy as np
import tensorrt as trt
import pycuda.autoinit
import pycuda.driver as cuda
class_names = ['person', 'bicycle', 'car', 'motorcycle', 'airplane', 'bus', 'train', 'truck', 'boat', 'traffic light',
'fire hydrant', 'stop sign', 'parking meter', 'bench', 'bird', 'cat', 'dog', 'horse', 'sheep', 'cow',
'elephant', 'bear', 'zebra', 'giraffe', 'backpack', 'umbrella', 'handbag', 'tie', 'suitcase', 'frisbee',
'skis', 'snowboard', 'sports ball', 'kite', 'baseball bat', 'baseball glove', 'skateboard', 'surfboard',
'tennis racket', 'bottle', 'wine glass', 'cup', 'fork', 'knife', 'spoon', 'bowl', 'banana', 'apple',
'sandwich', 'orange', 'broccoli', 'carrot', 'hot dog', 'pizza', 'donut', 'cake', 'chair', 'couch',
'potted plant', 'bed', 'dining table', 'toilet', 'tv', 'laptop', 'mouse', 'remote', 'keyboard', 'cell phone',
'microwave', 'oven', 'toaster', 'sink', 'refrigerator', 'book', 'clock', 'vase', 'scissors', 'teddy bear',
'hair drier', 'toothbrush'] #coco80类别
input_shape = (800, 1344)
score_threshold = 0.2
nms_threshold = 0.5
confidence_threshold = 0.2
def filter_box(outputs): #删除置信度小于confidence_threshold的BOX
flag = outputs[0][..., 4] > confidence_threshold
boxes = outputs[0][flag]
class_ids = outputs[1][flag].reshape(-1, 1)
output = np.concatenate((boxes, class_ids), axis=1)
return output
def letterbox(im, new_shape=(416, 416), color=(114, 114, 114)):
# Resize and pad image while meeting stride-multiple constraints
shape = im.shape[:2] # current shape [height, width]
# Scale ratio (new / old)
r = min(new_shape[0] / shape[0], new_shape[1] / shape[1])
# Compute padding
new_unpad = int(round(shape[1] * r)), int(round(shape[0] * r))
dw, dh = (new_shape[1] - new_unpad[0])/2, (new_shape[0] - new_unpad[1])/2 # wh padding
top, bottom = int(round(dh - 0.1)), int(round(dh + 0.1))
left, right = int(round(dw - 0.1)), int(round(dw + 0.1))
if shape[::-1] != new_unpad: # resize
im = cv2.resize(im, new_unpad, interpolation=cv2.INTER_LINEAR)
im = cv2.copyMakeBorder(im, top, bottom, left, right, cv2.BORDER_CONSTANT, value=color) # add border
return im
def scale_boxes(boxes, shape):
# Rescale boxes (xyxy) from input_shape to shape
gain = min(input_shape[0] / shape[0], input_shape[1] / shape[1]) # gain = old / new
pad = (input_shape[1] - shape[1] * gain) / 2, (input_shape[0] - shape[0] * gain) / 2 # wh padding
boxes[..., [0, 2]] -= pad[0] # x padding
boxes[..., [1, 3]] -= pad[1] # y padding
boxes[..., :4] /= gain
boxes[..., [0, 2]] = boxes[..., [0, 2]].clip(0, shape[1]) # x1, x2
boxes[..., [1, 3]] = boxes[..., [1, 3]].clip(0, shape[0]) # y1, y2
return boxes
def draw(image, box_data):
box_data = scale_boxes(box_data, image.shape)
boxes = box_data[...,:4].astype(np.int32)
scores = box_data[...,4]
classes = box_data[...,5].astype(np.int32)
for box, score, cl in zip(boxes, scores, classes):
top, left, right, bottom = box
cv2.rectangle(image, (top, left), (right, bottom), (255, 0, 0), 1)
cv2.putText(image, '{0} {1:.2f}'.format(class_names[cl], score), (top, left), cv2.FONT_HERSHEY_SIMPLEX, 0.6, (0, 0, 255), 1)
if __name__=="__main__":
