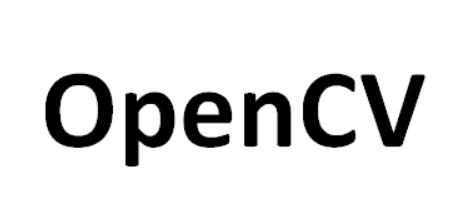
opencv实战---物体尺寸测量

物体尺寸测量的思路是找一个确定尺寸的物体作为参照物,根据已知的计算未知物体尺寸。
如下图所示,绿色的板子尺寸为220*300(单位:毫米),通过程序计算白色纸片的长度。
目录
1、相关库
opencv-python==4.2.0.34
numpy==1.21.6
2、读图+图片预处理
这一步就很常规的处理了。
为了看到图片所有的样子,所以进行resize一下。接下来进行灰度化+高斯模糊+canny边缘检测。然后为了加强边缘进行闭运算。
开运算:先腐蚀后膨胀。用来消除小物体,平滑边界,断开物体之间的粘连。
闭运算:先膨胀后腐蚀。用来填充物体内的小空洞,连接断开的轮廓线。
## 读图
path = '1.jpg'
img = cv2.imread(path)
## 图片预处理
# 由于原图太大,就按比例缩小。比例为0.18
img = cv2.resize(img, (0,0),None,0.18,0.18)
imgGray = cv2.cvtColor(img, cv2.COLOR_BGR2GRAY) # 灰度化
imgBlur = cv2.GaussianBlur(imgGray, (5,5), 1) # 高斯模糊
imgCanny = cv2.Canny(imgBlur,100,100) # 边缘检测
kernel = np.ones((5,5))
imgDial = cv2.dilate(imgCanny,kernel,iterations=3) # 膨胀
imgThre = cv2.erode(imgDial,kernel,iterations=2) # 腐蚀
imgThre结果:
3、寻找轮廓
# 寻找所有的外轮廓
contours, hiearchy = cv2.findContours(imgThre, cv2.RETR_EXTERNAL, cv2.CHAIN_APPROX_SIMPLE)
finalCountours = []
minArea = 1000
filter = 4
# 遍历找到的轮廓
for i in contours:
area = cv2.contourArea(i) # 轮廓的面积
if area > minArea: # 如果大于设置的最小轮廓值,就往下走
peri = cv2.arcLength(i, True) # 封闭轮廓的长度
approx = cv2.approxPolyDP(i, 0.02 * peri, True) # 封闭轮廓曲线拐点坐标
bbox = cv2.boundingRect(approx) # 找到轮廓的最小矩形
if filter > 0: # 需不需要根据拐点个数进行过滤轮廓
if len(approx) == filter: # 拐点个数,面积,拐点坐标,边界框,轮廓
finalCountours.append([len(approx), area, approx, bbox, i])
else:
finalCountours.append([len(approx), area, approx, bbox, i])
# 将轮廓从大到小进行排列
finalCountours = sorted(finalCountours,key=lambda x:x[1] , reverse=True)
if draw: # 是否要画出来轮廓
for con in finalCountours:
cv2.drawContours(img,con[4],-1,(0,0,255),3)
找到图像中的外轮廓
- 函数findContours()常用参数含义:第一个为输入图像,一般是二值图像。CV_RETR_EXTERNAL表示只检测外轮廓。CV_CHAIN_APPROX_SIMPLE表示保留该方向的终点坐标,也就是拐点坐标,例如一个矩形轮廓只需4个点来保存轮廓信息
遍历每个轮廓:
- 求每个轮廓的面积,如果面积大于阈值,就进行下一步(滤掉那些小的不重要的轮廓)
- 函数arcLength():计算轮廓的周长。参数:第一个为图像轮廓点集合;第二个表示轮廓是否封闭的
- 函数approxPolyDP():对图像轮廓进行多边形拟合。参数:第一个为图像轮廓点集合;第二个表示输出的精度,通俗来说,就是原本的曲线与拟合的曲线之间的最大距离;第三个表示轮廓是否封闭的。返回值是拐点坐标
- 函数boundingRect():计算轮廓的垂直边界最小矩形。找到闭合曲线对应的最小矩形
- 是否需要根据拐点个数进行过滤轮廓。如果需要的话,如果是曲线拐点个数等于过滤器设置的拐点个数,那就将这个曲线的拐点个数、面积、拐点坐标、曲线的最小矩阵作为一个元组存进finalCountours里。不需要过滤器的话,那就直接把这些东西放进finalCountours里。
将轮廓从大到小排列:
- 函数sorted():排列顺序。参数:第一个为可排序的对象;key为自定义指标进行排序;reverse排序规则,True为降序,False为升序(默认)
- 函数lambda:一种没有名字的函数。函数名是返回结果,一般用来定义简单的函数。
