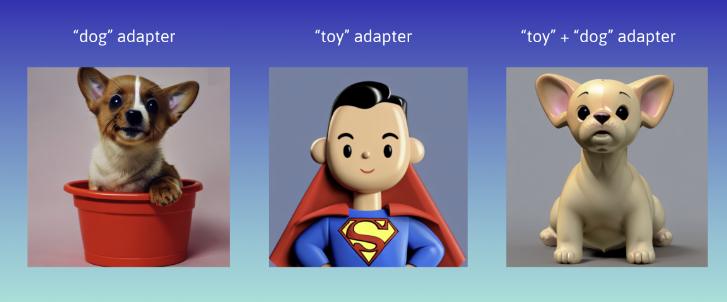
PEFT微调方式总结
PEFT微调方式总结
PEFT微调方式总结
PEFT介绍
PEFT 是 Huggingface 开源的一个参数高效微调库,它提供了最新的参数高效微调技术,并且可以与 Transformers 和 Accelerate 进行无缝集成。
安装peft
pip install peft
支持的微调方法和任务
class PeftType(str, enum.Enum):
PROMPT_TUNING = "PROMPT_TUNING"
P_TUNING = "P_TUNING"
PREFIX_TUNING = "PREFIX_TUNING"
LORA = "LORA"
ADALORA = "ADALORA"
ADAPTION_PROMPT = "ADAPTION_PROMPT"
class TaskType(str, enum.Enum):
SEQ_CLS = "SEQ_CLS"
SEQ_2_SEQ_LM = "SEQ_2_SEQ_LM"
CAUSAL_LM = "CAUSAL_LM"
TOKEN_CLS = "TOKEN_CLS"
SEQ_CLS
序列分类(Sequence Classification),对整个句子进行分类。如: 获取评论的情绪,检测电子邮件是否为垃圾邮件,确定句子在语法上是否正确或两个句子在逻辑上是否相关等
SEQ_2_SEQ_LM
条件生成任务,根据给定的输入(可能是文本、图片等)生成符合条件的输出。
与因果语言建模任务不同,条件生成不仅仅关注于给定上下文的连贯性,还关注于满足预定的任务要求。因果语言建模仅关注于根据给定的上下文生成文本序列。
条件生成的应用包括但不限于机器翻译、文本摘要、图像描述等。这些任务通常需要模型在输入和输出之间建立复杂的映射关系。
CAUSAL_LM
因果语言建模任务(CLM),在这种建模方法中,模型试图预测给定上下文中的下一个单词,该上下文通常包括在当前单词之前的所有单词。这种建模方法遵循因果原则,即当前单词只受到其前面单词的影响,而不受后面单词的影响。代表模型有GPT2、Bloom、OPT、GPT-Neo、GPT-J、LLaMA、ChatGLM。
TOKEN_CLS
Token 分类任务(Token Classification),对句子中的每个词进行分类。如: 识别句子的语法成分(名词、动词、形容词)或命名实体(人、地点、组织)。
模型加载
import transformers
model = transformers.AutoModelForCausalLM.from_pretrained(
model_args.model_name_or_path,
cache_dir=training_args.cache_dir,
device_map='auto',
torch_dtype='auto',
trust_remote_code=True
)
tokenizer = transformers.AutoTokenizer.from_pretrained(
model_args.model_name_or_path, trust_remote_code=True)
微调加载
PROMPT_TUNING
简介
它为每个任务定义了独特的提示(Prompt),并将这些提示与数据拼接以作为输入,但仅在输入层添加提示标记。
源码
class PromptEmbedding(torch.nn.Module):
"""
The model to encode virtual tokens into prompt embeddings.
Args:
config ([`PromptTuningConfig`]): The configuration of the prompt embedding.
word_embeddings (`torch.nn.Module`): The word embeddings of the base transformer model.
**Attributes**:
- **embedding** (`torch.nn.Embedding`) -- The embedding layer of the prompt embedding.
