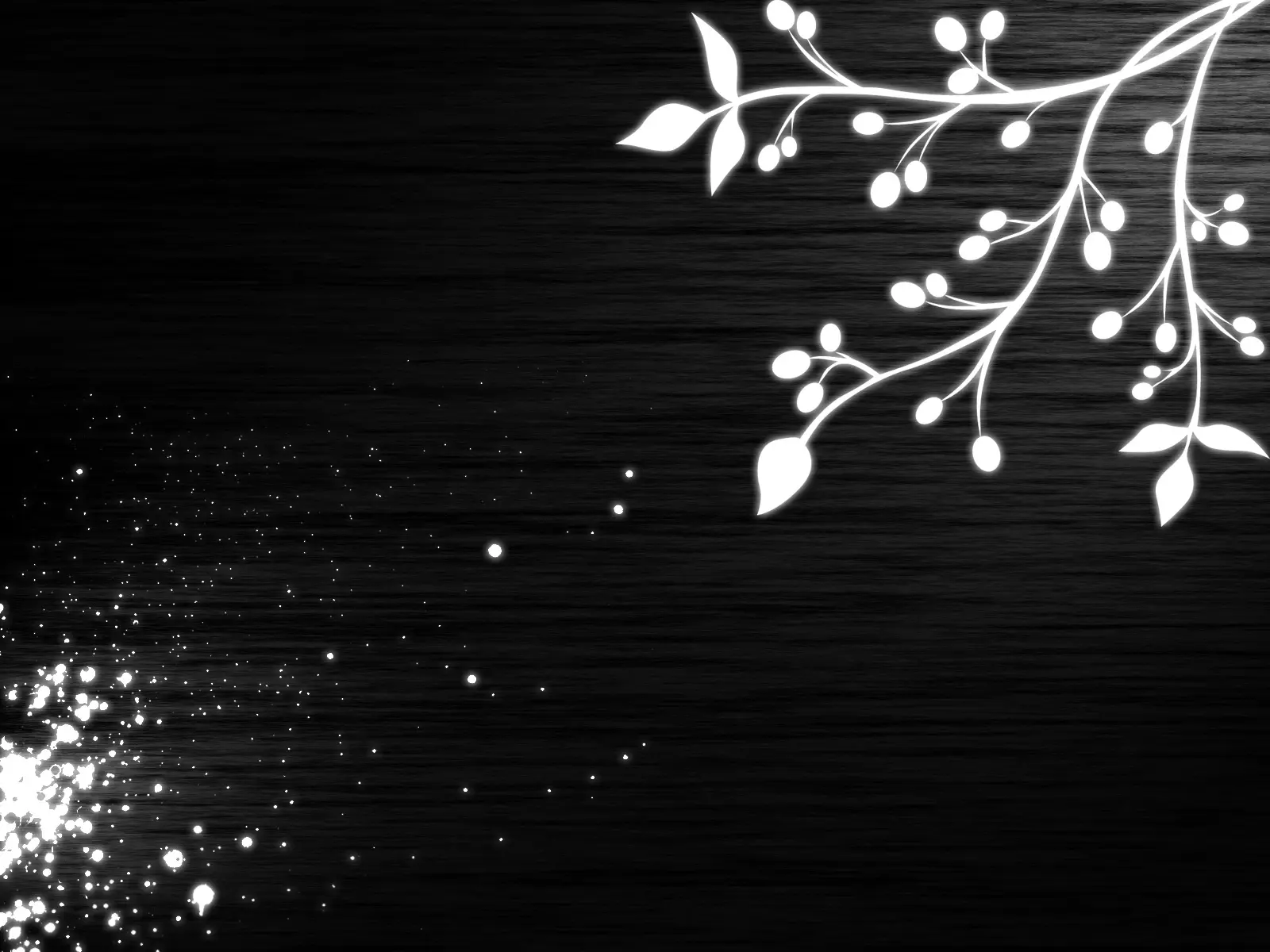
catboost参数详解及实战(强推)
·
目录
原理部分看这里:Catboost原理详解_Python风控模型与数据分析的博客-CSDN博客
一 参数详解
由于catboost参数较多,本文仅列出重要及常用参数(如需直接使用,可将 :替换为 # )
'''
公共参数
'''
params={
'loss_function': , : 损失函数,取值RMSE, Logloss, MAE, CrossEntropy, Quantile, LogLinQuantile, Multiclass, MultiClassOneVsAll, MAPE, Poisson。默认Logloss。
'custom_loss': , : 训练过程中计算显示的损失函数,取值Logloss、CrossEntropy、Precision、Recall、F、F1、BalancedAccuracy、AUC等等
'eval_metric': , : 用于过度拟合检测和最佳模型选择的指标,取值范围同custom_loss
'iterations': , : 最大迭代次数,默认500. 别名:num_boost_round, n_estimators, num_trees
'learning_rate': , : 学习速率,默认0.03 别名:eta
'random_seed': , : 训练的随机种子,别名:random_state
'l2_leaf_reg': , : l2正则项,别名:reg_lambda
'bootstrap_type': , : 确定抽样时的样本权重,取值Bayesian、Bernoulli(伯努利实验)、MVS(仅支持cpu)、Poisson(仅支持gpu)、No(取值为No时,每棵树为简单随机抽样);默认值GPU下为Bayesian、CPU下为MVS
'bagging_temperature': , : bootstrap_type=Bayesian时使用,取值为1时采样权重服从指数分布;取值为0时所有采样权重均等于1。取值范围[0,inf),值越大、bagging就越激进
'subsample': , : 样本采样比率(行采样)
'sampling_frequency': , : 采样频率,取值PerTree(在构建每棵新树之前采样)、PerTreeLevel(默认值,在子树的每次分裂之前采样);仅支持CPU
'random_strength': , : 设置特征分裂信息增益的扰动项,用于避免过拟合。子树分裂时,正常会寻找最大信息增益的特征+分裂点进行分裂,此处对每个特征+分裂点的信息增益值+扰动项后再确定最大值。扰动项服从正态分布、均值为0,random_strength参数值会作为正态分布的方差,默认值1、对应标准正态分布;设置0时则无扰动项
'use_best_model': , : 让模型使用效果最优的子树棵树/迭代次数,使用验证集的最优效果对应的迭代次数(eval_metric:评估指标,eval_set:验证集数据),布尔类型可取值0,1(取1时要求设置验证集数据)
'best_model_min_trees': , : 最少子树棵树,和use_best_model一起使用
'depth': , : 树深,默认值6
'grow_policy': , : 子树生长策略,取值SymmetricTree(默认值,对称树)、Depthwise(整层生长,同xgb)、Lossguide(叶子结点生长,同lgb)
'min_data_in_leaf': , : 叶子结点最小样本量
'max_leaves': , : 最大叶子结点数量
'one_hot_max_size': , : 对唯一值数量<one_hot_max_size的类别型特征使用one-hot编码
'rsm': , : 列采样比率,别名colsample_bylevel 取值(0,1],默认值1
'nan_mode': , : 缺失值处理方法,取值Forbidden(不支持缺失值,输入包含缺失时会报错)、Min(处理为该列的最小值,比原最小值更小)、Max(同理)
'input_borders': , : 特征数据边界(最大最小边界)、会影响缺失值的处理(nan_mode取值Min、Max时),默认值None、在训练时特征取值的最大最小值即为特征值边界
'class_weights': , : y标签类别权重、用于类别不均衡处理,默认各类权重均为1
