c++ opencv 图像处理:直方图处理(直方图均衡化,直方图匹配(规定化))

文章目录
前言
数字图像处理c++ opencv(VS2019 opencv4.53)持续更新
一、直方图(histogram)
非归一化直方图:
h
(
r
k
)
=
n
k
h(r_k)=n_k
h(rk)=nk
其中
r
k
r_k
rk为图像像素灰度值,比如常见的0-255,
n
k
n_k
nk为图像中某一灰度级的像素个数。
归一化直方图:
p
(
r
k
)
=
h
(
r
k
)
M
N
=
n
k
M
N
p(r_k)=\frac{h(r_k)}{MN}=\frac{n_k}{MN}
p(rk)=MNh(rk)=MNnk
其中MN为图像行数和列数,常说的图像直方图就是归一化直方图。
直方图的现状表现了图像的外观:
图摘自数字图像处理第四版,直方图分布较广较均匀的图像对比度高,视觉效果好。
获取图像直方图示例:
代码如下(示例):
#include<iostream>
#include<opencv2/opencv.hpp>
using namespace cv;
using namespace std;
int main()
{
Mat image, image_gray, hist; //定义输入图像,灰度图像, 直方图
image = imread("lena.png"); //读取图像;
if (image.empty())
{
cout << "读取错误" << endl;
return -1;
}
cvtColor(image, image_gray, COLOR_BGR2GRAY); //灰度化
imshow(" image_gray", image_gray); //显示灰度图像
//获取图像直方图
int histsize = 256;
float ranges[] = { 0,256 };
const float* histRanges = { ranges };
calcHist(&image_gray, 1, 0, Mat(), hist, 1, &histsize, &histRanges, true, false);
//创建直方图显示图像
int hist_h = 300;//直方图的图像的高
int hist_w = 512; //直方图的图像的宽
int bin_w = hist_w / histsize;//直方图的等级
Mat histImage(hist_h, hist_w, CV_8UC3, Scalar(0, 0, 0));//绘制直方图显示的图像
//绘制并显示直方图
normalize(hist, hist, 0, hist_h, NORM_MINMAX, -1, Mat());//归一化直方图
for (int i = 1; i < histsize; i++)
{
line(histImage, Point((i - 1) * bin_w, hist_h - cvRound(hist.at<float>(i - 1))),
Point((i)*bin_w, hist_h - cvRound(hist.at<float>(i))), Scalar(255, 0, 0), 2, 8, 0);
}
imshow("histImage", histImage);
waitKey(0); //暂停,保持图像显示,等待按键结束
return 0;
}
}
结果:
二、直方图处理
1.直方图均衡化
(1)均衡化原理
目的:通过均衡化处理使得输入图像的直方图变得范围较广以及分布较均匀。
原理公式:
s
k
=
(
L
−
1
)
∑
j
=
0
k
P
r
(
r
j
)
,
k
=
0
,
1
,
.
.
.
,
L
−
1
s_k=(L-1)\sum_{j=0}^k P_r(r_j), k=0,1,...,L-1
sk=(L−1)j=0∑kPr(rj),k=0,1,...,L−1
如:
s
1
=
(
L
−
1
)
P
r
(
1
)
s_1=(L-1)P_r(1)
s1=(L−1)Pr(1)
s
2
=
(
L
−
1
)
(
P
r
(
1
)
+
P
r
(
2
)
)
s_2=(L-1)(P_r(1)+P_r(2) )
s2=(L−1)(Pr(1)+Pr(2))
s
L
−
1
=
(
L
−
1
)
(
P
r
(
1
)
+
P
r
(
2
)
+
.
.
.