Example:
```py
>>> from peft import PromptEmbedding, PromptTuningConfig
>>> config = PromptTuningConfig(
... peft_type="PROMPT_TUNING",
... task_type="SEQ_2_SEQ_LM",
... num_virtual_tokens=20,
... token_dim=768,
... num_transformer_submodules=1,
... num_attention_heads=12,
... num_layers=12,
... prompt_tuning_init="TEXT",
... prompt_tuning_init_text="Predict if sentiment of this review is positive, negative or neutral",
... tokenizer_name_or_path="t5-base",
... )
>>> # t5_model.shared is the word embeddings of the base model
>>> prompt_embedding = PromptEmbedding(config, t5_model.shared)
```
Input Shape: (`batch_size`, `total_virtual_tokens`)
Output Shape: (`batch_size`, `total_virtual_tokens`, `token_dim`)
"""
def __init__(self, config, word_embeddings):
super().__init__()
total_virtual_tokens = config.num_virtual_tokens * config.num_transformer_submodules
self.embedding = torch.nn.Embedding(total_virtual_tokens, config.token_dim)
if config.prompt_tuning_init == PromptTuningInit.TEXT and not config.inference_mode:
from transformers import AutoTokenizer
tokenizer_kwargs = config.tokenizer_kwargs or {}
tokenizer = AutoTokenizer.from_pretrained(config.tokenizer_name_or_path, **tokenizer_kwargs)
init_text = config.prompt_tuning_init_text
init_token_ids = tokenizer(init_text)["input_ids"]
# Trim or iterate until num_text_tokens matches total_virtual_tokens
num_text_tokens = len(init_token_ids)
if num_text_tokens > total_virtual_tokens:
init_token_ids = init_token_ids[:total_virtual_tokens]
elif num_text_tokens < total_virtual_tokens:
num_reps = math.ceil(total_virtual_tokens / num_text_tokens)
init_token_ids = init_token_ids * num_reps
init_token_ids = init_token_ids[:total_virtual_tokens]
init_token_ids = torch.LongTensor(init_token_ids).to(word_embeddings.weight.device)
word_embedding_weights = word_embeddings(init_token_ids).detach().clone()
word_embedding_weights = word_embedding_weights.to(torch.float32)
self.embedding.weight = torch.nn.Parameter(word_embedding_weights)
def forward(self, indices):
# Just get embeddings
prompt_embeddings = self.embedding(indices)
return prompt_embeddings
demo
from peft import PromptTuningConfig,PromptTuningInit
peft_config = PromptTuningConfig(
task_type=TaskType.CAUSAL_LM,
prompt_tuning_init=PromptTuningInit.TEXT,
num_virtual_tokens=8,
prompt_tuning_init_text="Classify if the tweet is a complaint or not:",
tokenizer_name_or_path=model_args.model_name_or_path,
)
model = get_peft_model(model, peft_config)
model.print_trainable_parameters()
PromptTuningConfig配置类参数说明:
- task_type:指定任务类型。如:条件生成任务(SEQ_2_SEQ_LM),因果语言建模(CAUSAL_LM)等。
- prompt_tuning_init:提示嵌入的初始化方法。PEFT支持文本(TEXT)和随机(RANDOM)两种初始化方式。Prompt token 的初始化方法和长度对于模型性能有一定影响。与随机初始化和使用样本词汇表初始化相比,Prompt Tuning 采用类标签初始化模型的效果更佳。然而,随着模型参数规模的提升,这种差距最终会减小。因此,若需同时使用类标签和样本词汇表初始化,请指定为TEXT。
- prompt_tuning_init_text:用于文本初始化提示嵌入时的方法。
- num_virtual_tokens:指定虚拟 Token 数。当提示虚拟 Token 的长度在20左右时,性能表现良好。超过20后,增加 Prompt token 长度对模型性能提升影响不大;同样,这个差距会随着模型参数规模的提升而减小。
P_TUNING
简介
该方法将 Prompt 转换为可以学习的 Embedding 层,并用MLP+LSTM的方式来对Prompt Embedding进行一层处理。
源码
class PromptEncoder(torch.nn.Module):
"""
The prompt encoder network that is used to generate the virtual token embeddings for p-tuning.
Args:
config ([`PromptEncoderConfig`]): The configuration of the prompt encoder.
Example:
```py
>>> from peft import PromptEncoder, PromptEncoderConfig
>>> config = PromptEncoderConfig(
... peft_type="P_TUNING",
... task_type="SEQ_2_SEQ_LM",
... num_virtual_tokens=20,
... token_dim=768,
... num_transformer_submodules=1,
... num_attention_heads=12,
... num_layers=12,
... encoder_reparameterization_type="MLP",
... encoder_hidden_size=768,
... )
>>> prompt_encoder = PromptEncoder(config)
```
**Attributes**:
- **embedding** (`torch.nn.Embedding`) -- The embedding layer of the prompt encoder.