'auto_class_weights': , : 自动计算平衡各类别权重
'scale_pos_weight': , : 二分类中第1类的权重,默认值1(不可与class_weights、auto_class_weights同时设置)
'boosting_type': , : 提升类型,取值Ordered(catboost特有的排序提升,在小数据集上效果可能更好,但是运行速度较慢)、Plain(经典提升)
'feature_weights': , : 特征权重,在子树分裂时计算各特征的信息增益✖️该特征权重,选取最大结果对应特征分裂;设置方式:1、feature_weights = [0.1, 1, 3];2、feature_weights = {"Feature2":1.1,"Feature4":0.3}
}
'''
category参数
'''
params={
'max_ctr_complexity': , : 分类特征交叉的最高阶数,默认值4
}
'''
output 参数
'''
params={
'logging_level': , : 模型训练过程的信息输出等级,取值Silent(不输出信息)、Verbose(默认值,输出评估指标、已训练时间、剩余时间等)、Info(输出额外信息、树的棵树)、Debug(debug信息)
'metric_period': , : 计算目标值、评估指标的频率,默认值1、即每次迭代都输出目标值、评估指标
'verbose': , : 输出日记信息等级,类似于logging_level(两者只设置一个),取值True对应上方Verbose、False对应Silent
}
'''
过拟合检测设置
'''
params={
'early_stopping_rounds': , : 设置提前停止训练,在得到最佳的评估结果后、再迭代n(参数值为n)次停止训练,默认值不启用
'od_type': , : 过拟合检测类型,取值IncToDec(默认值)、Iter
'od_pval': , : IncToDec过拟合检测的阈值,当达到指定值时,训练将停止。要求输入验证数据集,建议取值范围[10e-10,10e-2s],默认值0、不使用过拟合检测
'od_wait': , : 与early_stopping_rounds部分相似,od_wait为达到最佳评估值后继续迭代的次数,检测器为IncToDec时达到最佳评估值后继续迭代n次(n为od_wait参数值);检测器为Iter时达到最优评估值后停止,默认值20
}
'''
设备类型参数
'''
params={
'task_type': , : 模型训练的处理单元类型,取值CPU(默认)、GPU
'devices': , : GPU设备id
}
'''
数值型变量分箱设置参数
'''
params={
'border_count': , : 数值型特征的分箱数,别名max_bin,取值范围[1,65535]、默认值254(CPU下)
'feature_border_type': , : 数值型特征的分箱方法,取值Median、Uniform、UniformAndQuantiles、MaxLogSum、MinEntropy、GreedyLogSum(默认值)
}
'''
文本型变量设置参数
'''
params={
'tokenizers': , : 分词器,如果给出一个分词器、三个字典和两个特征,则为每个原始文本功能总共创建了6组新的功能(1⋅3⋅2=6)。
'''
设置示例:
tokenizers = [{
'tokenizerId': 'Space',
'delimiter': ' ',
'separator_type': 'ByDelimiter',
},{
'tokenizerId': 'Sense',
'separator_type': 'BySense',
}]
'''
'dictionaries': , : 预处理文本型特征的参数字典,
'feature_calcers': , : 文本型特征名的列表
'text_processing': , : 文本型特征完整参数设置,仅设置该参数或设置上三个参数
}
二 实战
1 导包
import re
import os
import pandas as pd
import numpy as np
import warnings
warnings.filterwarnings('ignore')
import sklearn