logger = trt.Logger(trt.Logger.WARNING)
with open("../work_dir/trt/detr/end2end.engine", "rb") as f, trt.Runtime(logger) as runtime:
engine = runtime.deserialize_cuda_engine(f.read())
context = engine.create_execution_context()
h_input = cuda.pagelocked_empty(trt.volume(context.get_binding_shape(0)), dtype=np.float32)
h_output0 = cuda.pagelocked_empty(trt.volume(context.get_binding_shape(1)), dtype=np.int32)
h_output1 = cuda.pagelocked_empty(trt.volume(context.get_binding_shape(2)), dtype=np.float32)
d_input = cuda.mem_alloc(h_input.nbytes)
d_output0 = cuda.mem_alloc(h_output0.nbytes)
d_output1 = cuda.mem_alloc(h_output1.nbytes)
stream = cuda.Stream()
image = cv2.imread('bus.jpg')
input = letterbox(image, input_shape)
input = input[:, :, ::-1].transpose(2, 0, 1).astype(dtype=np.float32) #BGR2RGB和HWC2CHW
input[0,:] = (input[0,:] - 123.675) / 58.395
input[1,:] = (input[1,:] - 116.28) / 57.12
input[2,:] = (input[2,:] - 103.53) / 57.375
input = np.expand_dims(input, axis=0)
np.copyto(h_input, input.ravel())
with engine.create_execution_context() as context:
cuda.memcpy_htod_async(d_input, h_input, stream)
context.execute_async_v2(bindings=[int(d_input), int(d_output0), int(d_output1)], stream_handle=stream.handle)
cuda.memcpy_dtoh_async(h_output0, d_output0, stream)
cuda.memcpy_dtoh_async(h_output1, d_output1, stream)
stream.synchronize()
h_output = []
h_output.append(h_output1.reshape(1, 100, 5))
h_output.append(h_output0.reshape(1, 100))
boxes = filter_box(h_output)
draw(image, boxes)
cv2.imwrite('result.jpg', image)
使用mmdeploy的推理接口:
from mmdeploy.apis import inference_model
model_cfg = 'mmdetection/configs/detr/detr_r50_8xb2-150e_coco.py'
deploy_cfg = 'mmdeploy/configs/mmdet/detection/detection_tensorrt_static-800x1344.py'
img = 'bus.jpg'
backend_files = ['work_dir/trt/detr/end2end.engine']
device = 'cuda'
result = inference_model(model_cfg, deploy_cfg, backend_files, img, device)
print(result)
或者
from mmdeploy_runtime import Detector
import cv2
# 读取图片
img = cv2.imread('mmdetection/demo/demo.jpg')
# 创建检测器
detector = Detector(model_path='work_dir/trt/detr', device_name='cuda')
# 执行推理
bboxes, labels, _ = detector(img)
# 使用阈值过滤推理结果,并绘制到原图中
indices = [i for i in range(len(bboxes))]
for index, bbox, label_id in zip(indices, bboxes, labels):
[left, top, right, bottom], score = bbox[0:4].astype(int), bbox[4]
if score < 0.3:
continue
cv2.rectangle(img, (left, top), (right, bottom), (0, 255, 0))
cv2.imwrite('output_detection.png', img)
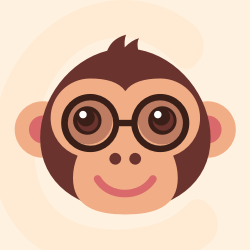



microsoft/onnxruntime: 是一个用于运行各种机器学习模型的开源库。适合对机器学习和深度学习有兴趣的人,特别是在开发和部署机器学习模型时需要处理各种不同框架和算子的人。特点是支持多种机器学习框架和算子,包括 TensorFlow、PyTorch、Caffe 等,具有高性能和广泛的兼容性。
最近提交(Master分支:1 个月前 )
1bda91fc
### Description
Fixes the problem of running into failure when GPU inputs shuffled
between iterations. 9 天前
52a8c1ca
### Description
Enables using the MLTensor to pass data between models.
### Motivation and Context
Using MLTensor instead of ArrayBuffers reduces the number of copies
between the CPU and devices as well as the renderer and GPU process in
Chromium. 10 天前
更多推荐
所有评论(0)