yolov5官方代码解读——前向传播

yolov5前向传播代码解读
前言
本笔记以yolov5 4.0版本为例
在yolov5/models/yolo.py中,我们可以运行main代码块查看yolov5s的结构。
from n params module arguments
0 -1 1 3520 models.common.Focus [3, 32, 3]
1 -1 1 18560 models.common.Conv [32, 64, 3, 2]
2 -1 1 18816 models.common.C3 [64, 64, 1]
3 -1 1 73984 models.common.Conv [64, 128, 3, 2]
4 -1 1 156928 models.common.C3 [128, 128, 3]
5 -1 1 295424 models.common.Conv [128, 256, 3, 2]
6 -1 1 625152 models.common.C3 [256, 256, 3]
7 -1 1 1180672 models.common.Conv [256, 512, 3, 2]
8 -1 1 656896 models.common.SPP [512, 512, [5, 9, 13]]
9 -1 1 1182720 models.common.C3 [512, 512, 1, False]
10 -1 1 131584 models.common.Conv [512, 256, 1, 1]
11 -1 1 0 torch.nn.modules.upsampling.Upsample [None, 2, 'nearest']
12 [-1, 6] 1 0 models.common.Concat [1]
13 -1 1 361984 models.common.C3 [512, 256, 1, False]
14 -1 1 33024 models.common.Conv [256, 128, 1, 1]
15 -1 1 0 torch.nn.modules.upsampling.Upsample [None, 2, 'nearest']
16 [-1, 4] 1 0 models.common.Concat [1]
17 -1 1 90880 models.common.C3 [256, 128, 1, False]
18 -1 1 147712 models.common.Conv [128, 128, 3, 2]
19 [-1, 14] 1 0 models.common.Concat [1]
20 -1 1 296448 models.common.C3 [256, 256, 1, False]
21 -1 1 590336 models.common.Conv [256, 256, 3, 2]
22 [-1, 10] 1 0 models.common.Concat [1]
23 -1 1 1182720 models.common.C3 [512, 512, 1, False]
24 [17, 20, 23] 1 229245 Detect [80, [[10, 13, 16, 30, 33, 23], [30, 61, 62, 45, 59, 119], [116, 90, 156, 198, 373, 326]], [128, 256, 512]]
Model Summary: 283 layers, 7276605 parameters, 7276605 gradients, 17.1 GFLOPS
在main代码块下面添加打印语句,手动打印yolov5的模型结构
print(model)
这时,我们就会惊讶的发现,yolov5的模型居然是只有一个nn.Sequential的顺序结构,不是说好的是下面这种复杂的网络结构吗??
这就是yolov5官方编写前向传播过程代码的巧妙之处了
yolov5的网络结构
yolov5/models/yolov5s.yaml
# parameters
nc: 80 # number of classes
depth_multiple: 0.33 # model depth multiple
width_multiple: 0.50 # layer channel multiple
# anchors
anchors:
- [10,13, 16,30, 33,23] # P3/8
- [30,61, 62,45, 59,119] # P4/16
- [116,90, 156,198, 373,326] # P5/32
# YOLOv5 backbone
backbone:
# [from, number, module, args]
[[-1, 1, Focus, [64, 3]], # 0-P1/2
[-1, 1, Conv, [128, 3, 2]], # 1-P2/4
[-1, 3, C3, [128]],
[-1, 1, Conv, [256, 3, 2]], # 3-P3/8
[-1, 9, C3, [256]],
[-1, 1, Conv, [512, 3, 2]], # 5-P4/16
[-1, 9, C3, [512]],
[-1, 1, Conv, [1024, 3, 2]], # 7-P5/32
[-1, 1, SPP, [1024, [5, 9, 13]]],
[-1, 3, C3, [1024, False]], # 9
]
# YOLOv5 head
head:
[[-1, 1, Conv, [512, 1, 1]],
[-1, 1, nn.Upsample, [None, 2, 'nearest']],
[[-1, 6], 1, Concat, [1]], # cat backbone P4
[-1, 3, C3, [512, False]], # 13
[-1, 1, Conv, [256, 1, 1]],
[-1, 1, nn.Upsample, [None, 2, 'nearest']],
[[-1, 4], 1, Concat, [1]], # cat backbone P3
[-1, 3, C3, [256, False]], # 17 (P3/8-small)
[-1, 1, Conv, [256, 3, 2]],
[[-1, 14], 1, Concat, [1]], # cat head P4
[-1, 3, C3, [512, False]], # 20 (P4/16-medium)
[-1, 1, Conv, [512, 3, 2]],
[[-1, 10], 1, Concat, [1]], # cat head P5
[-1, 3, C3, [1024, False]], # 23 (P5/32-large)
[[17, 20, 23], 1, Detect, [nc, anchors]], # Detect(P3, P4, P5)
]
yolov5官方在yolov5s.yaml 定义了yolov5s的网络结构,其中backbone和head定义了每层网络的
[数据来源,网络深度,网络类型,参数]
以[[-1, 6], 1, Concat, [1]], # cat backbone P4
这一行为例:
- 数据来源:表示该层的输入来自哪个层,-1代表上一层,“数字”代表顺序(从0开始)。该例中第一个
[-1, 6]
代表了该层的数据来自上一层和第6层。 - 网络深度
- 网络类型:该例中Concat代表该层为Concat层
- 参数:创建网络对象的参数,该例中第4个
[1]
代表创建Concat对象时,第一个参数dimension为1,下面时Concat层的定义。
class Concat(nn.Module):
# Concatenate a list of tensors along dimension
def __init__(self, dimension=1):
super(Concat, self).__init__()
