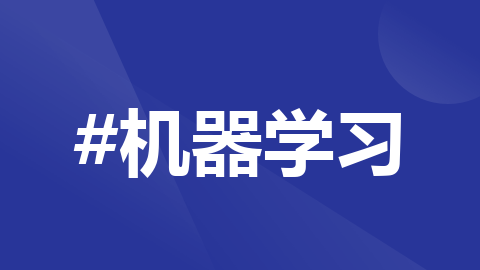
深度学习:huggingface简单介绍---常用函数(数据集处理+dict+model),简单使用(tokenzier + model),简单例子(中文分类+中文填空+中文句子关系推断)
一、什么是huggingface?
huggingface是一个开源社区,它提供了先进的NLP模型,数据集、以及其它便利工具。
huggingface提供的模型非常多,但主要的模型为:
自回归: GPT2 Trasnformer-XL XLNet
自编码: BERT ALBERT RoBERTa ELECTRA
SeqtoSeq: BART Pegasus T5
----------------------------------------------------------------------------------------------------------
自回归模型:预测下一个词,因为使用x获取y叫做回归,所有x预测下一个x称为自回归
自编码模型:还原出本身,根据上下为还原出本身的词
Seq2Seq: 从一个文本序列到另一个序列,比如机器翻译
二、huggingface的简单使用(以bert base chinese: bert中文模型为例子)
- 分词器tokenizer的使用:
from transformers import BertTokenizer
//加载分词器tokenizer时要传入一个name, 这个name往往和模型的name是一致的(即一个模型对应了一个分词器tokenizer)
tokenizer = BertTokenizer.from_pretrained(
pretrained_model_name_or_path=‘bert-base-chinese’,
cache_dir=None,
force_download=False,
)
sents = [
‘选择珠江花园的原因就是方便。’,
‘笔记本的键盘确实爽。’,
‘房间太小。其他的都一般。’,
‘今天才知道这书还有第6卷,真有点郁闷.’,
‘机器背面似乎被撕了张什么标签,残胶还在。’,
]
#编码两个句子(tokenizer.encode一次只能编码一个 or 一对句子)
out = tokenizer.encode(
text=sents[0],
text_pair=sents[1], //可不传,相当于只编码一个句子
#当句子长度大于max_length时,截断
truncation=True,
#一律补pad到max_length长度
padding=‘max_length’,
add_special_tokens=True,
//最大查高度
max_length=30,
return_tensors=None, //默认返回list, tf是指tensorflow, pt是指pytorch, np是指numpy
)
print(out)
// 输出为:[101, 6848, 2885, 4403, 3736, 5709, 1736, 4638, 1333, 1728, 2218, 3221, 3175, 912, 511, 102, 5011, 6381, 3315, 4638, 7241, 4669, 4802, 2141, 4272, 511, 102, 0, 0, 0]
tokenizer.decode(out)
// 输出为:[CLS] 选 择 珠 江 花 园 的 原 因 就 是 方 便 。 [SEP] 笔 记 本 的 键 盘 确 实 爽 。 [SEP] [PAD] [PAD] [PAD]
(CLS句子开始,SEP句子与句子之间分割符号,PAD句子长度不够时的补充符号)
#增强的编码函数(tokenizer.encode_plus)
out = tokenizer.encode_plus(
text=sents[0],
text_pair=sents[1],
#当句子长度大于max_length时,截断
truncation=True,
#一律补零到max_length长度
padding=‘max_length’,
max_length=30,
add_special_tokens=True,
#可取值tf,pt,np,默认为返回list
return_tensors=None,
#返回token_type_ids
return_token_type_ids=True,
#返回attention_mask
return_attention_mask=True,
#返回special_tokens_mask 特殊符号标识
return_special_tokens_mask=True,
#返回offset_mapping 标识每个词的起止位置,这个参数只能BertTokenizerFast使用
#return_offsets_mapping=True,
#返回length 标识长度
return_length=True,
)
