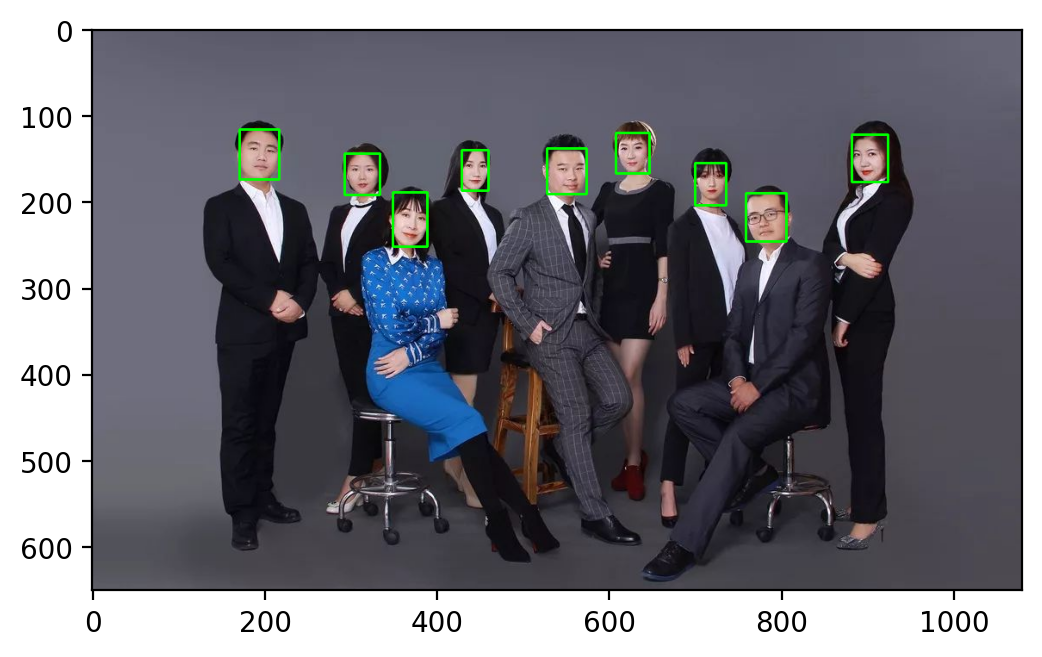
人脸检测5种方法
·
众所周知,人脸识别是计算机视觉应用的一个重大领域,在学习人脸识别之前,我们先来简单学习下人脸检测的几种用法。
常见的人脸检测方法大致有5种,Haar、Hog、CNN、SSD、MTCNN:
注:本文章图片来源于网络
相关构造检测器的文件:opencv/data at master · opencv/opencv · GitHub
基本步骤
- 读入图片
- 构造检测器
- 获取检测结果
- 解析检测结果
一、Haar
# 调整参数
img = cv2.imread('./images/001.jpg')
cv_show('img',img)
# 构造harr检测器
face_detector = cv2.CascadeClassifier('./weights/haarcascade_frontalface_default.xml')
# 转为灰度图
img_gray = cv2.cvtColor(img,cv2.COLOR_BGR2GRAY)
plt.imshow(img_gray,'gray')
# 检测结果 上图4个人脸所以4个方框坐标
# image
# scaleFactor控制人脸尺寸 默认1.1
detections = face_detector.detectMultiScale(img_gray,scaleFactor=1.3)
# 解析
for x,y,w,h in detections:
cv2.rectangle(img,(x,y),(x+w,y+h),(0,255,0))
plt.imshow(cv2.cvtColor(img,cv2.COLOR_BGR2RGB))
# 调整参数
img = cv2.imread('./images/004.jpeg')
cv_show('img',img)
# 构造harr检测器
face_detector = cv2.CascadeClassifier('./weights/haarcascade_frontalface_default.xml')
# 转为灰度图
img_gray = cv2.cvtColor(img,cv2.COLOR_BGR2GRAY)
plt.imshow(img_gray,'gray')
# 检测结果 上图4个人脸所以4个方框坐标
# image
# scaleFactor控制人脸尺寸 默认1.1
# minNeighbors 确定一个人脸框至少要有n个候选值 越高 质量越好
# [, flags[,
# minSize maxSize 人脸框的最大最小尺寸 如minSize=(40,40)
detections = face_detector.detectMultiScale(img_gray,scaleFactor=1.2, minNeighbors=10)# 在质量和数量上平衡
# 解析
for x,y,w,h in detections:
cv2.rectangle(img,(x,y),(x+w,y+h),(0,255,0))
plt.imshow(cv2.cvtColor(img,cv2.COLOR_BGR2RGB))
上述过程中:
- scaleFactor参数:用来控制人脸框的大小,可以用它来排除一些错误检测;
- minNeighbors参数:我们给人脸框起来的时候,一般一张脸会框许多的框,假如这张脸框得越多,说明质量越好,越是一张正确的“脸”。
二、Hog
对于第一次使用这个功能的同学,要提前下载一下dlib。
import dlib
# 构造HOG人脸检测器 不需要参数
hog_face_detetor = dlib.get_frontal_face_detector()
# 检测人脸获取数据
# img
# scale类似haar的scalFactor
detections = hog_face_detetor(img,1)
# 解析获取的数据
for face in detections:
# 左上角
x = face.left()
y = face.top()
# 右下角
r = face.right()
b = face.bottom()
cv2.rectangle(img,(x,y),(r,b),(0,255,0))
plt.imshow(cv2.cvtColor(img,cv2.COLOR_BGR2RGB))
三、CNN
