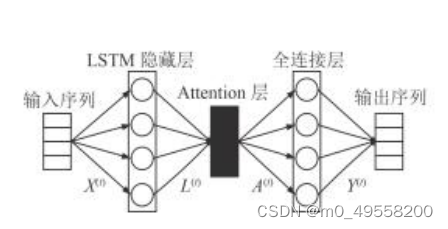
Attention-LSTM模型的python实现
1.模型结构
1.输入层
输入层是全部特征进行归一化之后的序列。归一化的目的是保证特征处于相似的尺度上,有利于加快梯度下降算法运行速度。可以使用MAX-MIN归一化的方法。归一化用EXCEL公式即可做到。
2.LSTM层
这里直接使用了keras包中自带的LSTM函数。LSTM层的代码如下所示:
inputs = tf.constant(x, dtype=tf.float32, shape=[1, 30025, 16])
# print("输入:", inputs.shape)
# print("输入:", inputs)
lstm = tf.keras.layers.LSTM(4, return_sequences=True)
output = lstm(inputs)
# print("LSTM特征提取结果:", output)#LSTM特征提取结果
###############################LSTM
这里解释一下几个参数。tf.constant函数中shape=[1,30025,16]。1表示数据的批数,30025为输入数据的条数,16为每条数据的特征数。tf.keras.layers.LSTM函数的第一个参数4表示输出向量的维数为4。
3.Attention层
输入数据 WQ、WK、WV 和矩阵相乘得到 Q,K,V。实际上就是输入数据经过线性变换得到 Q,K,V。X 为输入的数据。因此Attention层的输出向量维度也是4。设计 Attention 层时最重要的是设计权重矩阵 WQ、WK、WV。他们与输入数据决定了 Q、K、V的向量。不同的权重分配对预测有不同的效果。
Attention层的python实现如下:
def softmax(x):
x_exp = np.exp(x)
# 如果是列向量,则axis=0
x_sum = np.sum(x_exp, axis=0, keepdims=True)
s = x_exp / x_sum
return s
wq = np.array([[0.2, 0.2, 1, 0], [0.3, 0.2, 0.3, 1], [1, 0, 1, 0.1], [0.1, 0, 1, 1]])
wk = np.array([[0.3, 0, 1, 0], [0.3, 1, 0, 1], [1, 0, 1, 0], [1, 0.1, 0, 1]])
wv = np.array([[1, 0, 0.5, 0], [0, 1, 0.2, 1], [1, 0, 1, 0], [0.1, 1, 0.1, 0]]) # 权重矩阵
x = output.numpy()[0]
q = np.dot(x, wq)
# print(q)
k = np.dot(x, wk)
v = np.dot(x, wv)
a = 0
attention = []
while (a < 30025):
temp1 = np.dot(q[a], k[a].T)
temp2 = softmax(temp1) / 2
temp3 = np.dot(temp2, v[a])
attention.append(temp3)
a = a + 1
# print("attention输出",attention)#attention层输出
#################################Attention
此处wq,wk,wv三个矩阵需要自行调试。softmax函数的目的是将多分类的结果以概率的形式展现出来,是Attention层公式中的一个步骤。
计算输出结果attention矩阵时是将三万余条数据一条一条计算的,而没有将所有LSTM层的输出数据其看成一个大矩阵放入attention层进行计算。因为这样计算的复杂度太大,会报错,无法计算。
4.全连接层

def re(x):
s = 15.1 * x + 1.6
return s
input_nodes = 4
hidden_nodes = 3
output_nodes = 1
learning_rate = 0.005
n = test.neuralNetwork(input_nodes, hidden_nodes, output_nodes, learning_rate)
a = 0
while (a < 30025):
n.train(attention[a], sog[a])
a = a + 1
# 训练
a = 0
wucha = 0
yc = []
while (a < 30025):#平均误差率计算
yuce = n.query(attention[a])
yc.append(yuce)
wucha = wucha + math.fabs(re(yuce) - re(sog[a])) / (re(sog[a]) + 0.0001) # 防止分母为0 加的数字很小不影响
print(a, re(yuce), re(sog[a]), math.fabs(re(yuce) - re(sog[a])) / (re(sog[a]) + 0.0001))
a = a + 1
# 测试
#################################结束
解释代码的含义。attention数组为attention层的输出结果,一共30025条数据,每条数据4个特征。sog是实际结果的向量,共30025个数据,即期望的输出结果。第一个while循环的目的是对模型进行训练。train是定义的训练函数。
yc数组为全连接层的输出向量,也就是整个Attention-LSTM模型的输出,即预测值。要注意yc,sog,attention数组均为归一化之后的数据。
test.neuralNetwork即为上面引用的文章中的class neuralNetwork。由于本人在实现时将这个类放在了test.py文件中,因此写成test.neuralNetwork。要记得在一开头引用test.py文件,即import test。
第二个while循环用来计算误差,由于几个数组均为归一化之后的数据,故计算误差率的时候要进行反归一化。为防止0在分母,要在分母处加上一个特别小的数。