for k, v in out.items():
print(k, ‘:’, v)
//输出为:
input_ids : [101, 6848, 2885, 4403, 3736, 5709, 1736, 4638, 1333, 1728, 2218, 3221, 3175, 912, 511, 102, 5011, 6381, 3315, 4638, 7241, 4669, 4802, 2141, 4272, 511, 102, 0, 0, 0] (input_ids 就是编码后的词)
token_type_ids : [0, 0, 0, 0, 0, 0, 0, 0, 0, 0, 0, 0, 0, 0, 0, 0, 1, 1, 1, 1, 1, 1, 1, 1, 1, 1, 1, 0, 0, 0](第一个句子和特殊符号的位置是0,第二个句子的位置是1)
special_tokens_mask : [1, 0, 0, 0, 0, 0, 0, 0, 0, 0, 0, 0, 0, 0, 0, 1, 0, 0, 0, 0, 0, 0, 0, 0, 0, 0, 1, 1, 1, 1]( 特殊符号的位置是1,其他位置是0)
attention_mask : [1, 1, 1, 1, 1, 1, 1, 1, 1, 1, 1, 1, 1, 1, 1, 1, 1, 1, 1, 1, 1, 1, 1, 1, 1, 1, 1, 0, 0, 0]( pad的位置是0,其他位置是1)
length : 30
tokenizer.decode(out[‘input_ids’])
//输出为:‘[CLS] 选 择 珠 江 花 园 的 原 因 就 是 方 便 。 [SEP] 笔 记 本 的 键 盘 确 实 爽 。 [SEP] [PAD] [PAD] [PAD]’
#批量编码句子(tokenizer.batch_encode_plus,编码一个一个句子)
out = tokenizer.batch_encode_plus(
batch_text_or_text_pairs=[sents[0], sents[1]],
add_special_tokens=True,
#当句子长度大于max_length时,截断
truncation=True,
#一律补零到max_length长度
padding=‘max_length’,
max_length=15,
#可取值tf,pt,np,默认为返回list
return_tensors=None,
#返回token_type_ids
return_token_type_ids=True,
#返回attention_mask
return_attention_mask=True,
#返回special_tokens_mask 特殊符号标识
return_special_tokens_mask=True,
#返回offset_mapping 标识每个词的起止位置,这个参数只能BertTokenizerFast使用
#return_offsets_mapping=True,
#返回length 标识长度
return_length=True,
)
for k, v in out.items():
print(k, ‘:’, v)
//输出:
input_ids : [[101, 6848, 2885, 4403, 3736, 5709, 1736, 4638, 1333, 1728, 2218, 3221, 3175, 912, 102], [101, 5011, 6381, 3315, 4638, 7241, 4669, 4802, 2141, 4272, 511, 102, 0, 0, 0]](input_ids 就是编码后的词)
token_type_ids : [[0, 0, 0, 0, 0, 0, 0, 0, 0, 0, 0, 0, 0, 0, 0], [0, 0, 0, 0, 0, 0, 0, 0, 0, 0, 0, 0, 0, 0, 0]](第一个句子和特殊符号的位置是0,第二个句子的位置是1)
special_tokens_mask : [[1, 0, 0, 0, 0, 0, 0, 0, 0, 0, 0, 0, 0, 0, 1], [1, 0, 0, 0, 0, 0, 0, 0, 0, 0, 0, 1, 1, 1, 1]]( 特殊符号的位置是1,其他位置是0)
length : [15, 12]
attention_mask : [[1, 1, 1, 1, 1, 1, 1, 1, 1, 1, 1, 1, 1, 1, 1], [1, 1, 1, 1, 1, 1, 1, 1, 1, 1, 1, 1, 0, 0, 0]]( pad的位置是0,其他位置是1)
tokenizer.decode(out[‘input_ids’][0]), tokenizer.decode(out[‘input_ids’][1])
//输出为:(‘[CLS] 选 择 珠 江 花 园 的 原 因 就 是 方 便 [SEP]’, ‘[CLS] 笔 记 本 的 键 盘 确 实 爽 。 [SEP] [PAD] [PAD] [PAD]’)