import dlib
# 构造CNN人脸检测器
cnn_face_detector = dlib.cnn_face_detection_model_v1("./weights/mmod_human_face_detector.dat")
# 检测人脸 参数与上一种相似
detections = cnn_face_detector(img,1)
for face in detections:
# 左上角
x = face.rect.left()
y = face.rect.top()
# 右下角
r = face.rect.right()
b = face.rect.bottom()
# 置信度
c = face.confidence
print(c)
cv2.rectangle(img,(x,y),(r,b),(0,255,0))
plt.imshow(cv2.cvtColor(img,cv2.COLOR_BGR2RGB))
通过神经网络完成,这个过程中我们还可以查看每张脸检测时的置信度。
四、SSD
# 加载模型
face_detector = cv2.dnn.readNetFromCaffe('./weights/deploy.prototxt.txt','./weights/res10_300x300_ssd_iter_140000.caffemodel')
# 原图尺寸
img_height = img.shape[0]
img_width = img.shape[1]
# 放缩至输入尺寸
img_resized = cv2.resize(img,(500,300))
# 转为2进制
img_blob = cv2.dnn.blobFromImage(img_resized,1.0,(500,300),(104.0,177.0,123.0))
# 输入
face_detector.setInput(img_blob)
# 推理
detections = face_detector.forward()
此时
detections.shape # (1, 1, 200, 7)
说明有200个结果,后面的7则是我们做需要的一些数据,继续如下:
# 查看人脸数量
num_of_detections = detections.shape[2]
img_copy = img.copy()
for index in range(num_of_detections):
# 置信度
detections_confidence = detections[0,0,index,2]
# 通过置信度筛选
if detections_confidence > 0.15:
# 位置 乘以宽高恢复大小
locations = detections[0,0,index,3:7] * np.array([img_width,img_height,img_width,img_height])
# 打印
print(detections_confidence)
lx,ly,rx,ry = locations.astype('int')
# 绘制
cv2.rectangle(img_copy,(lx,ly),(rx,ry),(0,255,0),2)
plt.imshow(cv2.cvtColor(img_copy,cv2.COLOR_BGR2RGB))
五、MTCNN
# 导入MTCNN
from mtcnn.mtcnn import MTCNN
# 记载模型
face_detetor = MTCNN()
# 检测人脸
detections = face_detetor.detect_faces(img_cvt)
for face in detections:
x,y,w,h = face['box']
cv2.rectangle(img_cvt,(x,y),(x+w,y+h),(0,255,0),2)
plt.imshow(img_cvt)
对比
优势 | 劣势 | |
Haar | 速度最快、清凉、适合算力较小的设备 | 准确度低、偶尔误报、无旋转不变性 |
HOG+Dlib | 比Haar准确率高 | 速度比Haar低,计算量大、无旋转不变性、Dlib兼容性问题 |
SSD | 比Haar和hog准确率高、深度学习、大小一般 | 低光照片准确率低,受肤色影响。 |
CNN | 最准确、误报率低、轻量 | 相对于其他方法慢、计算量大、Dlib兼容性问题 |
更多推荐
所有评论(0)