self.d = dimension
def forward(self, x):
return torch.cat(x, self.d)
yolov5的前向传播代码
生成网络结构
官方在yolov5/models/yolo.py的main方法中定义了生成网络结构的代码。
该代码实际上就是读取yaml文件中的数据,创建对应的网络对象添加到layers列表中,然后创建一个nn.Sequential对象。
def parse_model(d, ch): # model_dict, input_channels(3)
logger.info('\n%3s%18s%3s%10s %-40s%-30s' % ('', 'from', 'n', 'params', 'module', 'arguments'))
anchors, nc, gd, gw = d['anchors'], d['nc'], d['depth_multiple'], d['width_multiple']
na = (len(anchors[0]) // 2) if isinstance(anchors, list) else anchors # number of anchors
no = na * (nc + 5) # number of outputs = anchors * (classes + 5)
layers, save, c2 = [], [], ch[-1] # layers, savelist, ch out
for i, (f, n, m, args) in enumerate(d['backbone'] + d['head']): # from, number, module, args
m = eval(m) if isinstance(m, str) else m # eval strings
for j, a in enumerate(args):
try:
args[j] = eval(a) if isinstance(a, str) else a # eval strings
except:
pass
n = max(round(n * gd), 1) if n > 1 else n # depth gain
if m in [Conv, Bottleneck, SPP, DWConv, MixConv2d, Focus, CrossConv, BottleneckCSP, C3]:
c1, c2 = ch[f], args[0]
args = [c1, c2, *args[1:]]
if m in [BottleneckCSP, C3]:
args.insert(2, n)
n = 1
elif m is nn.BatchNorm2d:
args = [ch[f]]
elif m is Concat:
c2 = sum([ch[x if x < 0 else x + 1] for x in f])
elif m is Detect:
args.append([ch[x + 1] for x in f])
if isinstance(args[1], int): # number of anchors
args[1] = [list(range(args[1] * 2))] * len(f)
elif m is Contract:
c2 = ch[f if f < 0 else f + 1] * args[0] ** 2
elif m is Expand:
c2 = ch[f if f < 0 else f + 1] // args[0] ** 2
else:
c2 = ch[f if f < 0 else f + 1]
m_ = nn.Sequential(*[m(*args) for _ in range(n)]) if n > 1 else m(*args) # module
t = str(m)[8:-2].replace('__main__.', '') # module type
np = sum([x.numel() for x in m_.parameters()]) # number params
m_.i, m_.f, m_.type, m_.np = i, f, t, np # attach index, 'from' index, type, number params
logger.info('%3s%18s%3s%10.0f %-40s%-30s' % (i, f, n, np, t, args)) # print
save.extend(x % i for x in ([f] if isinstance(f, int) else f) if x != -1) # append to savelist
layers.append(m_)
ch.append(c2)
return nn.Sequential(*layers), sorted(save)
其中最关键的就是下面这一句,将我们在yaml文件中定义的每一层的数据来源保存到该层的对象中。
m_.i, m_.f, m_.type, m_.np = i, f, t, np # attach index, 'from' index, type, number params
前向传播
前向传播的关键代码在yolov5/models/yolo.py中,Model(nn.Module)类的forward_once方法
Model(nn.Module).forward_once(self, x, profile=False)
def forward_once(self, x, profile=False):
y, dt = [], [] # outputs
for m in self.model:
if m.f != -1: # if not from previous layer
x = y[m.f] if isinstance(m.f, int) else [x if j == -1 else y[j] for j in m.f] # from earlier layers
if profile:
o = thop.profile(m, inputs=(x,), verbose=False)[0] / 1E9 * 2 if thop else 0 # FLOPS
t = time_synchronized()
for _ in range(10):
_ = m(x)
dt.append((time_synchronized() - t) * 100)
print('%10.1f%10.0f%10.1fms %-40s' % (o, m.np, dt[-1], m.type))
x = m(x) # run
y.append(x if m.i in self.save else None) # save output
if profile:
print('%.1fms total' % sum(dt))
return x
x是输入的数据,y是保存每层输出结果的列表
每次前向传播,程序就遍历self.model中保存的每一层网络,在下面这句代码中
x = y[m.f] if isinstance(m.f, int) else [x if j == -1 else y[j] for j in m.f] # from earlier layers
如果该层的数据来源m.f
是-1,就保留x为上一层的输出,否则,就从y
中取出对应层的输出结果。
之后,进行完该层的前向传播后,就将输出结果添加到y
中
x = m(x) # run
y.append(x if m.i in self.save else None) # save output
注意上面是只有该层索引m.i
在self.save
中时,才会保存结果,否则保存None
。
综上,yolov5官方代码的前向传播过程示意图如下:(注意,每层的输入还包括上一层的输出)
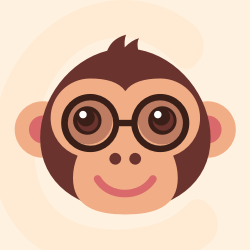



更多推荐
所有评论(0)