from sklearn.model_selection import train_test_split
from sklearn.metrics import roc_curve,roc_auc_score
import matplotlib.pyplot as plt
import gc
from bayes_opt import BayesianOptimization
from catboost import Pool, cv
2 数据读取
df=pd.read_csv('E:/train.csv',engine='python').head(80000)
print(df.shape)
df.head()
3 贷后y标签分布,逾期率20%
pd.concat([df_copy['isDefault'].value_counts()
,df_copy['isDefault'].value_counts(normalize=True)],axis=1)
4 预处理
employmentLength字段为工作年限,提取出年数
df_copy['employmentLength']=df_copy['employmentLength'].replace(' years','')
dic={'< 1':0,'10+':20}
df_copy['employmentLength']=df_copy['employmentLength'].map(dic).astype('float')
5 特征分布
import seaborn as sns
sns.pairplot(df_copy.loc[:,'loanAmnt':'isDefault'].drop(['issueDate'],axis=1)
, kind="scatter",hue="isDefault"
, plot_kws=dict(s=80, edgecolor="white", linewidth=2.5))
6 特征分组
float_col=list(df_copy.select_dtypes(exclude=['string','object']).drop(['id','isDefault'],axis=1).columns).copy()
cate_col=['grade', 'subGrade']
all_fea=float_col+cate_col
7 初始参数
params={
'loss_function': 'Logloss', # 损失函数,取值RMSE, Logloss, MAE, CrossEntropy, Quantile, LogLinQuantile, Multiclass, MultiClassOneVsAll, MAPE, Poisson。默认Logloss。
'custom_loss': 'AUC', # 训练过程中计算显示的损失函数,取值Logloss、CrossEntropy、Precision、Recall、F、F1、BalancedAccuracy、AUC等等
'eval_metric': 'AUC', # 用于过度拟合检测和最佳模型选择的指标,取值范围同custom_loss
'iterations': 50, # 最大迭代次数,默认500. 别名:num_boost_round, n_estimators, num_trees
'learning_rate': 0.1, # 学习速率,默认0.03 别名:eta
'random_seed': 123, # 训练的随机种子,别名:random_state
'l2_leaf_reg': 5, # l2正则项,别名:reg_lambda
'bootstrap_type': 'Bernoulli', # 确定抽样时的样本权重,取值Bayesian、Bernoulli(伯努利实验)、MVS(仅支持cpu)、Poisson(仅支持gpu)、No(取值为No时,每棵树为简单随机抽样);默认值GPU下为Bayesian、CPU下为MVS
# 'bagging_temperature': 0, # bootstrap_type=Bayesian时使用,取值为1时采样权重服从指数分布;取值为0时所有采样权重均等于1。取值范围[0,inf),值越大、bagging就越激进
'subsample': 0.6, # 样本采样比率(行采样)
