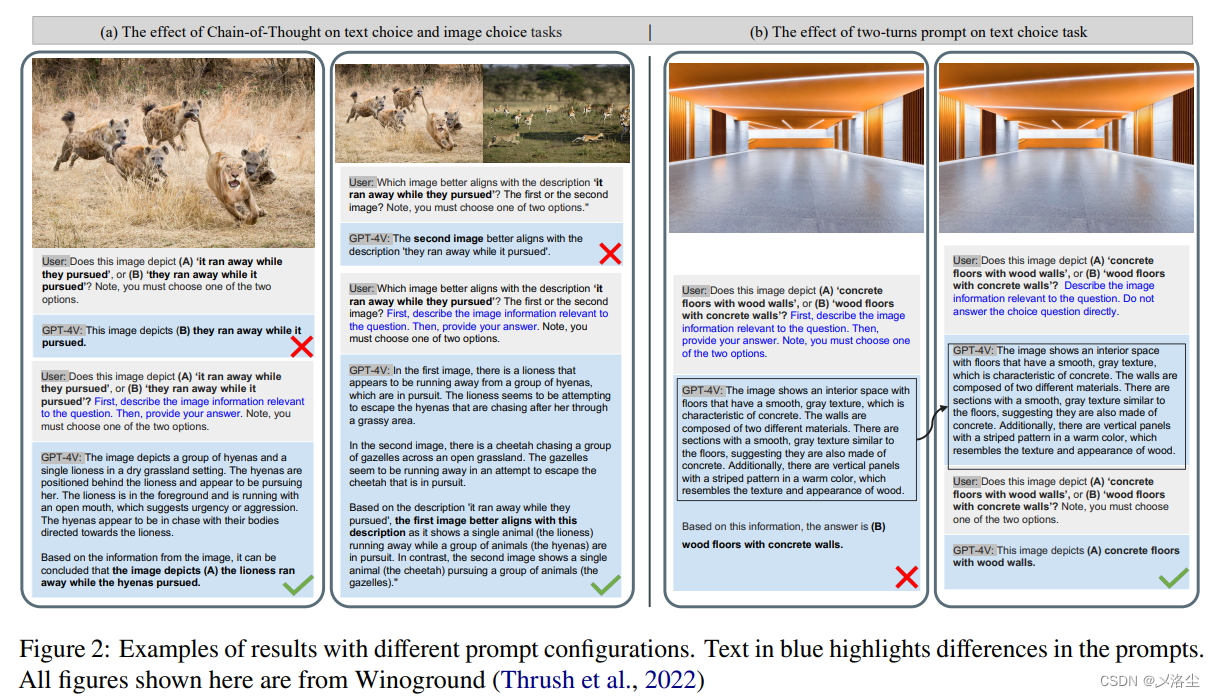
VL 任务 The Role of Chain-of-Thought in Complex Vision-Language Reasoning Task 论文阅读笔记

VL 任务 The Role of Chain-of-Thought in Complex Vision-Language Reasoning Task 论文阅读笔记
写在前面
尝试点新东西,这是一篇关于视觉语言多模态的文章,作者团队很亮眼呀~
- 论文地址:The Role of Chain-of-Thought in Complex Vision-Language Reasoning Task
- 代码地址:原文未提供
- 预计投稿于:某个会议
- Ps:2023 年每周一篇博文阅读笔记,主页 更多干货,欢迎关注呀,期待 6 千粉丝有你的参与呦~
一、Abstract
思维链的效果在语言任务中发挥了重要作用,特别是在需要复杂的感知和推理的视觉-语言任务中。基于人类处理信号的过程,本文提出 “描述后再决定” 的策略。这一策略提升了大概 50% 的性能,为视觉-语言中的推理任务奠定了坚实的基础。
二、引言
大语言模型在很多任务中表现不俗,因此促进了通用式 AI 助手的发展,最典型的就是 GPT-4V。然而在复杂的视觉-语言任务中,GPT-4V 仍落后于人类。 这些任务需要识别层面上的感知,例如定位和分类目标及其属性,以及识别层面上的推理。人类可以无缝地整合这两个阶段,但对于 LLMs 来说,还不太够。
思维链策略,最出名的就是能够将语言任务划分为包含多个中间步的子任务。本文研究的是其能否增强视觉-语言任务,特别是需要复杂推理的。
如上图所示,对于人类识别的研究可以提供一些线索。视觉信息通过双流进行传播,侧流 ‘what pathway’ 涉及目标识别的任务,而背流 ‘where pathway’ 则处理目标的空间位置。此双流将识别解耦为局部处理模块。识别部分,即推理和决策函数,主要通过正面的脑叶实现。基于此,本文设计了特定模块,用于不同阶段的推理。
最新的关于思维链在视觉-语言任务上的尝试是识别任务,以及定性分析。在本文中,分析了 Prompting 策略对复杂的视觉-语言任务的影响,提出的 Description (information-extracting) then Decision (decisionmaking) 策略一致提升了任务的性能。
三、探索性任务
以 Winoground 为一个研究案例,Winoground 同时包含一个数据集和一个特定任务用于评估视觉语言组合式推理:给定两张图像及两条字幕,旨在正确地匹配每条字幕和图像。需要注意的是,所有的字幕都使用相同的单词,但是顺序不同。其难点之处在于需要一个鲁邦的视觉识别能力去鉴别小的或者模糊的目标,以及区分相似目标的属性,同时也需要视觉-语言组合式推理。Winoground 的一些样本如下图所示:
原始的 Winoground 任务由两个实验步骤组成:文本得分和图像得分。前者评估模型从两个给定的字幕和一张图像中选择出正确字幕的能力。后者则需要评估模型从两个可选的图像和单条字幕中选择最合适的图像。Winoground 数据集由 400 对图像-字幕对组成。
Tristan Thrush, Ryan Jiang, Max Bartolo, Amanpreet Singh, Adina Williams, Douwe Kiela, and Candace Ross. 2022. Winoground: Probing vision and language models for visio-linguistic compositionality. In CVPR.
原始的 Winoground 是基于视觉语言模型中的特征相似度进行计算的,例如 CLIP。为评估最近的大规模视觉-语言模型,类似 GPT-4V,于是将 Winoground 塑造为基于选择的视觉问答任务。
给定图像
I
0
I_0
I0 和
I
1
I_1
I1,以及字幕
C
0
C_0
C0 和
C
1
C_1
C1,用于数据点
(
C
0
,
I
0
,
C
1
,
I
1
)
(C_0,I_0,C_1,I_1)
(C0,I0,C1,I1) 的计算如下:
s
(
C
0
,
I
0
,
C
1
,
I
1
)
=
{
1
i
f
f
(
C
0
,
C
1
,
I
0
)
=
C
0
,
a
n
d
f
(
C
0
,
C
1
,
I
1
)
=
C
1
0
o
t
h
e
r
w
i
s
e
\left.s\left(C_0,I_0,C_1,I_1\right)=\left\{\begin{array}{ll}1&\mathrm{~if~}f\left(C_0,C_1,I_0\right)=C_0,\\&\mathrm{~and~}f\left(C_0,C_1,I_1\right)=C_1\\0&\mathrm{~otherwise}\end{array}\right.\right.
s(C0,I0,C1,I1)=⎩
⎨
⎧10 if f(C0,C1,I0)=C0, and f(C0,C1,I1)=C1 otherwise其中
f
(
⋅
)
f(\cdot)
f(⋅) 为大语言模型,旨在根据生成过程提供答案。对于一个正确的数据点,所有的图像必须要与文本描述对齐。
类似的,对于图像选择任务,得分定义为:
s
(
C
0
,
I
0
,
C
1
,
I
1
)
=
{
1
i
f
f
(
I
0
,
I
1
,
C
0
)
=
I
0
,
a
n
d
f
(
I
0
,
I
1
,
C
1
)
=
I
1
0
o
t
h
e
r
w
i
s
e
\left.s\left(C_0,I_0,C_1,I_1\right)=\left\{\begin{array}{ll}1&\quad\mathrm{if~}f\left(I_0,I_1,C_0\right)=I_0,\\&\quad\mathrm{and~}f\left(I_0,I_1,C_1\right)=I_1\\0&\quad\mathrm{otherwise}\end{array}\right.\right.