- **mlp_head** (`torch.nn.Sequential`) -- The MLP head of the prompt encoder if `inference_mode=False`.
- **lstm_head** (`torch.nn.LSTM`) -- The LSTM head of the prompt encoder if `inference_mode=False` and
`encoder_reparameterization_type="LSTM"`.
- **token_dim** (`int`) -- The hidden embedding dimension of the base transformer model.
- **input_size** (`int`) -- The input size of the prompt encoder.
- **output_size** (`int`) -- The output size of the prompt encoder.
- **hidden_size** (`int`) -- The hidden size of the prompt encoder.
- **total_virtual_tokens** (`int`): The total number of virtual tokens of the
prompt encoder.
- **encoder_type** (Union[[`PromptEncoderReparameterizationType`], `str`]): The encoder type of the prompt
encoder.
Input shape: (`batch_size`, `total_virtual_tokens`)
Output shape: (`batch_size`, `total_virtual_tokens`, `token_dim`)
"""
def __init__(self, config):
super().__init__()
self.token_dim = config.token_dim
self.input_size = self.token_dim
self.output_size = self.token_dim
self.hidden_size = config.encoder_hidden_size
self.total_virtual_tokens = config.num_virtual_tokens * config.num_transformer_submodules
self.encoder_type = config.encoder_reparameterization_type
# embedding
self.embedding = torch.nn.Embedding(self.total_virtual_tokens, self.token_dim)
if not config.inference_mode:
if self.encoder_type == PromptEncoderReparameterizationType.LSTM:
lstm_dropout = config.encoder_dropout
num_layers = config.encoder_num_layers
# LSTM
self.lstm_head = torch.nn.LSTM(
input_size=self.input_size,
hidden_size=self.hidden_size,
num_layers=num_layers,
dropout=lstm_dropout,
bidirectional=True,
batch_first=True,
)
self.mlp_head = torch.nn.Sequential(
torch.nn.Linear(self.hidden_size * 2, self.hidden_size * 2),
torch.nn.ReLU(),
torch.nn.Linear(self.hidden_size * 2, self.output_size),
)
elif self.encoder_type == PromptEncoderReparameterizationType.MLP:
encoder_num_layers_default = PromptEncoderConfig.encoder_num_layers
if config.encoder_num_layers != encoder_num_layers_default:
warnings.warn(
f"for {self.encoder_type.value}, the argument `encoder_num_layers` is ignored. "
f"Exactly {encoder_num_layers_default} MLP layers are used."
)
layers = [
torch.nn.Linear(self.input_size, self.hidden_size),
torch.nn.ReLU(),
torch.nn.Linear(self.hidden_size, self.hidden_size),
torch.nn.ReLU(),
torch.nn.Linear(self.hidden_size, self.output_size),
]
self.mlp_head = torch.nn.Sequential(*layers)
else:
raise ValueError("Prompt encoder type not recognized. Please use one of MLP (recommended) or LSTM.")
def forward(self, indices):
input_embeds = self.embedding(indices)
if self.encoder_type == PromptEncoderReparameterizationType.LSTM:
output_embeds = self.mlp_head(self.lstm_head(input_embeds)[0])
elif self.encoder_type == PromptEncoderReparameterizationType.MLP:
output_embeds = self.mlp_head(input_embeds)
else:
raise ValueError("Prompt encoder type not recognized. Please use one of MLP (recommended) or LSTM.")
return output_embeds
demo
from peft import PromptEncoderConfig,get_peft_config,TaskType
peft_config = PromptEncoderConfig(task_type=TaskType.CAUSAL_LM, num_virtual_tokens=20, encoder_hidden_size=128)
model = get_peft_model(model, peft_config)
model.print_trainable_parameters()
PromptEncoderConfig配置类参数说明:
- task_type:训练的任务类型,如:序列分类(SEQ_CLS),因果语言建模(CAUSAL_LM)等。
- num_virtual_tokens:虚拟token的数量,换句话说就是提示(prompt)。
- encoder_hidden_size:编码器的隐藏大小,用于优化提示参数。
- encoder_reparameterization_type:指定如何重新参数化提示编码器,可选项有:MLP 或 LSTM,默认值为 MLP。
PREFIX_TUNING
简介
在输入token之前构造一段任务相关的virtual tokens作为Prefix;然后,在训练的时候只更新Prefix部分的参数,而 PLM 中的其他部分参数固定。同时,为了防止直接更新 Prefix 的参数导致训练不稳定和性能下降的情况,在 Prefix 层前面加了 MLP 结构,训练完成后,只保留 Prefix 的参数。
源码
class PrefixEncoder(torch.nn.Module):
r"""
The `torch.nn` model to encode the prefix.