- ----lambda x:x[1] 第一个x表示列表的第一个元素,这里表示finalCountours中的元组,x是形参,可以用任意字母代替。x[1]代表元组里的第二个元素,在这里也就是area。所以,key=lambda x:x[1]代表根据area的大小进行排序。
- 判断是否画出来轮廓。遍历降序排列之后的finalCountours,根据里面的每个元组(一个元组代表一个轮廓)的第五个元素,即i画出来轮廓。颜色为(0,0,255),红色。
画出来的结果:
为了方便,将预处理和寻找轮廓写成一个方法,以供后续调用。方法参数:图像,边缘检测阈值,是否展示边缘检测后的图像(默认为不展示),最小面积(默认为1000),过滤器的拐点大小(默认为0),是否画最后的轮廓(默认为不画)。返回值:图像+存放有每个轮廓的拐点个数、面积、拐点位置、边界框、轮廓的finalCountours
def getContours(img, cThr=[100,100], showCanny=False, minArea=1000, filter=0, draw=False):
imgGray = cv2.cvtColor(img, cv2.COLOR_BGR2GRAY)
imgBlur = cv2.GaussianBlur(imgGray, (5,5), 1)
imgCanny = cv2.Canny(imgBlur,cThr[0],cThr[1])
kernel = np.ones((5,5))
imgDial = cv2.dilate(imgCanny,kernel,iterations=3)
imgThre = cv2.erode(imgDial,kernel,iterations=2)
if showCanny:cv2.imshow('Canny',imgThre)
# 寻找所有的外轮廓
contours,hiearchy = cv2.findContours(imgThre,cv2.RETR_EXTERNAL,cv2.CHAIN_APPROX_SIMPLE)
finalCountours = []
# 遍历找到的轮廓
for i in contours:
area = cv2.contourArea(i) # 轮廓的面积
if area > minArea: # 如果大于设置的最小轮廓值,就往下走
peri = cv2.arcLength(i,True) # 封闭的轮廓的长度
approx = cv2.approxPolyDP(i,0.02*peri,True) # 封闭轮廓的拐点
bbox = cv2.boundingRect(approx) # 找到边界框
if filter > 0: # 需不需要根据拐点个数进行过滤轮廓
if len(approx)==filter: # 拐点个数,面积,拐点位置,边界框,轮廓
finalCountours.append([len(approx),area,approx,bbox,i])
else:
finalCountours.append([len(approx), area, approx, bbox, i])
finalCountours = sorted(finalCountours,key=lambda x:x[1] , reverse=True) # 根据轮廓大小进行从大到小的排序
if draw: # 是否要画出来轮廓
for con in finalCountours:
cv2.drawContours(img,con[4],-1,(0,0,255),3)
return img,finalCountours
4、找到参照物的轮廓,并且进行图像矫正
# 找到最大轮廓,也就是绿色板子的位置
if len(finalCountours)!=0:
biggest = finalCountours[0][2] # 最大轮廓的曲线拐点位置
因为已经将finalCountours进行排序了,finalCountours[0]就是最大的轮廓,也就是绿色板子的位置。finalCountours[0][2]也就是approx,曲线拐点位置坐标。
接下来就是图像矫正了。想要变成如下图所示的样子。
# 图像矫正的方法
def warpImg(img,points,w,h,pad=20):
points = reorder(points)
pts1 = np.float32(points)
pts2 = np.float32([[0,0],[w,0],[0,h],[w,h]])
matrix = cv2.getPerspectiveTransform(pts1,pts2)
imgWrap = cv2.warpPerspective(img,matrix,(w,h))
return imgWrap
主要的函数是getPerspectiveTransform和warpPerspective。
- 函数getPerspectiveTransform():由四对点计算透射变换。参数:输入图像的四边形顶点坐标pts1;输出图像的相应的四边形顶点坐标pts2。从而得到一个3*3的变换矩阵。pts1就是绿色板子四个点的坐标了,也就是biggest。pts2的坐标,根据最终要的形式来看,左上角的顶点需要变成(0,0),再根据绿色板子的宽和高,就可以推算出四个点的位置了。