'sampling_frequency': 'PerTree', # 采样频率,取值PerTree(在构建每棵新树之前采样)、PerTreeLevel(默认值,在子树的每次分裂之前采样);仅支持CPU
'use_best_model': True, # 让模型使用效果最优的子树棵树/迭代次数,使用验证集的最优效果对应的迭代次数(eval_metric:评估指标,eval_set:验证集数据),布尔类型可取值0,1(取1时要求设置验证集数据)
'best_model_min_trees': 50, # 最少子树棵树,和use_best_model一起使用
'depth': 4, # 树深,默认值6
'grow_policy': 'SymmetricTree', # 子树生长策略,取值SymmetricTree(默认值,对称树)、Depthwise(整层生长,同xgb)、Lossguide(叶子结点生长,同lgb)
'min_data_in_leaf': 500, # 叶子结点最小样本量
# 'max_leaves': 12, # 最大叶子结点数量
'one_hot_max_size': 4, # 对唯一值数量<one_hot_max_size的类别型特征使用one-hot编码
'rsm': 0.6, # 列采样比率,别名colsample_bylevel 取值(0,1],默认值1
'nan_mode': 'Max', # 缺失值处理方法,取值Forbidden(不支持缺失值,输入包含缺失时会报错)、Min(处理为该列的最小值,比原最小值更小)、Max(同理)
'input_borders': None, # 特征数据边界(最大最小边界)、会影响缺失值的处理(nan_mode取值Min、Max时),默认值None、在训练时特征取值的最大最小值即为特征值边界
'boosting_type': 'Ordered', # 提升类型,取值Ordered(catboost特有的排序提升,在小数据集上效果可能更好,但是运行速度较慢)、Plain(经典提升)
'max_ctr_complexity': 2, # 分类特征交叉的最高阶数,默认值4
'logging_level':'Verbose', # 模型训练过程的信息输出等级,取值Silent(不输出信息)、Verbose(默认值,输出评估指标、已训练时间、剩余时间等)、Info(输出额外信息、树的棵树)、Debug(debug信息)
'metric_period': 1, # 计算目标值、评估指标的频率,默认值1、即每次迭代都输出目标值、评估指标
'early_stopping_rounds': 20,
'border_count': 254, # 数值型特征的分箱数,别名max_bin,取值范围[1,65535]、默认值254(CPU下), # 设置提前停止训练,在得到最佳的评估结果后、再迭代n(参数值为n)次停止训练,默认值不启用
'feature_border_type': 'GreedyLogSum', # 数值型特征的分箱方法,取值Median、Uniform、UniformAndQuantiles、MaxLogSum、MinEntropy、GreedyLogSum(默认值)
}
8 catboost建模函数
import catboost
from catboost import CatBoostClassifier
def catboost_model(df,y_name,params,cate_col=[]):
x_train,x_test, y_train, y_test =train_test_split(df.drop(y_name,axis=1),df[y_name],test_size=0.2, random_state=123)
model = CatBoostClassifier(**params)
model.fit(x_train, y_train,eval_set=[(x_train, y_train),(x_test,y_test)],cat_features=cate_col)
train_pred = [pred[1] for pred in model.predict_proba(x_train)]
train_auc= roc_auc_score(list(y_train),train_pred)
test_pred = [pred[1] for pred in model.predict_proba(x_test)]
test_auc= roc_auc_score(list(y_test),test_pred)