#批量编码成对的句子(tokenizer.batch_encode_plus,编码一对一对句子)
out = tokenizer.batch_encode_plus(
batch_text_or_text_pairs=[(sents[0], sents[1]), (sents[2], sents[3])],
add_special_tokens=True,
#当句子长度大于max_length时,截断
truncation=True,
#一律补零到max_length长度
padding=‘max_length’,
max_length=30,
#可取值tf,pt,np,默认为返回list
return_tensors=None,
#返回token_type_ids
return_token_type_ids=True,
#返回attention_mask
return_attention_mask=True,
#返回special_tokens_mask 特殊符号标识
return_special_tokens_mask=True,
#返回offset_mapping 标识每个词的起止位置,这个参数只能BertTokenizerFast使用
#return_offsets_mapping=True,
#返回length 标识长度
return_length=True,
)
#length 返回句子长度
for k, v in out.items():
print(k, ‘:’, v)
//输出:
input_ids : [[101, 6848, 2885, 4403, 3736, 5709, 1736, 4638, 1333, 1728, 2218, 3221, 3175, 912, 511, 102, 5011, 6381, 3315, 4638, 7241, 4669, 4802, 2141, 4272, 511, 102, 0, 0, 0], [101, 2791, 7313, 1922, 2207, 511, 1071, 800, 4638, 6963, 671, 5663, 511, 102, 791, 1921, 2798, 4761, 6887, 6821, 741, 6820, 3300, 5018, 127, 1318, 117, 4696, 3300, 102]]
token_type_ids : [[0, 0, 0, 0, 0, 0, 0, 0, 0, 0, 0, 0, 0, 0, 0, 0, 1, 1, 1, 1, 1, 1, 1, 1, 1, 1, 1, 0, 0, 0], [0, 0, 0, 0, 0, 0, 0, 0, 0, 0, 0, 0, 0, 0, 1, 1, 1, 1, 1, 1, 1, 1, 1, 1, 1, 1, 1, 1, 1, 1]]
special_tokens_mask : [[1, 0, 0, 0, 0, 0, 0, 0, 0, 0, 0, 0, 0, 0, 0, 1, 0, 0, 0, 0, 0, 0, 0, 0, 0, 0, 1, 1, 1, 1], [1, 0, 0, 0, 0, 0, 0, 0, 0, 0, 0, 0, 0, 1, 0, 0, 0, 0, 0, 0, 0, 0, 0, 0, 0, 0, 0, 0, 0, 1]]
length : [27, 30]
attention_mask : [[1, 1, 1, 1, 1, 1, 1, 1, 1, 1, 1, 1, 1, 1, 1, 1, 1, 1, 1, 1, 1, 1, 1, 1, 1, 1, 1, 0, 0, 0], [1, 1, 1, 1, 1, 1, 1, 1, 1, 1, 1, 1, 1, 1, 1, 1, 1, 1, 1, 1, 1, 1, 1, 1, 1, 1, 1, 1, 1, 1]]
tokenizer.decode(out[‘input_ids’][0])
//输出为:‘[CLS] 选 择 珠 江 花 园 的 原 因 就 是 方 便 。 [SEP] 笔 记 本 的 键 盘 确 实 爽 。 [SEP] [PAD] [PAD] [PAD]’ - 分词器词典的操作:
#获取字典
zidian = tokenizer.get_vocab()
type(zidian), len(zidian), ‘月光’ in zidian,//输出为:(dict, 21128, False)
#添加新词
tokenizer分词默认一个字一个词,所有上述代码输出字与字之间有空格表示被分割,此时可自定义词典,这样多个字之间就不会被切开
tokenizer.add_tokens(new_tokens=[‘月光’, ‘希望’])
#添加新符号
tokenizer.add_special_tokens({‘eos_token’: ‘[EOS]’})
zidian = tokenizer.get_vocab()