+
P
r
(
L
−
1
)
)
=
L
−
1
s_{L-1}=(L-1)(P_r(1)+P_r(2) +...+P_r(L-1))=L-1
sL−1=(L−1)(Pr(1)+Pr(2)+...+Pr(L−1))=L−1
直方图均衡化就是将图像的直方图以上图中右图为目标进行转变。不管原图的直方图现状,直方图均衡化处理后都能达到右边的效果。
(2)代码如下:
using namespace cv;
using namespace std;
int main()
{
Mat image, image_gray, image_enhanced; //定义输入图像,灰度图像, 直方图
image = imread("lena.png"); //读取图像;
if (image.empty())
{
cout << "读取错误" << endl;
return -1;
}
cvtColor(image, image_gray, COLOR_BGR2GRAY); //灰度化
imshow(" image_gray", image_gray); //显示灰度图像
equalizeHist(image_gray, image_enhanced);//直方图均衡化
imshow(" image_enhanced", image_enhanced); //显示增强图像
waitKey(0); //暂停,保持图像显示,等待按键结束
return 0;
}
(3)结果:
2.直方图匹配(规定化)
(1)直方图匹配(规定化)原理
从字面意思理解,就是为需要处理的图像匹配另一幅图的直方图形状或规定一个目标直方图形状。比如前面的直方图均衡化处理也可以理解为特殊的直方图匹配,匹配目标直方图为:
类似直方图均衡化,以其他直方图形状为目标的处理为直方图匹配(规定)。
直方图匹配原理(个人理解):
前面的直方图均衡化提到:不管原图像的直方图是什么形状,直方图均衡化后的直方图都是一样的,如下图:
这里的映射函数规定为单调递增的,即变换后的直方图可以经过逆变换回到原直方图。那么直方图匹配就可以根据这个性质进行处理,示意图如下:
内容相同的图像,直方图均衡化后的直方图相似。想要a图所示的直方图转变到c图,那么可以有:
因此,直方图匹配的步骤为:
1,计算输入图像的直方图
P
(
r
)
P(r)
P(r),并进行直方图均衡化,得到均衡化后的灰度
s
k
,
k
=
1
,
2...
L
−
1
s_k,k=1,2...L-1
sk,k=1,2...L−1.(直方图a到直方图b)
2,根据
G
(
z
q
)
=
(
L
−
1
)
∑
i
=
0
q
P
z
(
z
j
)
G(z_q)=(L-1)\sum _{i=0} ^q P_z(z_j)
G(zq)=(L−1)i=0∑qPz(zj)计算
G
(
z
q
)
G(z_q)
G(zq)所有值,并存储到一个查找表中。(记录z与G的映射,z与G一一对应,逆变换就可以直接查对应的值)
3,对
s
k
,
k
=
1
,
2...
L
−
1
s_k,k=1,2...L-1
sk,k=1,2...L−1的每个值,用步骤2得到的表找到
z
q
z_q
zq对应的值,使得
G
(
z
q
)
G(z_q)
G(zq)最接近
s
k
s_k
sk,并存储s到z的映射。(逆变换)
4,使用步骤3找到的映射,将
s
k
s_k
sk值映射到直方图指定图像中值为
z
q
z_q
zq的对应像素,形成直方图指定图像。
(2)示例1
如下图将一个64*64大小的图进行直方图规定化
第一步:得到原图均衡化后的值并四舍五入
根据公式:
s
k
=
(
L
−
1
)
∑
j
=
0
k
P
r
(
r
j
)
,
k
=
0
,
1
,
.
.
.