result={
'train_auc':train_auc,
'test_auc':test_auc,
}
return model,result
9 初始模型
model,model_result=catboost_model(df_copy[all_fea+['isDefault']]
,'isDefault',params,cate_col)
10 特征重要性
def feature_importance_catboost(model):
result=pd.DataFrame(model.get_feature_importance(),index=model.feature_names_,columns=['FeatureImportance'])
return result.sort_values('FeatureImportance',ascending=False)
feature_importance_catboost(model)
11 贝叶斯调参
(1)自定义调参目标,此处使用测试集的AUC值为调参目标
def catboost_cv(iterations,learning_rate,depth,subsample,rsm):
params={
'loss_function': 'Logloss', # 损失函数,取值RMSE, Logloss, MAE, CrossEntropy, Quantile, LogLinQuantile, Multiclass, MultiClassOneVsAll, MAPE, Poisson。默认Logloss。
'custom_loss': 'AUC', # 训练过程中计算显示的损失函数,取值Logloss、CrossEntropy、Precision、Recall、F、F1、BalancedAccuracy、AUC等等
'eval_metric': 'AUC', # 用于过度拟合检测和最佳模型选择的指标,取值范围同custom_loss
'iterations': 50, # 最大迭代次数,默认500. 别名:num_boost_round, n_estimators, num_trees
'learning_rate': 0.1, # 学习速率,默认0.03 别名:eta
'random_seed': 123, # 训练的随机种子,别名:random_state
'l2_leaf_reg': 5, # l2正则项,别名:reg_lambda
'bootstrap_type': 'Bernoulli', # 确定抽样时的样本权重,取值Bayesian、Bernoulli(伯努利实验)、MVS(仅支持cpu)、Poisson(仅支持gpu)、No(取值为No时,每棵树为简单随机抽样);默认值GPU下为Bayesian、CPU下为MVS
# 'bagging_temperature': 0, # bootstrap_type=Bayesian时使用,取值为1时采样权重服从指数分布;取值为0时所有采样权重均等于1。取值范围[0,inf),值越大、bagging就越激进
'subsample': 0.6, # 样本采样比率(行采样)
'sampling_frequency': 'PerTree', # 采样频率,取值PerTree(在构建每棵新树之前采样)、PerTreeLevel(默认值,在子树的每次分裂之前采样);仅支持CPU
'use_best_model': True, # 让模型使用效果最优的子树棵树/迭代次数,使用验证集的最优效果对应的迭代次数(eval_metric:评估指标,eval_set:验证集数据),布尔类型可取值0,1(取1时要求设置验证集数据)
'best_model_min_trees': 50, # 最少子树棵树,和use_best_model一起使用
'depth': 4, # 树深,默认值6
'grow_policy': 'SymmetricTree', # 子树生长策略,取值SymmetricTree(默认值,对称树)、Depthwise(整层生长,同xgb)、Lossguide(叶子结点生长,同lgb)
'min_data_in_leaf': 500, # 叶子结点最小样本量
# 'max_leaves': 12, # 最大叶子结点数量
'one_hot_max_size': 4, # 对唯一值数量<one_hot_max_size的类别型特征使用one-hot编码