type(zidian), len(zidian), zidian[‘月光’], zidian[‘[EOS]’]//输出为:(dict, 21131, 21128, 21130) - 数据集:
from datasets import load_dataset
dataset = load_dataset(path=‘seamew/ChnSentiCrop’, split=“train”) //split值为train,test,返回由train_test_split切分后的结果
dataset.sort('列名‘) //根据某一列排序
dataset[‘label’][:10} //取label列第0-9的值
dataset.shuffle(seed=42) //随机打乱,seed为随机种子,是为了再现打乱的数据集用的
dataset.train_test_split(stest_size=0.1) //切分训练集与测试集
dataset.shard(num_shards=4,index=0) //将数据切分到四个桶中,均匀分配
dataset.rename_column(old_name, new_name) //列重命名
dataset.remove_column(column_name) //列删除
dataset.set_format(type=‘torch’, columns=['label])//类型转换,转换为pytorch
上述函数直接操作dataset对象,而不是返回新的的对象
dataset[‘text’].filter(fn) //起选择过滤作用,fn为自定义过滤规则的函数,比如def fn(data): return data.startswith(‘xxx’)
dataset_map = dataset.map(function) //参数为一个函数,map()函数是python内置的高阶函数,对传入的list的每一个元素进行映射,返回一个新的映射之后的list
from datasets import load_from_disk
dataset.save_to_disk(path) //保存磁盘
dataset = load_from_disk(path) //加载
dataset.to_csv(path) //保存csv
dataset.to_json(path) //保存json - 评价函数,可看github上的Huggingface_Toturials项目的代码(4.metrics.ipynb文件,地址在文章末尾)
- pipeline()管道函数, 提供了一些不需要训练模型就可以执行的nlp任务(句子情感分类,阅读理解,完形填空,文本生成(起个头,模型往后写),命名实体识别,文本总结,机器翻译等)
form transformers import pipeline
具体使用教程可在最后github中查看 - 中文分类+中文天空+中文句子关系推断(具体代码可在最后github中查看):
中文分类:对句子的情感分类只需要拿特征中的第0个词就行了
三个例子:主要是定义训练下游模型,而不需要训练预定义模型。由最后的准确率中可以看到在很块、很短的时间内就达到了70、80的准确率,这就是使用bert预训练模型的威力,在原本自然语言数据集想做一个文本分类任务,想训练到70、80的准确率,需要极大的训练量,且模型难以收敛
三、所有的NLP任务都基于bert中文模型来抽取特征,它能够极大的节约你训练的时间和精力
迁移学习举例:你已经认识了很多张图片,这时候你将100章图片放入预训练模型时,它会抽取特征,此时你再下游定义一个模型,只需要提供100照片和是不是猫两个集合,就可以训练出一个辨别是否为猫的模型(也可以为多分类)–只需要训练下游模型参数,而不需要训练预训练模型(REQUIRES_GRAD = False)
四、网址链接
-
视频地址:https://www.bilibili.com/video/BV1a44y1H7Jc/?spm_id_from=333.337.search-card.all.click&vd_source=a66e728886f37b4b360358ac380f6885
-
github地址:https://github.com/lansinuote/Huggingface_Toturials/
1.install.ipynb 安装
2.tokenizer.ipynb 字典和分词根据
3.datasets.ipynb 数据集操作
4.metrics.ipynb 评价函数的使用
5.pipeline.ipynb 管道方法的使用
7.中文分类.ipynb 实战任务1(重点)
8.中文填空.ipynb 实战任务2(重点)
9.中文句子关系推断.ipynb 实战任务3(重点)
10.trainer.ipynb
11.中文分类_CUDA.ipynb
1-5是对huggingface提供的工具集分门别类的简单快速讲解 -
huggubface网址:https://huggingface.co
更多推荐
所有评论(0)