,
L
−
1
s_k=(L-1)\sum_{j=0}^k P_r(r_j), k=0,1,...,L-1
sk=(L−1)j=0∑kPr(rj),k=0,1,...,L−1
有:
s
0
=
7
∗
∑
j
=
0
0
P
r
(
r
j
)
=
1.33
−
−
1
s_0=7*\sum_{j=0}^0 P_r(r_j)= 1.33--1
s0=7∗j=0∑0Pr(rj)=1.33−−1
s
1
=
7
∗
∑
j
=
0
1
P
r
(
r
j
)
=
3.08
−
−
3
s_1=7*\sum_{j=0}^1 P_r(r_j)= 3.08--3
s1=7∗j=0∑1Pr(rj)=3.08−−3
最后有:
第二步:使用 p z p_z pz计算G(z)的值
根据公式:
G
(
z
q
)
=
(
L
−
1
)
∑
i
=
0
q
P
z
(
z
j
)
G(z_q)=(L-1)\sum _{i=0} ^q P_z(z_j)
G(zq)=(L−1)i=0∑qPz(zj)
有:
G
(
z
0
)
=
7
∗
∑
i
=
0
0
P
z
(
z
i
)
=
0
−
−
0
G(z_0)=7*\sum_{i=0}^0 P_z(z_i)= 0--0
G(z0)=7∗i=0∑0Pz(zi)=0−−0
最终有:
第三步:找
s
k
s_k
sk和
z
q
z_q
zq的映射(G的逆变换)
将原
s
k
s_k
sk的改为映射的值后的值有:
(3)示例2
以下图为例,将上面的直方图向下面的直方图进行转变
代码如下
#include<iostream>
#include<opencv2/opencv.hpp>
using namespace cv;
using namespace std;
int main()
{
Mat image1, image1_gray, hist1, image2, image2_gray, hist2, image_enhanced; //定义修改图像,灰度修改图像, 修改直方图,目标图像,灰度目标图像,目标直方图, 规定化增强图像
image1 = imread("lena.png"); //读取图像;
if (image1.empty())
{
cout << "读取错误" << endl;
return -1;
}
cvtColor(image1, image1_gray, COLOR_BGR2GRAY); //灰度化
imshow(" image1_gray", image1_gray); //显示灰度图像
image2 = imread("1043.jpg"); //读取图像;
if (image2.empty())
{
cout << "读取错误" << endl;
return -1;
}
cvtColor(image2, image2_gray, COLOR_BGR2GRAY); //灰度化
imshow(" image2_gray", image2_gray); //显示灰度图像
//均衡化处理
equalizeHist(image1_gray, image1_gray);
equalizeHist(image2_gray, image2_gray);
//获取两个均衡化图像的直方图
int histsize = 256;
float ranges[] = { 0,256 };
const float* histRanges = { ranges };
calcHist(&image1_gray, 1, 0, Mat(), hist1, 1, &histsize, &histRanges, true, false);
calcHist(&image2_gray, 1, 0, Mat(), hist2, 1, &histsize, &histRanges, true, false);
//计算两个均衡化图像直方图的累积概率
float hist1_cdf[256] = { hist1.at<float>(0) };
float hist2_cdf[256] = { hist2.at<float>(0) };
for (int i = 1; i < 256; i++)
{
hist1_cdf[i] = (hist1_cdf[i - 1] + hist1.at<float>(i)) ;
hist2_cdf[i] = (hist2_cdf[i - 1] + hist2.at<float>(i)) ;
}
for (int i = 0; i < 256; i++)
{
hist1_cdf[i] = hist1_cdf[i] / (image1_gray.rows * image1_gray.cols);
hist2_cdf[i] = hist2_cdf[i] / (image2_gray.rows * image2_gray.cols);
}
// 两个累计概率之间的差值,用于找到最接近的点
float diff_cdf[256][256];
for (int i = 0; i < 256; i++) {
for (int j = 0; j < 256; j++)
{
diff_cdf[i][j] = fabs(hist1_cdf[i] - hist2_cdf[j]);
}
}
Mat lut(1, 256, CV_8U);
for (int i = 0; i < 256; i++)
{
//查找源灰度级为i的映射灰度和i的累积概率差最小(灰度接近)的规定化灰度
float min = diff_cdf[i][0];
int index = 0;
for (int j = 0; j < 256; j++) {
if (min > diff_cdf[i][j]) {
min = diff_cdf[i][j];
index = j;
}
}
lut.at<uchar>(i) = index;
}
LUT(image1_gray, lut, image_enhanced); //图像中进行映射
imshow("image_enhanced", image_enhanced);
waitKey(0); //暂停,保持图像显示,等待按键结束
return 0;
}
结果:
三、opencv函数总结
1.equalizeHist图像均衡化
equalizeHist(image1_gray, image1_gray);
2.calcHist获取图像直方图
int histsize = 256;
float ranges[] = { 0,256 };
const float* histRanges = { ranges };
calcHist(&image1_gray, 1, 0, Mat(), hist1, 1, &histsize, &histRanges, true, false);
3.LUT数据映射
LUT(image1_gray, lut, image_enhanced); //图像中进行映射
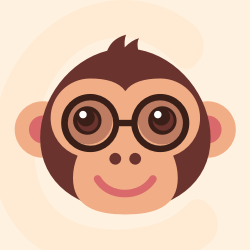



更多推荐
所有评论(0)