- 函数warpPerspective():对图像进行透视变换。参数:需要变换的原始图像;变换矩阵;输出图像的大小。
了解了什么是图像矫正,那就开始想办法得到这些参数:原始图像,pts1,pts2。原始图像已知,pts2已知(绿色板子作为参照物,宽和高是已知的),虽然表面上pst1好像就是biggest,但biggest的四个点的坐标顺序不是按照下图所示进行排序的,所以pts1需要进一步的处理。
左上角1的点,横坐标和纵坐标之和肯定是最小的那个;右下角4的点横坐标和纵坐标之和肯定是最大的那个;右上角2的点,它的差分是最小的;左下角3的点,差分是最大的。
所以书写一个方法,将轮廓坐标按顺序重新排列。
# 将轮廓拐点重新排列的方法
def reorder(myPoints):
myPointsNew = np.zeros_like(myPoints)
myPoints = myPoints.reshape((4,2))
add = myPoints.sum(1)
myPointsNew[0] = myPoints[np.argmin(add)]
myPointsNew[3] = myPoints[np.argmax(add)]
diff = np.diff(myPoints,axis=1)
myPointsNew[1] = myPoints[np.argmin(diff)]
myPointsNew[2] = myPoints[np.argmax(diff)]
return myPointsNew
调用方法。wP为绿板的宽度220,hP为绿板的高度300。
imgWrap = warpImg(img, biggest, wP, hP)
结果发现会出现蓝色箭头所示的边缘地区没有填充满。
所以用pad进行填充。 加入图像矫正的方法里。得到这一步理想的结果。
imgWrap = imgWrap[pad:imgWrap.shape[0]-pad,pad:imgWrap.shape[1]-pad]
5、结束
找到纸片的位置,用线条框出,拐点重新排序。这些步骤和前面一致。
imgContours2, conts2 = utils.getContours(imgWrap, minArea=2000, filter=4, cThr=[50,50])
if len(conts)!=0:
for obj in conts2:
cv2.polylines(imgContours2,[obj[2]],True,(0,255,0),2)
nPoints = utils.reorder(obj[2])
nPoints为每个轮廓重新排序后的四个拐点坐标。
计算纸片宽度和高度。根据勾股定理,计算斜边的长度。用方法表示:
def findDis(pts1,pts2):
return ((pts2[0]-pts1[0])**2 + (pts2[1]-pts1[1])**2)**0.5
调用该方法,转换成厘米为单位,然后保留一位小数:
nW = round((findDis(nPoints[0][0],nPoints[1][0])/10),1)
nH = round((findDis(nPoints[0][0],nPoints[2][0])/10),1)
创建箭头:
cv2.arrowedLine(imgContours2, (nPoints[0][0][0],nPoints[0][0][1]),(nPoints[1][0][0],nPoints[1][0][1]),(255,0,255),3,8,0,0.05)
cv2.arrowedLine(imgContours2, (nPoints[0][0][0],nPoints[0][0][1]),(nPoints[2][0][0],nPoints[2][0][1]),(255,0,255),3,8,0,0.05)
把尺寸也标上去:
x,y,w,h = obj[3]
cv2.putText(imgContours2,'{}cm'.format(nW),(x+30,y-10),cv2.FONT_HERSHEY_COMPLEX_SMALL,1,(255,0,255),2)
cv2.putText(imgContours2, '{}cm'.format(nH), (x - 70, y + h // 2), cv2.FONT_HERSHEY_COMPLEX_SMALL, 1, (255, 0, 255), 2)
最终结果:
完整代码:
其中scale主要也是想让矫正后的图片能显示得大点。后面用绿板的尺寸计算纸片的时候再除回去的。
import cv2
import numpy as np
import cv2
import numpy as np
scale = 2
wP = 220*scale
hP = 300*scale
def getContours(img, cThr=[100,100], showCanny=False, minArea=1000, filter=0, draw=False):