s(C0,I0,C1,I1)=⎩
⎨
⎧10if f(I0,I1,C0)=I0,and f(I0,I1,C1)=I1otherwise
四、评估
4.1 思维链的作用
定性结果如表 1 所示,定量结果如图 2(a) 所示。接下来展示用于评估 GPT-4V 文本得分和图像得分在有无思维链的 prompt:
- GPT-4V (Text):
[‘image-0’ or ‘image-1’] Does this image present (A) [‘caption-0’] or (B) [‘caption-1’]? Note, you must choose one of the two options. - GPT-4V CoT (Text):
[‘image-0’ or ‘image-1’] Does this image present (A) [‘caption-0’], or (B)
[‘caption-1’]? First, describe the image information relevant to the question. Then, provide your answer. Note you must choose one of the two options. - GPT-4V (Image):
[‘image-0’], [‘image-1’] Which image better aligns with the description [‘caption-0’ or ‘caption-1’]? The first image or the second image? Note you must choose one of two options. - GPT-4V CoT (Image):
[‘image-0’], [‘image-1’] Which image better aligns with the description
[‘caption-0’ or ‘caption-1’]? The first image or the second image? First, describe the image information relevant to the question. Then, provide your answer. Note you must choose one of two options.
一些例子如下图所示:
4.2 两种 Prompt 的效果
如图 (2) b 所示。接下来展示一些 prompt:
- GPT-4 QA:
[‘image description’] Based on this image description, does this image depict (A) [‘caption-0’], or (B) [‘caption-1’]? Note, you must choose one of the two options. - GPT-4 CoT:
[‘image description’] Based on this image description, does this image depict (A)
[‘caption-0’], or (B) [‘caption-1’]? First, analyze the two options, then provide your answer. Note,
you must choose one of the two options. - GPT-4V QA:
[‘image description’] Does this image depict (A) [‘caption-0’], or (B) [‘caption-1’]?
Note, you must choose one of the two options. - GPT-4V CoT:
[‘image description’] Does this image depict (A) [‘caption-0’], or (B) [‘caption-1’]?
First, analyze the two options, then provide your answer. Note, you must choose one of the two options.
结果如下表所示:
五、误差分析
为区分不同属性,那些基于视觉线索的,例如颜色和形状更容易区分。而更抽象的属性,例如尺寸或数量、重量,则需要外部的知识。涉及到 “Series, Pragmatics, Size/Amount, Weight, Object-Centric Spatial, Temporal” 则更为复杂。
六、结论
本研究引入了 “描述后决定” 的策略用于视觉-语言任务。从神经感知角度来说,人类进行识别和推理需要多个步骤,而从模型训练的角度来说,大语言模型在语言任务上会更有效率。通过图像字幕,视觉编码器可以对齐这些语言模型。给定一个视觉-语言任务,“描述后决定” 的方法将任务转化为两个训练好的任务,在不同的模型上都有性能的提升,促进了将来对视觉-语言任务的研究。
写在后面
首次读这类涉及到 GPT 的文章,感觉咋说呢,相比于传统的方法,缺失了一定的叙述手段,就是那种鸡肋似的,“难登大雅之堂” 吧?
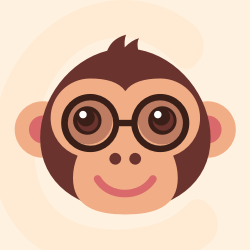



更多推荐
所有评论(0)