Args:
config ([`PrefixTuningConfig`]): The configuration of the prefix encoder.
Example:
```py
>>> from peft import PrefixEncoder, PrefixTuningConfig
>>> config = PrefixTuningConfig(
... peft_type="PREFIX_TUNING",
... task_type="SEQ_2_SEQ_LM",
... num_virtual_tokens=20,
... token_dim=768,
... num_transformer_submodules=1,
... num_attention_heads=12,
... num_layers=12,
... encoder_hidden_size=768,
... )
>>> prefix_encoder = PrefixEncoder(config)
```
**Attributes**:
- **embedding** (`torch.nn.Embedding`) -- The embedding layer of the prefix encoder.
- **transform** (`torch.nn.Sequential`) -- The two-layer MLP to transform the prefix embeddings if
`prefix_projection` is `True`.
- **prefix_projection** (`bool`) -- Whether to project the prefix embeddings.
Input shape: (`batch_size`, `num_virtual_tokens`)
Output shape: (`batch_size`, `num_virtual_tokens`, `2*layers*hidden`)
"""
def __init__(self, config):
super().__init__()
self.prefix_projection = config.prefix_projection
token_dim = config.token_dim
num_layers = config.num_layers
encoder_hidden_size = config.encoder_hidden_size
num_virtual_tokens = config.num_virtual_tokens
if self.prefix_projection and not config.inference_mode:
# Use a two-layer MLP to encode the prefix
self.embedding = torch.nn.Embedding(num_virtual_tokens, token_dim)
self.transform = torch.nn.Sequential(
torch.nn.Linear(token_dim, encoder_hidden_size),
torch.nn.Tanh(),
torch.nn.Linear(encoder_hidden_size, num_layers * 2 * token_dim),
)
else:
self.embedding = torch.nn.Embedding(num_virtual_tokens, num_layers * 2 * token_dim)
def forward(self, prefix: torch.Tensor):
if self.prefix_projection:
prefix_tokens = self.embedding(prefix)
past_key_values = self.transform(prefix_tokens)
else:
past_key_values = self.embedding(prefix)
return past_key_values
demo
from peft import PrefixTuningConfig,get_peft_config,TaskType
peft_config = PrefixTuningConfig(task_type=TaskType.CAUSAL_LM, num_virtual_tokens=30)
model = get_peft_model(model, peft_config)
model.print_trainable_parameters()
PrefixTuningConfig 配置类参数说明:
- task_type:指定任务类型。如:条件生成任务(SEQ_2_SEQ_LM),因果语言建模(CAUSAL_LM)等。
- num_virtual_tokens:虚拟token的数量,换句话说就是提示(prompt)。
- inference_mode:是否在推理模式下使用Peft模型。
- prefix_projection:是否投影前缀嵌入(token),默认值为false,表示使用P-Tuning v2, 如果为true,则表示使用 Prefix Tuning。
Prefix Tuning 与 P-Tuning v2 最主要的差别就是是否进行重新参数化编码,包含两个线性层的多层感知机(MLP)。
LORA
简介
该方法的核心思想就是通过低秩分解来模拟参数的改变量,从而以极小的参数量来实现大模型的间接训练。
demo
from peft import LoraConfig, get_peft_model
LORA_R = 32
LORA_DROPOUT = 0.05
TARGET_MODULES = [
"o_proj","gate_proj", "down_proj", "up_proj"
]
config = LoraConfig(
r=LORA_R,
target_modules=TARGET_MODULES,
lora_dropout=LORA_DROPOUT,
bias="none",
task_type="CAUSAL_LM",
)
model = get_peft_model(model, config)
model.print_trainable_parameters()
LoraConfig配置类参数说明:
task_type
:指定任务类型。如:条件生成任务(SEQ_2_SEQ_LM),因果语言建模(CAUSAL_LM)等。inference_mode
:是否在推理模式下使用Peft模型。r
: LoRA低秩矩阵的维数。关于秩的选择,通常,使用4,8,16即可。lora_alpha
: LoRA低秩矩阵的缩放系数,为一个常数超参,调整alpha与调整学习率类似。lora_dropout