imgGray = cv2.cvtColor(img, cv2.COLOR_BGR2GRAY)
imgBlur = cv2.GaussianBlur(imgGray, (5,5), 1)
imgCanny = cv2.Canny(imgBlur,cThr[0],cThr[1])
kernel = np.ones((5,5))
imgDial = cv2.dilate(imgCanny,kernel,iterations=3)
imgThre = cv2.erode(imgDial,kernel,iterations=2)
if showCanny:cv2.imshow('Canny',imgThre)
# 寻找所有的外轮廓
contours,hiearchy = cv2.findContours(imgThre,cv2.RETR_EXTERNAL,cv2.CHAIN_APPROX_SIMPLE)
finalCountours = []
# 遍历找到的轮廓
for i in contours:
area = cv2.contourArea(i) # 轮廓的面积
if area > minArea: # 如果大于设置的最小轮廓值,就往下走
peri = cv2.arcLength(i,True) # 封闭的轮廓的长度
approx = cv2.approxPolyDP(i,0.02*peri,True) # 封闭轮廓的拐点
bbox = cv2.boundingRect(approx) # 找到边界框
if filter > 0: # 需不需要根据拐点个数进行过滤轮廓
if len(approx)==filter: # 拐点个数,面积,拐点位置,边界框,轮廓
finalCountours.append([len(approx),area,approx,bbox,i])
else:
finalCountours.append([len(approx), area, approx, bbox, i])
finalCountours = sorted(finalCountours,key=lambda x:x[1] , reverse=True) # 根据轮廓大小进行从大到小的排序
if draw: # 是否要画出来轮廓
for con in finalCountours:
cv2.drawContours(img,con[4],-1,(0,0,255),3)
return img,finalCountours
# 四个点是随机的,于是重新排序
def reorder(myPoints):
myPointsNew = np.zeros_like(myPoints)
myPoints = myPoints.reshape((4,2))
add = myPoints.sum(1)
myPointsNew[0] = myPoints[np.argmin(add)]
myPointsNew[3] = myPoints[np.argmax(add)]
diff = np.diff(myPoints,axis=1)
myPointsNew[1] = myPoints[np.argmin(diff)]
myPointsNew[2] = myPoints[np.argmax(diff)]
return myPointsNew
def warpImg(img,points,w,h,pad=20):
# print(points)
points = reorder(points)
pts1 = np.float32(points)
pts2 = np.float32([[0,0],[w,0],[0,h],[w,h]])
matrix = cv2.getPerspectiveTransform(pts1,pts2)
imgWrap = cv2.warpPerspective(img,matrix,(w,h))
imgWrap = imgWrap[pad:imgWrap.shape[0]-pad,pad:imgWrap.shape[1]-pad]
return imgWrap
def findDis(pts1,pts2):
return ((pts2[0]-pts1[0])**2 + (pts2[1]-pts1[1])**2)**0.5
path = '1.jpg'
img = cv2.imread(path)
img = cv2.resize(img, (0, 0), None, 0.18, 0.18)
img, conts = getContours(img, minArea=8000, filter=4)
if len(conts) != 0:
biggest = conts[0][2] # 最大轮廓的拐点位置
# print(biggest)
imgWrap = warpImg(img, biggest, wP, hP)
imgContours2, conts2 = getContours(imgWrap, minArea=2000, filter=4, cThr=[50, 50])
if len(conts) != 0:
for obj in conts2:
cv2.polylines(imgContours2, [obj[2]], True, (0, 255, 0), 2)
nPoints = reorder(obj[2])
nW = round((findDis(nPoints[0][0] // scale, nPoints[1][0] // scale) / 10), 1)
nH = round((findDis(nPoints[0][0] // scale, nPoints[2][0] // scale) / 10), 1)
# 创建箭头
cv2.arrowedLine(imgContours2, (nPoints[0][0][0], nPoints[0][0][1]), (nPoints[1][0][0], nPoints[1][0][1]),
(255, 0, 255), 3, 8, 0, 0.05)
cv2.arrowedLine(imgContours2, (nPoints[0][0][0], nPoints[0][0][1]), (nPoints[2][0][0], nPoints[2][0][1]),
(255, 0, 255), 3, 8, 0, 0.05)
x, y, w, h = obj[3]
cv2.putText(imgContours2, '{}cm'.format(nW), (x + 30, y - 10), cv2.FONT_HERSHEY_COMPLEX_SMALL, 1,
(255, 0, 255), 2)
cv2.putText(imgContours2, '{}cm'.format(nH), (x - 70, y + h // 2), cv2.FONT_HERSHEY_COMPLEX_SMALL, 1,
(255, 0, 255), 2)
cv2.imshow('background', imgContours2)
cv2.imshow('Original', img)
cv2.waitKey(0)
实时实现物体尺寸计算代码:
注意:必须有参照物在,不然实现不了。
放方法的程序utils.py:
import cv2
import numpy as np
def getContours(img, cThr=[100,100], showCanny=False, minArea=1000, filter=0, draw=False):
imgGray = cv2.cvtColor(img, cv2.COLOR_BGR2GRAY)
imgBlur = cv2.GaussianBlur(imgGray, (5,5), 1)
imgCanny = cv2.Canny(imgBlur,cThr[0],cThr[1])
kernel = np.ones((5,5))
imgDial = cv2.dilate(imgCanny,kernel,iterations=3)
imgThre = cv2.erode(imgDial,kernel,iterations=2)
if showCanny:cv2.imshow('Canny',imgThre)
# 寻找所有的外轮廓
contours,hiearchy = cv2.findContours(imgThre,cv2.RETR_EXTERNAL,cv2.CHAIN_APPROX_SIMPLE)
finalCountours = []
# 遍历找到的轮廓
for i in contours:
area = cv2.contourArea(i) # 轮廓的面积
if area > minArea: # 如果大于设置的最小轮廓值,就往下走
peri = cv2.arcLength(i,True) # 封闭的轮廓的长度
approx = cv2.approxPolyDP(i,0.02*peri,True) # 封闭轮廓的拐点
bbox = cv2.boundingRect(approx) # 找到边界框
if filter > 0: # 需不需要根据拐点个数进行过滤轮廓
if len(approx)==filter: # 拐点个数,面积,拐点位置,边界框,轮廓
finalCountours.append([len(approx),area,approx,bbox,i])
else:
finalCountours.append([len(approx), area, approx, bbox, i])
finalCountours = sorted(finalCountours,key=lambda x:x[1] , reverse=True) # 根据轮廓大小进行从大到小的排序
if draw: # 是否要画出来轮廓
for con in finalCountours:
cv2.drawContours(img,con[4],-1,(0,0,255),3)
return img,finalCountours
# 四个点是随机的,于是重新排序
def reorder(myPoints):
myPointsNew = np.zeros_like(myPoints)
myPoints = myPoints.reshape((4,2))
add = myPoints.sum(1)