5.Attention-LSTM模型python实现的完整代码
import math
import random
import keras
import numpy
import numpy as np
import tensorflow as tf
import csv
import os
import scipy.special
import test#此处引用的文件里是全连接层的实现内容,即neuralNetwork类
import time
import matplotlib.pyplot as plt
start=time.time()
def softmax(x):
x_exp = np.exp(x)
# 如果是列向量,则axis=0
x_sum = np.sum(x_exp, axis=0, keepdims=True)
s = x_exp / x_sum
return s
def re(x):
s = 15.1 * x + 1.6
return s
os.environ['TF_CPP_MIN_LOG_LEVEL'] = '2'
x = []
with open("xxxx.csv", "r") as csvfile:#此处csv文件保存的是输入特征归一化之后的数据,即自变量
reader = csv.reader(csvfile)
print(type(reader))
for row in reader:
# print(row)
x.append(row)
for i in range(len(x)):
for j in range(len(x[i])):
x[i][j] = float(x[i][j])
# 字符串转为数字 此处取出的数据为字符串,要转化为数字
##############################取数据
inputs = tf.constant(x, dtype=tf.float32, shape=[1, 30025, 16])
# print("输入:", inputs.shape)
# print("输入:", inputs)
lstm = tf.keras.layers.LSTM(4, return_sequences=True)
output = lstm(inputs)
# print("LSTM特征提取结果:", output)#LSTM特征提取结果
###############################LSTM
wq = np.array([[0.2, 0.2, 1, 0], [0.3, 0.2, 0.3, 1], [1, 0, 1, 0.1], [0.1, 0, 1, 1]])
wk = np.array([[0.3, 0, 1, 0], [0.3, 1, 0, 1], [1, 0, 1, 0], [1, 0.1, 0, 1]])
wv = np.array([[1, 0, 0.5, 0], [0, 1, 0.2, 1], [1, 0, 1, 0], [0.1, 1, 0.1, 0]]) # 权重矩阵
x = output.numpy()[0]
q = np.dot(x, wq)
# print(q)
k = np.dot(x, wk)
v = np.dot(x, wv)
a = 0
attention = []
while (a < 30025):
temp1 = np.dot(q[a], k[a].T)
temp2 = softmax(temp1) / 2
temp3 = np.dot(temp2, v[a])
attention.append(temp3)
a = a + 1
# print("attention输出",attention)#attention层输出
#################################Attention
sog = [] # 取出归一化后的全连接层
with open("xxx.csv", "r") as csvfile:#此处取出的是实际值,即理想的输出目标
reader = csv.reader(csvfile)
sog = [row[0] for row in reader]
for i in range(len(sog)):
sog[i] = float(sog[i])
# print(sog)
#################################全连接层
input_nodes = 4
hidden_nodes = 3
output_nodes = 1
learning_rate = 0.005
n = test.neuralNetwork(input_nodes, hidden_nodes, output_nodes, learning_rate)
print(attention[0], sog[0])
a = 0
while (a < 30025):
n.train(attention[a], sog[a])
a = a + 1
# 训练
a = 0
wucha = 0
yc = []
while (a < 30025):
yuce = n.query(attention[a])
yc.append(yuce)
wucha = wucha + math.fabs(re(yuce) - re(sog[a])) / (re(sog[a]) + 0.0001) # 防止分母为0 加的数字很小不影响
print(a, re(yuce), re(sog[a]), math.fabs(re(yuce) - re(sog[a])) / (re(sog[a]) + 0.0001))
a = a + 1
# 测试
#################################结束
end=time.time()
print(end-start)#计算了运行时间
此模型最复杂的是模型中各个参数的选取,只能靠手动调试。本文题的输入特征数为16个,输出为1个。部分参数的选择如下所示:
三个权重矩阵的选取如下:
最终的平均预测误差为10%左右,如果参数继续优化,误差会更小。
更多推荐
所有评论(0)