:LoRA 层的丢弃(dropout)率,取值范围为[0, 1)
。target_modules
:要替换为 LoRA 的模块名称列表或模块名称的正则表达式。针对不同类型的模型,模块名称不一样,因此,我们需要根据具体的模型进行设置,比如,LLaMa的默认模块名为[q_proj, v_proj]
,我们也可以自行指定为:[q_proj,k_proj,v_proj,o_proj]
。 在 PEFT 中支持的模型默认的模块名如下所示:
ADALORA
简介
● Adalora,即自适应 LORA,主要通过在不同的 Transformer Block 层中动态分配原生 LORA 中的秩,确保这些秩在微调过程中能够随着 block 重要性的变化而变化。
● Adalora 的效果通常比 LORA 更好,原因在于 LORA 使用两个矩阵 BA 来拟合满秩张量,而 Adalora 使用三个矩阵 PAQ,并在损失函数中限制 P 和 Q 正 交。这种拟合方式符合奇异值分解(SVD)的原理。
● 在微调训练的每一步,根据 block 中参数对损失的影响计算其重要性,取 top N 为秩进行下一步的正向计算。然后在接下来的反向传播中重新计算重要性, 以此实现动态分配。
demo
from peft import AdaLoraConfig, get_peft_model
LORA_R = 32
LORA_DROPOUT = 0.05
config = LoraConfig(
r=LORA_R,
target_modules=TARGET_MODULES,
lora_dropout=LORA_DROPOUT,
bias="none",
task_type="CAUSAL_LM",
)
config = AdaLoraConfig(
peft_type="ADALORA", task_type="CAUSAL_LM", r=LORA_R, lora_alpha=32, target_modules=["q", "v"],
lora_dropout=LORA_DROPOUT,
)
model = get_peft_model(model, config)
model.print_trainable_parameters()
微调模型合并
- 加载微调模型
base_model_name_or_path = "internlm-7b"
lora_model_name_or_path = "/checkpoint-9695"
model = AutoModelForCausalLM.from_pretrained(
base_model_name_or_path,
torch_dtype="auto",
trust_remote_code=True,
).cuda(0)
model =PeftModel.from_pretrained(model,model_id=lora_model_name_or_path)
model.eval()
tokenizer = AutoTokenizer.from_pretrained(
base_model_name_or_path, trust_remote_code=True, padding_side="left"
)
- 合并模型
model = model.merge_and_unload()
model.save_pretrained("internlm-7b-lml")
tokenizer.save_pretrained("internlm-7b-lml")
模型推理
- 加载微调模型
base_model_name_or_path = "internlm-7b"
lora_model_name_or_path = "/checkpoint-9695"
model = AutoModelForCausalLM.from_pretrained(
base_model_name_or_path,
torch_dtype="auto",
trust_remote_code=True,
).cuda(0)
model =PeftModel.from_pretrained(model,model_id=lora_model_name_or_path)
model.eval()
tokenizer = AutoTokenizer.from_pretrained(
base_model_name_or_path, trust_remote_code=True, padding_side="left"
)
- 定义批量推理函数
def batch_generate_data(
text_input: List[str], use_train_model: bool = True, temp: float = 0.7
):
text_input_format = [generate_input(i) for i in text_input]
batch_inputs = tokenizer.batch_encode_plus(
text_input_format, padding="longest", return_tensors="pt"
)
batch_inputs["input_ids"] = batch_inputs["input_ids"].cuda()
batch_inputs["attention_mask"] = batch_inputs["attention_mask"].cuda()
if use_train_model:
# with model.disable_adapter():
outputs = model.generate(
**batch_inputs,
max_new_tokens=256,
do_sample=True,
temperature=temp,
top_p=0.8,
)
else:
with model.disable_adapter():
outputs = model.generate(
**batch_inputs,
max_new_tokens=256,
do_sample=True,
temperature=temp,
top_p=0.8,
)
outputs = tokenizer.batch_decode(
outputs.cpu()[:, batch_inputs["input_ids"].shape[-1] :],
skip_special_tokens=True,
)
return outputs
- 调用推理
text_input = ["工作压力太大怎么办\n"] * 32
batch_generate_data(text_input, use_train_model=True, temp=0.8)
# 原来的模型
batch_generate_data(text_input, use_train_model=False, temp=0.8)
更多推荐
所有评论(0)