'rsm': 0.6, # 列采样比率,别名colsample_bylevel 取值(0,1],默认值1
'nan_mode': 'Max', # 缺失值处理方法,取值Forbidden(不支持缺失值,输入包含缺失时会报错)、Min(处理为该列的最小值,比原最小值更小)、Max(同理)
'input_borders': None, # 特征数据边界(最大最小边界)、会影响缺失值的处理(nan_mode取值Min、Max时),默认值None、在训练时特征取值的最大最小值即为特征值边界
'boosting_type': 'Ordered', # 提升类型,取值Ordered(catboost特有的排序提升,在小数据集上效果可能更好,但是运行速度较慢)、Plain(经典提升)
'max_ctr_complexity': 2, # 分类特征交叉的最高阶数,默认值4
'logging_level':'Silent', # 模型训练过程的信息输出等级,取值Silent(不输出信息)、Verbose(默认值,输出评估指标、已训练时间、剩余时间等)、Info(输出额外信息、树的棵树)、Debug(debug信息)
'metric_period': 1, # 计算目标值、评估指标的频率,默认值1、即每次迭代都输出目标值、评估指标
'early_stopping_rounds': 20,
'border_count': 254, # 数值型特征的分箱数,别名max_bin,取值范围[1,65535]、默认值254(CPU下), # 设置提前停止训练,在得到最佳的评估结果后、再迭代n(参数值为n)次停止训练,默认值不启用
'feature_border_type': 'GreedyLogSum', # 数值型特征的分箱方法,取值Median、Uniform、UniformAndQuantiles、MaxLogSum、MinEntropy、GreedyLogSum(默认值)
# 'cat_features':cate_col
}
params.update({'iterations':int(iterations),'depth':int(depth),'learning_rate':learning_rate,'subsample':subsample,'rsm':rsm})
# model = CatBoostClassifier(**params)
# cv_result=cross_validate(model,df_copy[all_fea],df_copy['isDefault'], cv=5,scoring='roc_auc',return_train_score=True)
model,result=catboost_model(df_copy[all_fea+['isDefault']],'isDefault',params,cate_col)
return result.get('test_auc')
(2)调参
param_value_dics={
'iterations':(20, 50),
'learning_rate':(0.02,0.2),
'depth':(3, 6),
'subsample':(0.6, 1.0),
'rsm':(0.6, 1.0)
}
cat_bayes = BayesianOptimization(
catboost_cv,
param_value_dics
)
cat_bayes.maximize(init_points=1,n_iter=20) #init_points-调参基准点,n_iter-迭代次数
cat_bayes.max.get('params')
(3)设置最优参数并重新训练模型
cat_bayes.max.get('params')
params.update(
{
'depth': 5,
'iterations': 45,
'learning_rate': 0.189,
'rsm': 0.707,
'subsample': 0.890
}
)
model,model_result=catboost_model(df_copy[all_fea+['isDefault']],'isDefault',params,cate_col)
model_result
划重点
获取数据及完整代码,关注威信公众号Python风控模型与数据分析、回复catboost实战获取
更多推荐
所有评论(0)