myPointsNew[0] = myPoints[np.argmin(add)]
myPointsNew[3] = myPoints[np.argmax(add)]
diff = np.diff(myPoints,axis=1)
myPointsNew[1] = myPoints[np.argmin(diff)]
myPointsNew[2] = myPoints[np.argmax(diff)]
return myPointsNew
def warpImg(img,points,w,h,pad=20):
# print(points)
points = reorder(points)
pts1 = np.float32(points)
pts2 = np.float32([[0,0],[w,0],[0,h],[w,h]])
matrix = cv2.getPerspectiveTransform(pts1,pts2)
imgWrap = cv2.warpPerspective(img,matrix,(w,h))
imgWrap = imgWrap[pad:imgWrap.shape[0]-pad,pad:imgWrap.shape[1]-pad]
return imgWrap
def findDis(pts1,pts2):
return ((pts2[0]-pts1[0])**2 + (pts2[1]-pts1[1])**2)**0.5
主程序:
import cv2
import numpy as np
import utils
########################################
webcam = False
path = '1.jpg'
cap = cv2.VideoCapture(0)
cap.set(10,160) # 改变亮度
cap.set(3,680) # 改变宽度
cap.set(4,1080) # 改变高度
scale = 2
wP = 220*scale
hP = 300*scale
while True:
if webcam:success,img = cap.read() # 如果webCam为True,那就打开摄像头
else:img = cv2.imread(path) # 否则就读图片
img = cv2.resize(img, (0,0),None,0.18,0.18)
img, conts = utils.getContours(img,minArea=8000,filter=4)
if len(conts)!=0:
biggest = conts[0][2] # 最大轮廓的拐点位置
# print(biggest)
imgWrap = utils.warpImg(img, biggest, wP, hP)
imgContours2, conts2 = utils.getContours(imgWrap, minArea=2000, filter=4, cThr=[50,50])
if len(conts)!=0:
for obj in conts2:
cv2.polylines(imgContours2,[obj[2]],True,(0,255,0),2)
nPoints = utils.reorder(obj[2])
nW = round((utils.findDis(nPoints[0][0]//scale,nPoints[1][0]//scale)/10),1)
nH = round((utils.findDis(nPoints[0][0]//scale,nPoints[2][0]//scale)/10),1)
# 创建箭头
cv2.arrowedLine(imgContours2, (nPoints[0][0][0],nPoints[0][0][1]),(nPoints[1][0][0],nPoints[1][0][1]),
(255,0,255),3,8,0,0.05)
cv2.arrowedLine(imgContours2, (nPoints[0][0][0],nPoints[0][0][1]),(nPoints[2][0][0],nPoints[2][0][1]),
(255,0,255),3,8,0,0.05)
x,y,w,h = obj[3]
cv2.putText(imgContours2,'{}cm'.format(nW),(x+30,y-10),cv2.FONT_HERSHEY_COMPLEX_SMALL,1,(255,0,255),2)
cv2.putText(imgContours2, '{}cm'.format(nH), (x - 70, y + h // 2), cv2.FONT_HERSHEY_COMPLEX_SMALL, 1, (255, 0, 255), 2)
cv2.imshow('background', imgContours2)
cv2.imshow('Original',img)
cv2.waitKey(1)
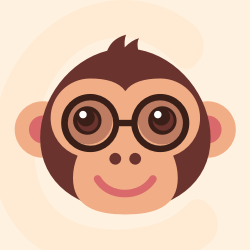



更多推荐
所有评论(0)